AI could improve diagnostic scope of routine coronary angiography
Key takeaways:
- AI accurately predicted several measures of cardiac function from routine coronary angiography.
- Such measurements would otherwise require additional testing via echocardiography and cardiac catheterization.
AI accurately predicted several measures of cardiac function from coronary angiography that would otherwise require additional tests such as echocardiography or catheterization, a speaker reported.
Results of the AI-ENCODE study were presented at the Society for Cardiovascular Angiography and Interventions Scientific Sessions.
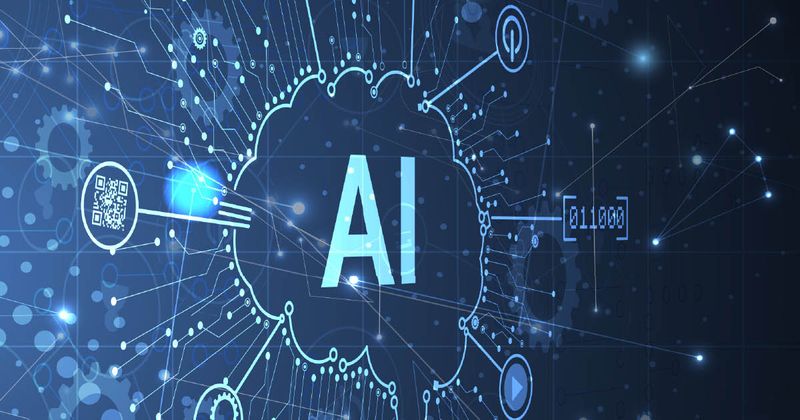
“As the name of the study entails, its objective is to explore whether we can leverage artificial intelligence to discern clinically meaningful data from a coronary angiogram,” Mohamad Alkhouli, MD, FSCAI, division chair of research and innovation at Mayo Clinic School of Medicine in Rochester, Minnesota, said during a press conference. “The hypothesis is that there is a wealth of data on a coronary angiogram and it’s easy to miss what we don’t selectively look for.”

Alkhouli and colleagues used more than 20,000 angiograms performed at Mayo Clinic to train and validate multiple AI algorithms. The selected patients’ angiograms were also accompanied by data from subsequent echocardiography and right heart catheterization to assess predictive accuracy of the AI. The researchers trained the AI algorithms to detect four measures of cardiac function: left ventricular ejection fraction, filling pressure, cardiac output and right ventricular function. The Ensamble deep-learning model was trained to predict reduced LVEF and the 3D-CNN model was trained to predict filling pressure, RV function and cardiac index.
Compared with echocardiography, the Ensamble model accurately predicted reduced LVEF at varying levels, with an area under the curve of 0.84 for EF of 35% or less, 0.87 for EF of 40% or less and 0.81 for EF of 45% or less.
The 3D-CNN model accurately predicted filling pressures, with an AUC of 0.872 and RV function with an AUC of 0.8, compared with echocardiography. Moreover, the 3D-CNN model also predicted abnormal or normal cardiac index of 1.6, 1.8, 2, 2.2 and 2.5, all with an AUC of 0.77 or more, compared with cardiac catheterization.
“The AI-ENCODE project proved that AI can be leveraged to unlock and deliver a broader, more meaningful spectrum of clinical findings from existing angiograms,’’ Alkhouli said in a press release. “This study truly shows us AI’s prowess in revealing insights beyond what the human eye can see. It is important that we leverage AI’s capabilities as a diagnostic tool to provide the best possible [care] for our patients.”
Reference:
- Artificial intelligence yields promising results for advancing coronary angiography. https://scai.org/artificial-intelligence-yields-promising-results-advancing-coronary-angiography. Published May 2, 2024. Accessed May 6, 2024.