Researchers create suicide risk prediction model using EHR data
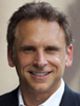
An electronic health record-based suicide risk prediction modeling approach detected risk for suicidal behavior among patients across independent health care systems, according to results of a prognostic study published in JAMA Network Open.
“Most people who attempt or die by suicide are seen by a clinician in the month leading up to the event, but we know from previous studies that clinicians’ ability to predict suicide is only slightly better than chance,” Jordan W. Smoller, MD, ScD, trustees endowed chair in psychiatric neuroscience at Massachusetts General Hospital and professor of psychiatry at Harvard Medical School, told Healio Psychiatry. “The vast resource of longitudinal electronic health records provides a new opportunity to build risk prediction tools that could enhance our ability to identify those at risk. This study demonstrates the feasibility of combining the large, real-world data captured in electronic health records with machine learning to train and validate a risk prediction algorithm for suicide attempts across multiple diverse healthcare systems.”
Smoller and colleagues used readily available structured longitudinal data from EHRs to evaluate the cross-site performance and generalizability of a risk prediction method in predicting incident suicide attempts across multiple independent U.S. health care systems. They extracted data of more than 3.7 million patients from five health care systems that participated in the Accessible Research Commons for Health network. Available data spanned 6 to 17 years, up to 2018, and comprised ICD-9 diagnoses, procedure codes, laboratory test results and medications. The researchers defined outcomes by ICD-9 codes reflecting incident suicide attempts and trained models using naive Bayes classifiers in each of the five systems. They cross-validated models in independent data sets at each site and calculated performance metrics.
Smoller and colleagues identified 39,162 (1.1%) suicide attempts. Although predictive features varied by site, the most common predictors reflected mental health conditions. These included borderline personality disorder, which had odds ratios of 8.1 to 12.9, and bipolar disorder, which had odds ratios of 0.9 to 9.1. Substance use disorders also were common, including drug withdrawal syndrome, which had odds ratios of 7 to 12.9.
Across sites, model performance was similar, with areas under the curve ranging from 0.71 (95% CI, 0.7-0.72) to 0.76 (95% CI, 0.75-0.77), despite variation in population health characteristics, demographic characteristics and geographical location. The models detected a mean 38% of cases a mean 2.1 years in advance across sites, at a specificity of 90%.
“It’s important to note that the goal of this work is to inform and not to replace clinical judgment,” Smoller told Healio Psychiatry. “We also recognize that a number of challenges remain before such a model could be deployed clinically — including the need to optimize the trade-off between false positives and false negatives. However, we are optimistic that this work and efforts by other researchers can move us into a new era in which clinicians can more effectively assess risk and prevent this tragic outcome.” – by Joe Gramigna
Disclosures: Smoller reports being an unpaid member of the Bipolar/Depression Research Community Advisory Panel of 23andMe. Please see the study for all other authors’ relevant financial disclosures.