‘AI holds great promise’ in early diagnosis of rheumatic heart disease
Click Here to Manage Email Alerts
Key takeaways:
- AI may have a role in future early detection of rheumatic heart disease.
- Machine learning and deep learning models accurately identified mitral regurgitation on pediatric echocardiograms.
Artificial intelligence may have a role in the early detection of rheumatic heart disease using mitral regurgitation jet analysis, according to study findings published in the Journal of the American Heart Association.
“Rheumatic heart disease affects about 40 million people globally, claims nearly 300,000 lives each year. ... Globally, most patients with rheumatic heart disease are diagnosed late, only once symptoms of advanced cardiac disease or complications develop,” Kelsey Brown, MD, pediatric cardiologist at Children’s National Hospital in Washington, D.C., and colleagues wrote. “However, opportunities exist for earlier detection. ... AI is promising in its early stages of identifying mitral regurgitation, present in 80% to 90% of children with early rheumatic heart disease. ... [T]he deep learning method goes beyond specific mitral regurgitation measurements to diagnose rheumatic heart disease using holistic image interpretation. Both machine learning methods for mitral regurgitation jet characterization and deep learning methods were evaluated in parallel to establish if one method is superior in rheumatic heart disease detection in the same data cohort.”
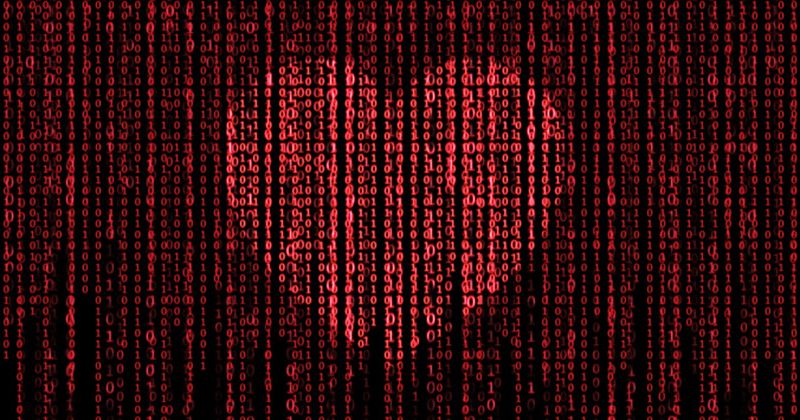
Image: Adobe Stock
Using 511 pediatric echocardiograms and color Doppler images of the mitral valve, following echocardiogram harmonization to localize the left atrium during systole using convolutional neural networks, rheumatic heart disease was detected with machine learning and deep learning models using mitral regurgitation jet analysis.
The images also underwent independent expert panel adjudication.
Overall, 229 echocardiograms were normal and 282 had rheumatic heart disease.
The correct view was identified with an average accuracy of 0.99; the correct systolic frame with apical view was identified with an average accuracy of 0.94; and the correct systolic frame with parasternal long axis view was identified with an accuracy of 0.93, according to the study.
The left atrium apical view was localized with an average Dice coefficient of 0.88 and the left atrium parasternal long axis view was identified with a Dice coefficient of 0.9.
Maximum mitral regurgitation jet measurements were similar compared with the expert panel review (P = .83).
Machine learning for detection of rheumatic heart disease based on mitral regurgitation jet analysis indicated:
- area under the receiver operating characteristic curve (AUROC) of 0.93;
- precision of 0.83;
- recall of 0.92; and
- F1 score of 0.87.
When the researchers tested a deep learning model that combined multiview 3D convolutional neural networks and multiview transformer, analyses indicated:
- AUROC of 0.84;
- precision of 0.78;
- recall of 0.98; and
- F1 score of 0.87.
“AI holds great promise for widespread echocardiography diagnosis of rheumatic heart disease by nonphysician health care workers, but there has been limited progress in this field to date. Our results show that machine learning algorithms for mitral regurgitation jet length and analysis and deep learning algorithms for rheumatic heart disease detection have a high degree of accuracy,” the researchers wrote. “Our machine learning algorithm is the only clinical work to date that looks at automated measurement of mitral regurgitation length and novel mitral regurgitation jet analysis, combining machine learning and deep learning methods, to diagnose rheumatic heart disease.”