Algorithm may predict risk for PTSD among trauma survivors
An algorithm may be able to predict the likelihood of trauma survivors developing PTSD, according to results of a study published in Nature Medicine.
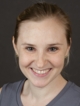
“Although some people who experience trauma are at high risk for developing PTSD, the majority of the 139 million people who visit EDs in the U.S. annually do not report trauma exposure or do not develop PTSD despite exposure to trauma,” Katharina Schultebraucks, PhD, assistant professor of behavioral and cognitive sciences in emergency medicine at Columbia University Vagelos College of Physicians and Surgeons, told Healio Psychiatry. “To predict who is at risk and who is likely not at risk is of high importance as it may provide opportunities for more targeted preventive measures. Multiple independent studies have identified predictive signals of long-term risk after trauma exposure that can be routinely assessed by ED clinicians.”
These signals included aspects of the biological stress response, immune response, threat perception, psychophysiological arousal and psychosocial determinants of clinical risk. Schultebraucks and colleagues sought to determine whether this routinely available data can be used to quantify the likelihood of ED patients developing PTSD within a 12-month period following trauma exposure. Specifically, they aimed to develop a clinical prediction model that would be useful for future research on targeted prevention of PTSD among trauma survivors.
Using supervised machine learning, the investigators developed the algorithm to compute a single PTSD risk score by combining 70 clinical data points, as well as incorporating a brief clinical assessment of a patient’s immediate stress response. They collected data of 377 adult trauma survivors in Atlanta and then tested the algorithm among 221 adult trauma survivors in New York City.
Results showed 90% of patients who the algorithm categorized as having PTSD risk developed long-lasting symptoms within 1 year. Further, the algorithm identified 5% of patients who were free of long-lasting PTSD symptoms as being at risk. Among patients predicted to have few or no PTSD symptoms, 29% developed long-lasting PTSD.
“One possible implementation of our model may be as a rapid and automated clinical read-out available for ED clinician and discharge planning,” Schultebraucks told Healio Psychiatry. “The nature of the candidate predictor variables and the timing of their measurement make this goal amenable for further investigation and would have significant implications for both the early identification of posttraumatic stress following trauma, as well as integration of acute care providers managing such patients in the near term. However, for the current algorithm to be used in routine care, important additional steps are required to promote the interoperability and implementation of the predictive model into the medical infrastructure.
“Moreover, we also found that acute stress symptoms are important predictors of long-term PTSD risk. Therefore, it can be recommended to pay close attention to such symptoms in trauma survivors and to closely monitor these patients in order to offer follow-up care and to improve their stress management if so indicated.”