Risk scores, algorithms less accurate in stroke prediction in Black vs. white adults
Risk scores and machine learning algorithms were less accurate at predicting stroke for Black adults than for white adults, researchers reported in JAMA.
“Our findings suggest that we may not be adequately capturing all risk factors of stroke,” Michael Pencina, PhD, professor in the biostatistics and bioinformatics and director of AI Health at Duke University School of Medicine, told Healio. “For example, the algorithms tested here generally do not account for social determinants of health and some other factors suggested in Stroke Prevention Guidelines. We need to improve data collection procedures and expand the pool of risk factors for stroke to close the performance gap between algorithms.”
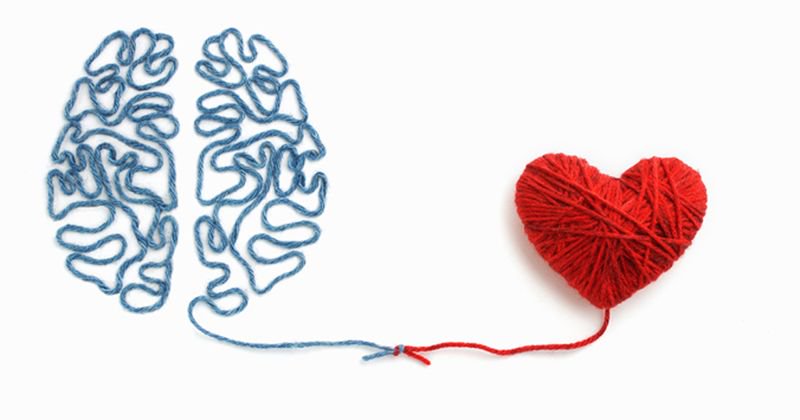
Source: Adobe Stock
Estimating stroke risk
Pencina and colleagues conducted a retrospective cohort study of 62,482 participants (median age, 61 years; 54% women; 29% Black) from four U.S. cohort studies — Framingham Offspring, ARIC, MESA and REGARDS — who were aged at least 45 years and free from stroke or transient ischemic attack at baseline.

“Stroke is the fifth-leading cause of death in the U.S. and incidence of stroke is much higher in Black vs. white adults. High quality predictive algorithms can play an important role in effective preventive strategies,” Pencina told Healio. “We wanted to assess the performance of common risk algorithms for prediction of stroke, across race, sex and age groups. We also wanted to know if advanced machine learning techniques improve performance of the algorithms.”
The researchers estimated 10-year risk for ischemic or hemorrhagic stroke using two risk scores (Framingham and REGARDS) based on self-reported risk factors, pooled cohort equations for atherosclerotic CVD, and two novel machine learning algorithms.
In the overall cohort, discrimination C indexes did not differ between the Framingham and REGARDS models and the pooled cohort equations (Framingham, 0.72; 95% CI, 0.72-0.73; REGARDS, 0.73; 95% CI, 0.72-0.74; pooled cohort equations, 0.72; 95% CI, 0.71-0.73; P for all > .05), according to the researchers.
However, the C index for all three models in white women was 0.76 compared with 0.69 for Black women (P for all < .001), and it was between 0.71 and 0.72 in white men compared with between 0.64 and 0.66 in Black men (P for all < .001), Pencina and colleagues found.
In both races, model discrimination was better for participants younger than 60 years than for those aged 60 years or older.
The ratio of observed-to-expected stroke rates at 10 years was close to 1 in the REGARDS model (1.05; 95% CI, 1-1.09) but was well under 1, indicating overestimation, in the Framingham model (0.86; 95% CI, 0.82-0.89) and the pooled cohort equations (0.74; 95% CI, 0.71-0.77), according to the researchers.
Neither machine learning model produced a C index of above 0.7, except in white women, nor did they outperform the risk prediction models in other aspects, the researchers wrote.
‘Complex math does not help’
“While advanced AI techniques have been touted as the most promising path for better algorithms, our results indicate that for simpler types of data like the ones used in our study, complex math does not help,” Pencina told Healio. “The better accuracy of simpler algorithms based on patients’ self-reporting could be a promising and potentially cost-effective avenue for preventative stroke care. It suggests a need to go out into patient communities, listen, learn, and allow that knowledge to shape our health care tools of tomorrow.”
Reference:
- Algorithms to assess stroke risk are markedly worse for Black Americans. https://corporate.dukehealth.org/news/algorithms-assess-stroke-risk-are-markedly-worse-black-americans. Published Jan. 24, 2023. Accessed Jan. 24, 2023.
For more information:
Michael Pencina, PhD, can be reached at michael.pencina@duke.edu; Twitter: @pencinaphd.