AI shows potential for early detection of atrial septal defect
Key takeaways:
- Artificial intelligence effectively detected atrial septal defect on ECGs from three separate institutions.
- Machine learning may improve early identification of the congenital anomaly.
An artificial intelligence-based network successfully identified atrial septal defects from ECGs and holds potential to improve early screening for the congenital anomaly, researchers reported.
Shinichi Goto, MD, PhD, instructor in the division of cardiovascular medicine at Brigham and Women’s Hospital, told Healio that the population-level prevalence of atrial septal defect — the most common form of adult congenital disease — is reportedly approximately 0.2%, and in most cases is asymptomatic until irreversible complications occur.
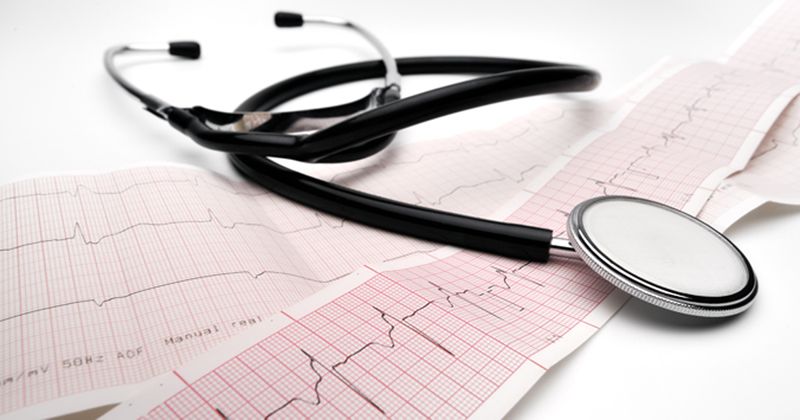
Image: Shutterstock
“Atrial septal defect can be treated in a minimally invasive manner by percutaneous closure to prevent irreversible complications. Thus, early detection and treatment before the onset of irreversible complications is essential,” Goto told Healio.
For this study, the researchers gathered ECG data from Brigham and Women’s Hospital, Keio University Hospital and Dokkyo Saitama Medical Center and labeled them for the presence of atrial septal defect. Patients who underwent atrial septal closure were excluded from the analysis. The analysis included 671,201 ECGs from 80,947 patients.
A convolutional neural network was trained to identify atrial septal defect in a derivation cohort of ECGs from Brigham and Women’s Hospital and Keio University Hospital and tested in a validation cohort from Dokkyo Saitama Medical Center.
The AI model had an area under receiver-operating characteristic curve (AUROC) for detecting atrial septal defect of 0.9 for the test dataset of ECGs from Keio University Hospital and 0.88 for the test dataset of ECGs from Brigham and Women’s Hospital. The AUROC was 0.85 in the external validation dataset from Dokkyo Saitama Medical Center (P for difference between test and validation datasets = .52).
The findings were published in eClinicalMedicine.
“Since the goal is to detect atrial septal defect in an asymptomatic population without other indications for cardiac testing, the model would have to be evaluated in such a population,” Goto told Healio. “Of the three hospitals involved in the study, Dokkyo Saitama Medical Center is a community hospital and thus reflects a more general population than the other two large teaching hospitals. The fact that the model performed similarly in this population supports the generalizability of the model to a wide variety of people. However, since this is still a hospital-based cohort, the model would ideally have to be tested in a prospective study enrolling asymptomatic individuals.”
Moreover, screening simulation using the AI model increased sensitivity from 80.6% to 93.7% at 33.6% specificity compared with using overt ECG abnormalities, according to the study.
“AI is a powerful tool to extract information from complex medical data. Various AI studies have shown that complex medical data (such as images) contains much more information than what is currently used by experts,” Goto told Healio. “AI has the ability to improve health care by maximally utilizing the information content in medical data without the need to develop costly novel tests.”
For more information:
Shinichi Goto, MD, PhD, can be reached at sgoto1@bwh.harvard.edu.