AI shows ‘technical and clinical feasibility’ to detect HFpEF from a TTE video clip
Key takeaways:
- An AI model has been designed to diagnose HF with preserved ejection fraction from a single transthoracic echocardiogram video clip.
- The algorithm demonstrated high levels of sensitivity and specificity.
Artificial intelligence demonstrated strong diagnostic performance in the detection of HF with preserved ejection fraction using a single transthoracic echocardiogram video clip, according to data published in JACC: Advances.
“We hypothesized that from a limited amount of echocardiographic information, we would be able to develop an AI model to detect HFpEF. This diagnosis is difficult to make clinically and even a complete comprehensive echocardiogram may not yield diagnostic results,” Patricia A. Pellikka, MD, the Betty Knight Scripps Professor of Cardiovascular Disease Clinical Research, chair of the division of cardiovascular ultrasound at Mayo Clinic and editor-in-chief of the Journal of the American Society of Echocardiography, told Healio. “HF is an important diagnosis to make; it is estimated to affect more than 64 million people worldwide and is increasing in prevalence. HF is a major cause of hospitalization and death, and treatments are available; it is important to make the diagnosis.”
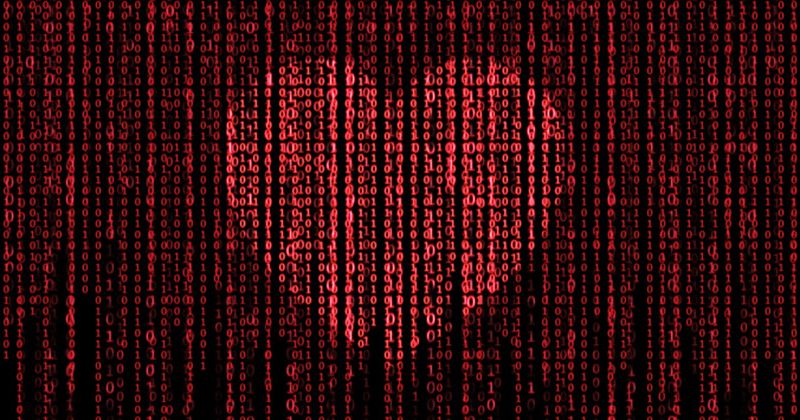
Image: Adobe Stock
Akerman and colleagues trained and validated a 3D convolutional neural network AI using apical four-chamber transthoracic echocardiogram (TTE) video clips from 2,971 patients with HFpEF and 3,785 controls.

“We selected the apical four-chamber video clip, which is a standard part of every echocardiographic examination,” Pellikka told Healio. “This particular view, which shows all four cardiac chambers, contains a lot of information about chamber sizes, wall thicknesses, motion of the mitral and tricuspid annulus, and cardiac function. We used our comprehensive echocardiographic database and linkage to the electronic medical record, which allowed us to correctly identify patients with and without HFpEF in order to train the model. All data were deidentified.”
AI training, validation and performance
The AI model had an area under receiver-operating characteristic curve (AUROC) of 0.97 (95% CI, 0.96-0.97) in training and an AUROC of 0.95 (95% CI, 0.93-0.96) in validation.
In independent testing conducted using 646 cases of HFpEF and 638 controls, the AI demonstrated a sensitivity to detect HFpEF of 87.8% (95% CI, 84.5-90.9) and a specificity of 81.9% (95% CI, 78.2-85.6) with high repeatability and reproducibility, according to the researchers.
Furthermore, performance was assessed in an independent dataset in which the diagnostic value of the AI was compared with the previously validated Heart Failure Association-Pretest Assessment, Echocardiographic and Natriuretic Peptide Score, Functional Testing, and Final Etiology (HFA-PEFF) and Heavy, Hypertensive, Atrial Fibrillation, Pulmonary Hypertension, Elder and Filling Pressure (H2FPEF) scores.
The AI correctly detected HFpEF among 73.5% of 701 indeterminate outputs from the HFA-PEFF score and 73.6% of 776 indeterminate outputs from the H2FPEF score, according to the study.
During a median follow-up of 2.3 years, the researchers observed increased mortality risk among patients with HFpEF as determined by the AI compared with patients in whom the AI did not report HFpEF (HR = 1.9; 95% CI, 1.5-2.4).
“There is currently a shortage of cardiac sonographers in the United States and in some places it can take weeks to get an echocardiogram which is a first line test in suspected HF,” Pellikka told Healio. “Our AI classifier, which requires only one single echo video clip, could facilitate initial triage of patients. Treatment could be initiated on those who have clear evidence of HFpEF.
“There is still much to be learned,” she said. “We need to find out if our model can be used to stratify disease severity, recognize different phenotypes and patterns of HF, and if it can be used to monitor response to therapy. We are also working on an additional AI echo model to detect amyloid heart disease.”
AI as a ‘second read’ when other metrics uncertain
In a related editorial, Andreas B. Gevaert, MD, PhD, postdoctoral researcher in the department of genetics, pharmacology and physiopathology of heart, blood vessels and skeleton at the University of Antwerp, and colleagues discussed the AI model’s performance compared with the two validated scoring systems and the algorithm’s place in clinical decision-making.
“The better performance of the model compared to the scoring systems needs to be interpreted with caution,” the authors wrote. “Natriuretic peptide levels are an essential part of the HFA-PEFF score. However, these were only available in 12% of the validation population, implying that a diagnosis of HFpEF could not be established anyway in the majority of patients using this score. Both scoring systems mandate further (exercise) testing in patients labeled as ‘intermediate’ risk, which was not accounted for in the current study.
“As the authors imply in their paper, the ideal use for this algorithm (or similar AI applications) would be as a ‘second read’ when other clinical or echocardiographic metrics remain uncertain regarding a diagnosis of HFpEF,” they wrote. “AI could serve as a ‘decision maker’ in intermediate or indeterminate cases and/or a ‘gatekeeper’ for advanced exercise testing to enhance early HFpEF diagnosis and enable timely treatment.”
For more information:
Patricia A. Pellikka, MD, can be reached at pellikka.patricia@mayo.edu.