AI performs ‘exceptionally well’ predicting post-PCI outcomes
Key takeaways:
- AI could improve prognostic assessment of specific adverse events after percutaneous coronary intervention.
- Many patients reported interest in considering the model’s findings before undergoing PCI.
Researchers at University of Michigan Health developed an artificial intelligence model to accurately assess risk for post-procedural outcomes after PCI.
Patients reported that discussion of the model’s findings before PCI would be important, especially with regard to a specific event, and not generalized composite endpoints, according to the study published in the European Heart Journal.
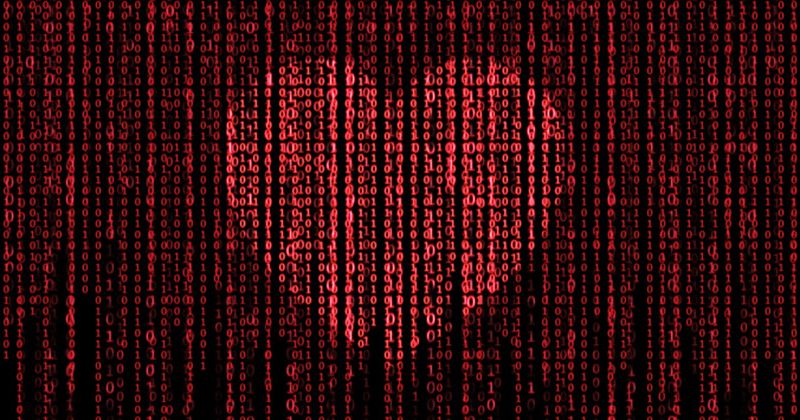
Image: Adobe Stock
“This model was developed using XGBoost, a machine learning algorithm that is especially powerful at identifying complex interactions and nonlinear relationships,” Hitinder S. Gurm, MBBS, interventional cardiologist, professor of internal medicine and interim chief medical officer at University of Michigan Health, told Healio. “The model was developed using a random sample of data from patients undergoing angioplasty and stenting across multiple hospitals in Michigan and then was validated in an independent validation cohort of Michigan patients. We then performed a second validation of the model among a totally new sample of patients from Washington state and the model performed exceptionally well in this population also.”
Model training and validation
The model was trained and developed using a cohort of 107,793 patients in the Blue Cross Blue Shield of Michigan Cardiovascular Consortium (BMC2) registry who underwent PCI from April 2018 to December 2021 (mean age, 67 years; 68% men). Nearly half of the patients had undergone a prior PCI and 15.8% underwent a prior CABG.
“Urgent” PCI was performed among 42.2% of the cohort and the most common indication for PCI was non-STEMI (22.2%).
In-hospital mortality occurred in 1.85% of the cohort, whereas 2.51% had acute kidney injury, 0.44% had new-onset dialysis, 0.41% had stroke, 0.89% had major bleeding and 2.41% underwent transfusion, according to the study.
Gurm and colleagues reported that the machine learning model demonstrated robust prognostic value for outcomes after PCI:
- area under the receiver operating characteristic curve (AUROC) for mortality of 0.93 (95% CI, 0.92-0.94);
- AUROC for acute kidney injury of 0.893 (95% CI, 0.883-0.903);
- AUROC for dialysis of 0.951 (95% CI 0.939-0.964);
- AUROC for stroke of 0.751 (95% CI, 0.714-0.787);
- AUROC for transfusion of 0.917 (95% CI, 0.907-0.925); and
- AUROC for major bleeding 0.887 (95% CI, 0.87-0.905).
External validation and patient survey

“Previous attempts at using such machine learning approaches have suggested the potential for more accurate predictions and our work confirms that possibility,” Gurm told Healio. “What was impressive about the model was that it performed exceptionally well in a totally different population in an independent evaluation performed by the team from Washington state. That speaks to the power and the generalizability of these models.”
External validation using the Cardiac Care Outcomes Assessment Program registry of 56,583 PCI procedures at 33 hospitals in Washington, demonstrated similar discrimination to what was observed in the BMC2 registry, according to the study.
In addition, Gurm and colleagues conducted a semiquantitative survey of 66 individuals (67% women; 85% white) to assess preferences in regard to discussion of the risk prediction results before PCI.
The researchers found that most respondents said each outcome was important; should be discussed before PCI; and requested that risk display include all risks individually, not a composite of endpoints such as “major adverse events vs. minor adverse events.”
“Patient panel feedback came out very strongly in favor of showing data on all key outcomes individually rather than lumping together what are traditionally considered major vs. minor complications,” Gurm told Healio. “The feedback we received was that different patients may value different outcomes differently and the risk calculation and presentation should be individualized.
“We believe additional optimization of these models will require incorporation of a different level of information such as direct consideration of patient angiograms,” he said. “The rapid growth of AI models and cloud computing makes such approaches feasible and I won’t be surprised if such models are developed within a few years.”