Existing suicide risk prediction models may be cost-effective in health care settings
Click Here to Manage Email Alerts
Existing suicide risk prediction models appeared sufficiently accurate to be cost-effective in United States health care settings, according to results of an economic evaluation published in JAMA Psychiatry.
“Multiple research groups have developed suicide risk prediction models using electronic health record data,” Eric L. Ross, MD, of the department of psychiatry at McLean Hospital in Boston, and colleagues wrote. “A recent systematic review identified such predictive models that have been described in the literature. In reviewing the accuracy of these models, the authors highlighted their low positive predictive value (PPV) (most models had a PPV <1% for predicting suicide mortality) and suggested that this attribute might preclude any practical application of such models. Subsequent commentators have challenged this conclusion, noting that the health economic value of risk prediction depends not only on its accuracy but also on the consequences of the condition it predicts and the efficacy of interventions for that condition; within this cost-benefit framework, what constitutes an adequate accuracy for suicide risk prediction is unknown.”
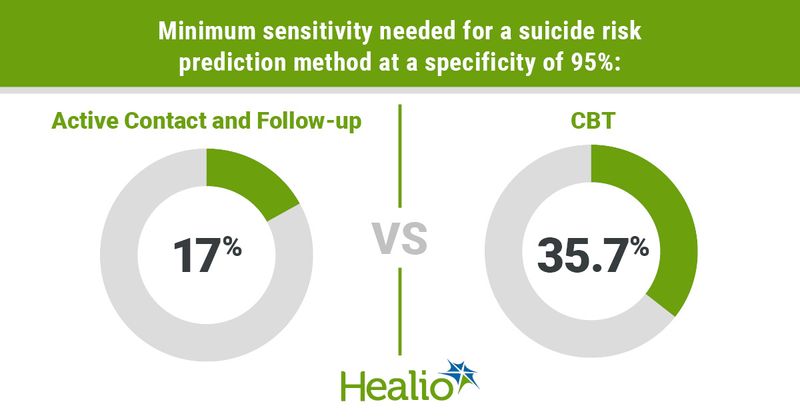
The investigators aimed to address this research gap by pinpointing threshold values of sensitivity, specificity and PPV that would make a suicide risk prediction method cost-effective for targeting a suicide risk reduction intervention to individuals at high risk. Using a novel decision analytic model, they analyzed data related to suicide epidemiology, suicide health care and societal costs and the costs and efficacy of suicide risk reduction interventions. The model estimated lifetime health economic outcomes related to suicide for a population of U.S. adults with a primary care physician. Individual at high predicted risk received either active contact and follow-up, which had a relative risk for suicide attempt of 0.83 and an annual health care cost of $96, or cognitive behavioral therapy (CBT), which had a relative risk for suicide attempt of 0.47 and an annual health care cost of $1,088. Main outcomes and measures included fatal and nonfatal suicide attempts; quality-adjusted life-years (QALYs); health care sector costs and societal costs in 2016 U.S. dollars; and incremental cost-effectiveness ratios, with those of $150,000 per QALY considered cost-effective.
Results showed primary care-based suicide risk prediction with a specificity of 95% and a sensitivity of 25% was able to reduce suicide death rates by 0.5 per 100,000 person-years when targeting active contact and follow-up or by 1.6 per 100,000 person-years when targeting CBT from a baseline of 15.3 per 100,000 person-years. A risk prediction method at a specificity of 95% needed a sensitivity of 17% or greater (95% CI, 7.4-37.3) and 35.7% or greater (95% CI, 23.1-60.3) for cost-effectiveness from a health care sector perspective when targeting active contact and follow-up and CBT, respectively. Active contact and follow-up needed PPVs of 0.8% for predicting suicide attempt and 0.07% for predicting suicide death, and CBT needed PPVs of 1.7% for suicide attempt and 0.2% for suicide death, to achieve cost-effectiveness.
“Although the results do not conclusively demonstrate that any specific suicide risk prediction model is cost-effective, they suggest that current risk prediction models have achieved sufficient accuracy for health systems to move forward with pilot implementation projects,” Ross and colleagues wrote.