AI stands to change cardiology practice, but guidance, evidence of benefit are needed
Click Here to Manage Email Alerts
Key takeaways:
- Guidance is emerging on the use of AI to improve CVD outcomes.
- AI has demonstrated potential in areas such as imaging and ECG interpretation, but outcomes data and interpretability are lacking.
Editor’s Note: This is part one of a three-part Healio Exclusive series on the development and use of AI to improve clinical outcomes in cardiovascular medicine and considerations for regulatory labeling and patient privacy. Part two can be read here. Part three can be read here.
Interest in the use and development of AI to not only improve outcomes but enhance day-to-day productivity is on the rise, and rigorously validated tools and clinical guidance are needed to best implement its use.

In February, American Heart Association councils issued a scientific statement on the use of AI to improve CV outcomes. Considerations for areas including cardiac imaging, electrocardiography, in-hospital monitoring, implantable and wearable technology, genetics and electronic health record data interpretation are detailed in the scientific statement, which was published in Circulation.
“This statement is the first one of the AHA on AI and CVD and care. The objective of this manuscript is to present the state of the art on the use of AI to enable precision medicine in CV research and clinical care,” Antonis A. Armoundas, PhD, associate professor of medicine at Harvard Medical School, affiliate member of Broad Institute at Massachusetts Institute of Technology and assistant in biology at Massachusetts General Hospital Cardiovascular Research Center, told Healio. “This task has been propelled by academia, industry and global governmental agencies that are investing immense resources to transform health care delivery with AI, resulting in a rapid growth rate of scientific research papers on health care-related AI research, which is likely to accelerate in the coming years. Yet, despite enormous academic interest and industry financing, AI-based tools, algorithms and systems of care have yet to improve patient outcomes at scale. Therefore, another objective of this manuscript is to identify best practices, gaps and challenges that may improve the applicability of AI tools in each domain.”
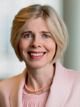
Patricia A. Pellikka, MD, the Betty Knight Scripps Professor of Cardiovascular Disease Clinical Research, president-elect of the Mayo Clinic Officers and Councilors, editor-in-chief of the Journal of the American Society of Echocardiography and consultant in the department of cardiovascular medicine at Mayo Clinic in Rochester, Minnesota, emphasized the potential of AI to significantly impact CV care.
“[The AHA scientific statement] provides an excellent overview of a very dynamic field in medicine,” Pellikka told Healio. “[AI] is certainly needed as an administrative tool to expedite and facilitate documentation and orders. Its greatest impact to society, however, will be in diagnostic and decision support for personalized medicine.”

AI for imaging and ECG interpretation
Machine learning tools are being developed for a variety of uses within CV diagnosis and management, especially within ECG interpretation and cardiac imaging.
“Two areas where AI has made significant strides are in imaging and electrocardiography. There have been studies in which the AI algorithms performed better than the expert clinician,” Armoundas told Healio. “The growing need for ECG interpretation, coupled with the limited skills and availability of human experts, motivates efforts for automated and accurate interpretation of ECGs.”
In the imaging space, AI algorithms are used for referring and scheduling image acquisition, image analysis, reducing image acquisition and processing times, reducing radiation exposure and contrast dose use, assisting diagnosis and reporting and prognostic estimation.
“Expertise in image interpretation takes years to acquire, and experts are often overburdened with their clinical tasks,” Armoundas told Healio. “Moreover, expertise in image interpretation may be scarce, exacerbating inequities in access to high-quality patient care in underserved areas, between lower- and higher-income populations, and between low- and rich-resource countries. AI algorithms for imaging of CVD and stroke address many of these concerns and are, therefore, of increasing interest.”
The AHA statement provided several best practices for the advancement of AI into the imaging space, including specific identification of the clinical problem and needs alongside ethical issues with use of AI tools, as well as not dismissing a patient’s perspective on the utility and effect of AI tools.
Additionally, when developing AI tools for this space, an a priori-defined hypothesis, study objectives and designs are key to assessing an algorithm’s accuracy and validity, according to the statement.
The statement also includes considerations for AI in ECG interpretation, an area where automation can not only scale human interpretation but identify subtle, nonlinear patterns on an ECG and isolate potentially occult disease.
The AHA statement provided the following best practices when validating AI and machine learning algorithms for ECG interpretation:
- The acquisition environment of ECGs used to train AI should match real clinical environments.
- To improve generalizability, clinicians should include as diverse populations as possible.
- Algorithms should be tested in data structures outside of the one they were created in to also confirm generalizability.
Diagnosing low ejection fraction
The results of the randomized controlled EAGLE trial evaluating an AI-powered clinical decision support tool for the early diagnosis of low ejection fraction were published in Nature Medicine in 2021.
