AI decision-support tool accurately identifies aortic stenosis with poor survival odds
In a large, real-world cohort undergoing routine echocardiography, an artificial intelligence decision-support algorithm identified patients with moderate to severe forms of aortic stenosis associated with poor survival if left untreated.
Surgical and transcatheter aortic valve replacement are associated with mortality reduction across all aortic stenosis subgroups, yet data show fewer than half of patients with aortic stenosis with an indication or potential indication for valve replacement are treated, Geoffrey A. Strange, PhD, FCSANZ, senior academic lead at the Heart Research Institute in New South Wales, Australia, said during a press conference at the European Society of Cardiology Congress. An AI decision-support system could better identify patients at risk for death, he said.
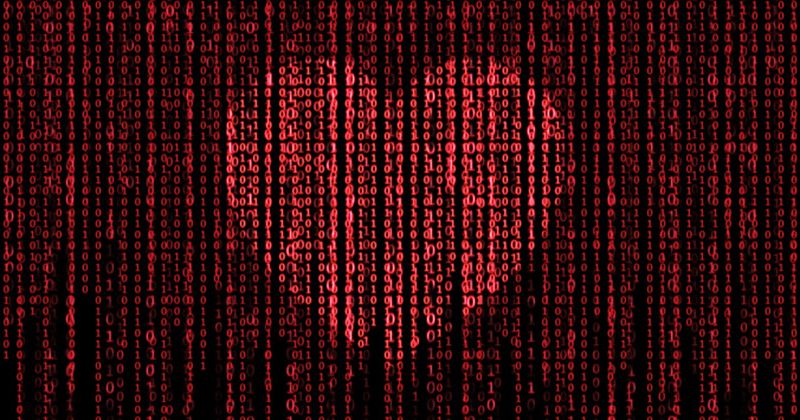
“The decision support algorithm correctly identified patients with significant mortality risk, so in those with moderate disease — 1.4% of the population — when validated against a large database, we were able to show 56% 5-year mortality,” Strange said. “In the severe group, we were able to show 67% 5-year mortality. Within that severe group, there were two cohorts: one that met the current guidelines for severe aortic stenosis, and another that fell just outside the guidelines, but the AI detected a similar risk profile within that population.”
Training a new model
For the ENHANCED-AI study, Strange and colleagues analyzed data from 1,077,145 investigations from 631,824 adults across 23 centers in Australia from 1985 to 2019, with an average 7.2 years of follow-up (mean age, 61 years). The researchers included 70% of the population in an AI training set and 30% of the cohort in a validation group to assess the algorithm’s performance. All data were linked with the National Death Index of Australia.
“We had a six-step process for training the algorithm,” Strange said. “The raw data from the 1 million studies, an imputation model to take care of the discrete nature of echocardiography, the neural network, a Gaussian distribution of the outcome, an error calculation of that outcome and backpropagation.”
The 5-year mortality rates were 22.9% in the low-probability group, 56.2% in those with moderate to severe aortic stenosis and 67.9% in those with severe aortic stenosis.
Compared with the low-probability group, those with moderate to severe aortic stenosis were nearly twice as likely to die within 5 years (OR = 1.82; 95% CI, 1.63-2.02; P < .001). Those with severe aortic stenosis were nearly three times as likely to die within 5 years compared with the low-probability group (OR = 2.8; 95% CI, 2.25-3.06; P < .001).
“We were able to demonstrate that the algorithm’s ability to put different patients into different ‘buckets’ of risk was also associated with long-term survival outcomes,” Strange said.
In analyses that stratified the two severe aortic stenosis groups, those patients that met guidelines for severe aortic stenosis (n = 2,081) were only 26% more likely to die within 5 years compared with patients identified as high risk by the decision-support tool (n = 711; OR = 1.26; 95% CI, 1.04-1.53; P = .021).
Predicting risk in the workflow
In a question-and-answer session after the press conference, Strange said the decision-support algorithm can be deployed directly to an echocardiography lab database.
“What we are planning to do in the future is to ensure there is an alert system within the echo reports themselves,” Strange said. “This algorithm would be deployed either in retrospective time at the end of a week or month, depending on the institution’s workflow, and also in real-time to signal an alert to the reporting physician.”