AI identifies reasons for statin nonuse in patients with diabetes at a single center
Key takeaways:
- An AI model identified reasons for statin nonuse among patients with diabetes.
- The most common reasons included statin hesitancy, guideline-discordant practice and clinical inertia.
A deep learning model utilized unstructured electronic health record data to identify specific patient-, physician- and system-level reasons for statin nonuse among patients with diabetes, researchers reported.
Reasons for statin nonuse varied across age, race/ethnicity, insurance and diabetes type, according to the study published in the Journal of the American Heart Association.
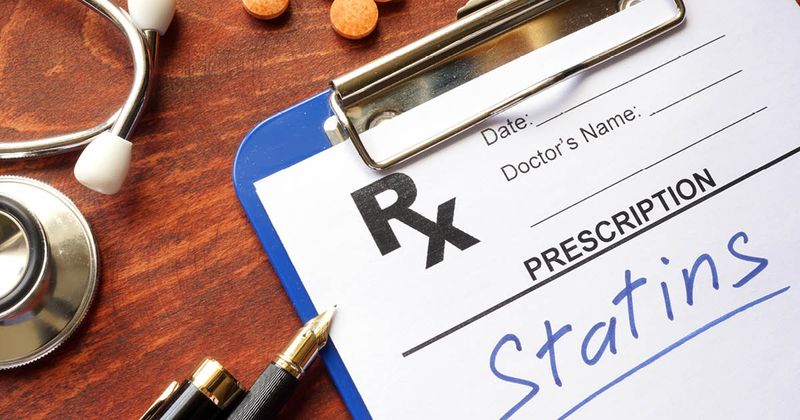
Image: Adobe Stock
“Our data showed gaps and disparities in real-world statin use in individuals with diabetes, and an AI approach can be helpful in assessing complex, unstructured clinical notes to shed light on understanding the reasons behind statin nonuse in a health system,” Ashish Sarraju, MD, former fellow in cardiovascular medicine at Stanford University and current staff cardiologist at Cleveland Clinic, told Healio. “The statin nonuse seen in this study — and a prior study we did with patient with established atherosclerotic CVD — was consistent with what has been described in the literature, so we were not surprised. We knew from prior work that our AI approach performed well with assessing clinical notes, but we were still encouraged by how well it could identify complex reasons for statin nonuse.”

To test their hypothesis, Sarraju and colleagues developed a deep learning NLP algorithm using Clinical Bidirectional Encoder Representations from Transformers (BERT) to identify statin nonuse and actionable reasons for nonuse among 33,461 patients with diabetes at Stanford Health Care Alliance (mean age, 59 years; 49% women; 36% white).
Accuracy of Clinical BERT
The algorithm’s performance was evaluated against expert clinician review and compared with other NLP approaches.
Sarraju and colleagues observed Clinical BERT successfully identify statin nonuse with an area under receiver operating characteristic curve of 0.99 and patient, clinician and system reasons for statin nonuse with an AUC of 0.9.
The researchers reported that Clinical BERT demonstrated good concordance with expert clinician review and outperformed other NLP approaches.
Overall, 47% of the cohort had no statin prescriptions and 16% were using a statin despite no documented statin prescriptions.
Researchers reported that statin hesitancy (19%), guideline-discordant practice (19%) and clinical inertia (18%) were more common compared with side effects and/or contraindications (12%) as the reason for statin nonuse.
Specific reasons for statin nonuse
Reasons for nonuse varied by age, race/ethnicity, insurance and diabetes type, according to the study.
Patients older than 75 years were more likely to experience statin-associated side effects and/or contraindications than statin hesitancy, clinical inertia or discordant practice compared with younger patients (P < .05).
Hispanic individuals were most likely to experience guideline-discordant practice compared with most other reasons for statin nonuse (P < .05), whereas Black patients were most likely to experience clinical inertia as their reason (P < .05), according to the study.
Patients with Medicaid insurance were more likely to experience guideline-discordant practice compared with the other reasons for nonuse (P < .05).
Moreover, patients with type 1 diabetes were more likely to experience guideline-discordant practice compared with the other reasons for statin nonuse (P < .05), according to the study.

“Like many health systems, Stanford is developing dashboards and decision support tools to ensure that patients are receiving guideline-directed statin therapy,” Fatima Rodriguez, MD, MPH, FACC, FAHA, associate professor of cardiovascular medicine and section chief of preventive cardiology at Stanford University School of Medicine, told Healio. “Still, gaps in use of evidence-based therapies are pervasive. Our study highlights that augmenting structured data in clinical notes can help understand some of the reasons behind these gaps and help develop health-system-level interventions to address them.”
For more information:
Fatima Rodriguez, MD, MPH, FACC, FAHA, can be reached at frodrigu@stanford.edu.
Ashish Sarraju, MD, can be reached at sarraja@ccf.org.