Apple Watch app, using AI, identifies left ventricular dysfunction
Applying artificial intelligence to an Apple Watch ECG can reliably and safely identify left ventricular dysfunction in a nonclinical setting, researchers reported in a proof-of-concept study.
HF and LV dysfunction affect about 9% of adults aged 60 years and older, and there are effective treatments to lower mortality and hospitalization risk if diagnosed at an early stage, Zachi I. Attia, PhD, MSEE, co-director of AI in cardiology at Mayo Clinic, said during a presentation at Heart Rhythm 2022. Diagnosis of such conditions requires an ECG, MRI or CT, which can be expensive and time-consuming, Attia said, adding an AI model paired with a wearable smart device could prove a useful tool to enable early detection of LV dysfunction.
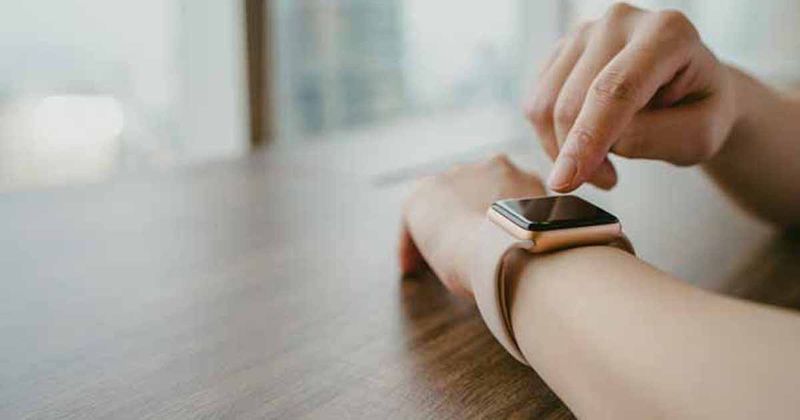
“Two years ago, we created a neural network model using a 12-lead ECG to detect what an echo would say,” Attia said during a press conference. “We did that by showing the neural network about 50,000 ECGs of patients who had an echo, and the network was able to learn subtle patterns predicting the presence of low ejection fraction.”
The AI technology was initially able to detect low EF with an area under the curve of 0.93 and a sensitivity and specificity of 86%, Attia said.
“We asked ourselves, can we use something like the Apple Watch to make this scalable?” Attia said. “We were not sure if we could remotely recruit patients and if they would remain engaged. The second question was, would this work with an Apple Watch ECG?”
Assessing smartwatch data
For the Mayo ECG Watch study, Attia and colleagues analyzed data from 2,454 patients from 46 U.S. states and 11 countries, recruited via email, who had a Mayo Clinic app and owned an Apple Watch. The mean age of participants was 53 years and 56% were women. Patients downloaded a study app that sent all previously recorded ECGs for clinician review; ECGs acquired from the watches within 1 month of a clinically ordered ECG were analyzed by AI for the presence of low EF, using a model adapted for single-lead use.
Participants uploaded 125,610 ECGs in less than 5 months, with 92% of patients using the app more than once (mean, 7.8 uses per user). The researchers assessed area under the receiver operating characteristic curve (AUC), sensitivity and specificity of the AI for EF 40% or lower.
“The patients, even though they had no real incentives, they were not paid, kept uploading their ECGs,” Attia said. “Most uploaded about eight times, or once every other week.”
Within the cohort, 421 patients had at least one normal sinus rhythm ECG (mean, 17 ECGs) within 30 days before or after an Apple Watch ECG. Among the participants, 3.8% had an EF of 40% or lower, with 13 of the 16 patients identified by the watch AI ECG. The AUC was 0.875, sensitivity was 81.2% and specificity 81.3%.
“This is similar to what you would get from a 12-lead, clinically done ECG,” Attia said.
“While [participants] uploaded multiple ECGs, we were still able to take even a single ECG, closest to the echo and still get the AUC of 0.88. We found that this was a useful way to scan patients from diverse populations and still apply these tools when the patient is in their own home.”
Attia said adapting ECG monitoring to an AI-enabled smartwatch for use outside the clinic setting could make such screenings scalable for hospital systems to better serve patients, particularly in remote communities.
“The AI low EF model could be for everyone,” Attia said. “This is a great way to keep monitoring patients who are at higher risk — patients with diabetes, patients with hypertension.”
Questions remain

In discussion after the presentation, Sana M. Al-Khatib, MD, MHS, FHRS, professor of medicine at Duke University School of Medicine and Duke Clinical Research Institute, noted that the sensitivity and specificity of the low EF algorithm were good and the data demonstrated successful development of an infrastructure to recruit patients remotely, transmit data securely and maintain patient engagement. However, only 3.8% of Mayo patients initially surveyed for recruitment were interested in participating; the number of participants with analyzable data was even lower.
“To me, this raises questions about the generalizability of their findings,” Al-Khatib said. “It will be important to see these findings validated and replicated by other groups. While this technology is innovative and promising, it is not ready for application in clinical practice. I am eager to see what the next steps will be.”