'You have to look' to see if suicide prediction models harm certain racial/ethnic groups
Certain suicide prediction models may disproportionately benefit white, Hispanic and Asian patients vs. Black and American Indian/Alaskan Native patients and those with unrecorded race/ethnicity.
Researchers published these findings in a diagnostic/prognostic study published in JAMA Psychiatry.
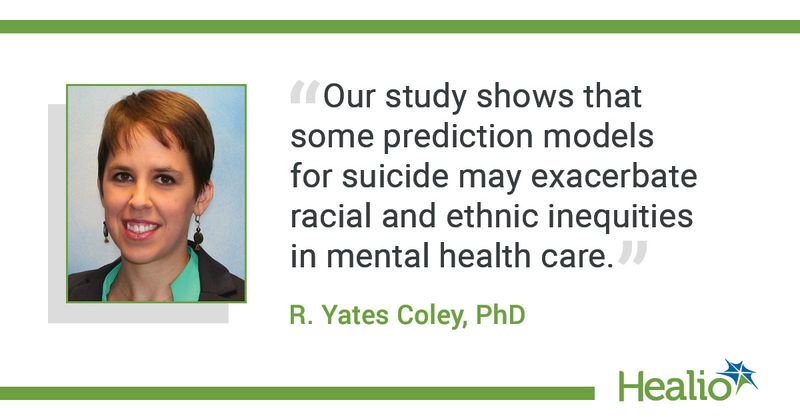
“Prediction models are increasingly being used in clinical care, but they don’t necessarily perform the same across all racial and ethnic groups,” R. Yates Coley, PhD, assistant investigator at Kaiser Permanente Washington Health Research Institute, told Healio Psychiatry. “We are not aware of anyone previously examining suicide prediction models to evaluate whether they may widen disparities in mental health access, treatment and outcomes between different racial and ethnic groups. We must insist that new technologies and methods be used to reduce racial and ethnic inequities in care, not exacerbate them.”
Coley and colleagues sought to assess the performance of suicide prediction models according to racial/ethnic differences. Specifically, they assessed all outpatient mental health visits to seven large integrated heath care systems by individuals aged 13 years or older, with the study conducted between Jan. 1, 2009, and Sept. 30, 2017, and follow-up through Dec. 31, 2017. They used logistic regression with Least Absolute Shrinkage and Selection Operator variable selection and random forest among a training set featuring all visits from a 50 random sample of patients, for 6,984,184 visits, to estimate prediction models. They assessed performance in the remaining 6,996,386 visits, which included 4,031,135 visits from white individuals, 1,664,166 visits from Hispanic individuals, 578,508 visits from Black individuals, 313,011 visits from Asian individuals and 48,025 visits from American Indian/Alaskan Native individuals, as well as 274,702 visits from individuals whose race/ethnicity was not recorded. Data were available for 1,433,543 individuals (64% women; mean age, 42 years). Variables related to demographics, diagnoses, prescriptions and utilization and responses to the Patient Health Questionnaire 9 served as exposures. Suicide death in the 90 days following a visit served as the main outcome.
Coley and colleagues reported 768 suicide deaths within 90 days after 3,143 visits. Visits by patients whose race/ethnicity were not recorded had the highest suicide rates, for a rate of 5.71 per 10,000 visits, followed by visits by Asian (rate = 2.99 per 10,000 visits), white (rate = 2.65 per 10,000 visits), American Indian/Alaskan Native (rate = 2.18 per 10,000 visits), Hispanic (rate = 1.18 per 10,000 visits) and Black (rate = 0.56 per 10,000 visits) patients. Both models exhibited high area under the curve and sensitivity for white, Hispanic and Asian patients; however, both were poor for Black and American Indian/Alaskan Native patients and those without race/ethnicity recorded. As an example, the researchers noted an AUC for the logistic regression model of 0.828 (95% CI, 0.815-0.84) for white patients compared with 0.64 (95% CI, 0.598-0.681) for those with unrecorded race/ethnicity and 0.599 (95% CI, 0.513-0.686) for American Indian/Alaskan Native patients. Further, they noted sensitivity at the 90th percentile of 62.2% (95% CI, 59.2-65) for white patients vs. 27.5% (95% CI, 21-34.7) for those with unrecorded race/ethnicity and 10% (95% CI, 0-23) for Black patients. They observed similar results for random forest models.
“Our study shows that some prediction models for suicide may exacerbate racial and ethnic inequities in mental health care,” Coley said. “Before we implement any prediction model in our clinics, we must test for disparities in accuracy and consider the possible negative consequences, including harms that may be disproportionately experienced by BIPOC populations. Our study provides guidance on how to do such evaluations and lays out the steps that should be taken when considering whether to implement a prediction model to ensure inequities are not perpetuated.”
Coley noted that it’s impossible to know whether a prediction model is useful or harmful until it is evaluated.
“The first message of our study is this: You have to look,” Coley added.