Machine learning may identify treatment options for depression
Click Here to Manage Email Alerts
Key takeaways:
- Ensemble machine learning models may predict secondary treatment for depression that does not respond to antidepressants initially.
- Prediction success depends on treatment type.
WASHINGTON — Ensemble machine learning models may indicate second-line treatments for people whose depression does not benefit from antidepressant pharmacotherapy, according to a poster presented here.
The poster was awarded best poster from an early career professional by the Anxiety & Depression Association of America.
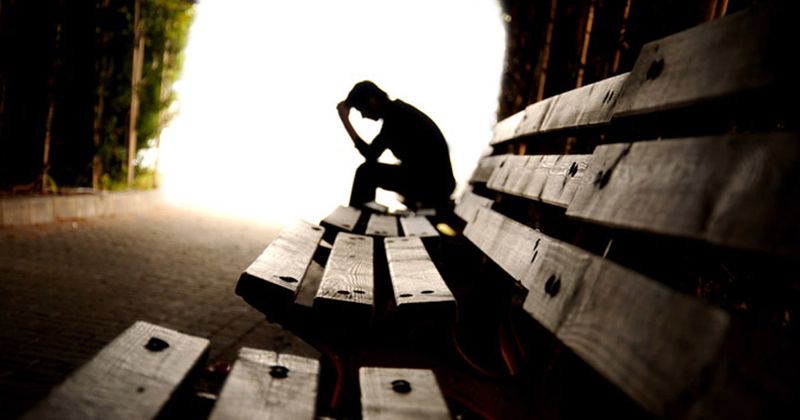
Joshua Curtiss, PhD, a postdoctoral fellow in psychology at the Depression Clinical & Research Program at Massachusetts General Hospital in Boston, and colleagues used data from the Sequenced Treatment Alternatives to Relieve Depression (STAR*D) study to evaluate ensemble machine learning models. Specifically, they used data from 1,439 STAR*D participants who were randomly assigned to seven types of treatment after antidepressant treatment.
To evaluate the ensemble model, which implemented multiple types of machine learning algorithms, the researchers used 155 predictor variables drawn from clinical and demographic variables to identify the likelihood of remission. They also evaluated the model using the top 10 predictors of remission.
“Including every single feature, every single variable in the world, mindlessly isn’t necessarily always the best step. You still want to be thoughtful about how you do this,” Curtiss said during the presentation. “Just using a simple t-test filter including the top 10 predictors is one of the simplest things you could do. You can outperform chance better than if you use absolutely every [predictor].”
Analyses of the machine learning predictions revealed they were least successful for augmented medication options (area under the curve [AUC] = 0.53-0.56) and most successful for cognitive therapy (AUC = 0.82).
“This is a good first step, but overall predictive performance was modest,” Curtiss said. “This is consistent with other machine learning sources and studies looking at these kinds of things.”
Moving forward, the researchers said future studies should identify better strategies for analyses and better predictor sets.