In a cohort of more than 22,000 patients at 45 clinics, the researchers demonstrated that AI improved diagnosis of low EF by 31% compared with standard care without increasing the overall rate of echocardiogram usage. The algorithm subsequently received FDA 510(k) clearance for the identification of low LVEF.
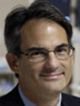
“If a tech company in isolation builds something, it may not be suitable to the workforce; it may not fit into your workflow; and it may not be reliable enough for your needs. For all those reasons, it’s important that clinicians are involved. The health care system is currently very burdened and one of the reasons we enrolled more than 22,000 people so quickly was we didn’t change anybody’s workflow. We didn’t have to teach them how to do something new,” Paul A. Friedman, MD, FHRS, cardiac electrophysiologist and chair of cardiovascular medicine at Mayo Clinic in Rochester, Minnesota, and co-author of the AHA scientific statement, told Healio. “We said, ‘Here’s the test. You already know how to order it. Order it whenever you normally would.’ And when you get the result back, it has that additional information.
“Because it was so easy, people saw it and then they could act on it without having to learn new skills. The more it’s integrated into our workflows, the more we get the implementation right after we confirm the test works and the more it can actually impact human health,” Friedman said.
Training AI for a range of uses
Outside of cardiac imaging and ECG interpretation, the AHA Scientific Statement provides best practices for training AI for a range of uses, including in-hospital monitoring, use of DNA sequencing data to predict potential future CV risk, and interpreting physiological data from implantable and wearable devices. Shaan Khurshid, MD, MPH, cardiac electrophysiologist and assistant in medicine at the Telemachus and Irene Demoulas Family Foundation Center for Cardiac Arrhythmias at Massachusetts General Hospital, instructor of medicine at Harvard Medical School and affiliated scientist at Broad Institute of Harvard and MIT, told Healio about his experiences with the latter.
“I do catheter ablation procedures and device implants of pacemakers and defibrillators and there is a long history of AI in those areas. For example, when we put an implantable monitor in a patient, we get notifications if that monitor finds a dangerous arrhythmia. That is AI. It’s been using AI for a decade,” Khurshid said. “But with the recent explosion of AI in medicine, more people are starting to recognize that. Another example is when we do ablation procedures, there’s a lot of computing that utilizes AI, and that’s already happening in the background.”
Evidence for improved outcomes needed
Any of these applications for AI in clinical practice has key limitations, including the overall lack of outcomes data and interpretability of AI’s decision-making pathways, Khurshid said.
“The key limitation of AI is that the outcomes data are still relatively lacking. ... There’s one randomized trial [EAGLE] where these AI tools were actually validated and found to work. We just don’t have that body of data yet for all of these different tools,” Khurshid said. “One thing that gets brought up is the lack of interpretability. Some of these more complicated models, they just take the angiogram, image or the ECG and spit out a prediction, and there’s no straightforward translation for how it got to that prediction.
“There are techniques that provide some interpretability, that highlight areas of the ECG that are important for the prediction and highlight areas of the image that the algorithm is looking at more than others,” he said. “At the end of the day, as a clinician, if you have that outcome data, if you have those large, randomized trials that show that this saves lives, then I think most clinicians would probably be willing to sacrifice a little bit of interpretability about what the algorithm is doing as long as they know it works.”
What this means, Pellikka told Healio, is that more work needs to be done to ensure AI models work in broad populations.
“Existing AI models require further refinement and prospective testing to ensure that they work in all populations,” she said. “Importantly, we need evidence that we are not only capable of identifying patients at risk, but that we can apply our AI tools to improve clinical outcomes.”
Editor's Note: Part two of this Healio Exclusive series will delve into the framework for successful implementation of AI in CV care as well as areas where clinicians should consider caution.
We want to hear from you:
Healio wants to hear from you: How is AI affecting day-to-day life in your clinical practice? Share your thoughts with Healio by emailing the author at sbuzby@healio.com or tagging @CardiologyToday on X (Twitter). We will contact you if we wish to publish any part of your story.
For more information:
Antonis A. Armoundas, PhD, can be reached at armoundas.antonis@mgh.harvard.edu.
Paul A. Friedman, MD, can be reached at friedman.paul@mayo.edu; X (Twitter): @drpaulfriedman.
Shaan Khurshid, MD, MPH, can be reached at skhurshid@mgb.org; X (Twitter): @shaan_khurshid.
Patricia A. Pellikka, MD, can be reached at pellikka.patricia@mayo.edu; X (Twitter): @pattypellikka.
References:
- Armoundas AA, et al. Circulation. 2024;doi:10.1161/CIR.0000000000001201.
- Khera R, et al. J Am Coll Cardiol. 2024;doi:10.1016/j.jacc.2024.05.003.
- Yao X, et al. Nat Med. 2021;doi:10.1038/s41591-021-01335-4.