‘Deep learning’ potential breakthrough in diabetic retinopathy management
Click Here to Manage Email Alerts
IBM Research announced a technological advance that uses “deep learning” to achieve the highest-ever recorded accuracy using pathology-driven analysis for identifying diabetic retinopathy severity, based on an international, clinical diabetic retinopathy scale that determines severity in about 20 seconds.
The technology may create new levels of efficiency, helping practitioners screen a greater number of patients with diabetes, and more quickly refer those who need specialist care, according to IBM.
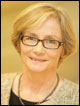
Joanna Batstone, PhD, vice president and lab director, IBM Research–Australia, and chief technology officer, IBM Australia and New Zealand, spoke to Healio.com/Primary Care Optometry News about these new advancements.
Healio: What exactly is deep learning?
Batstone: Deep learning is a class of machine learning algorithms inspired by the structure and function of the brain. It involves many processing layers for extracting features and detecting patterns in an image, where each layer uses the output from the previous layer as input. Each layer learns a different level of representation corresponding to a different level of abstraction. For example, starting from low-level features such as color, to mid-level features such as specific pathology patterns within the image (hemorrhages and micro-aneurysms), to high-level features such as the disease state of the image. Convolutional neural networks (CNN) is one of most popular techniques in deep learning.
Healio: How was this technology developed and how does it work?
Batstone: We have trained a CNN-based algorithm to analyze images pixel by pixel and patch by patch. Based on these visual characteristics, it learns patterns associated with diabetic retinopathy (DR). We are coupling the CNN-based approach with a dictionary learning-based method to more precisely capture fine-grained pathology information to improve the final DR classification results. Our trained deep convolutional neural network automatically learns the hierarchical representation of retinal images such as color, edges, orientation of vessels, intensity variation patterns, etc., associated with different severity levels of DR, using a large number of expert annotated retinal fundus images.
Additionally, we have developed a machine learning technique that uses expert annotated retinal pathologies such as hemorrhage, exudates, etc., and healthy regions in the retinal fundus image to create a visual dictionary of retinal pathologies. This dictionary encodes a query retinal image with respect to the frequency of pathology and healthy areas within the image.
Finally, our technology combines these components to compute the severity level of DR.
Healio: Where do you see this technology fitting into the health care continuum?
Batstone: For the developed world and metropolitan areas, we see the potential for this technology to help streamline disease assessment, which is currently a manual and subjective process in the clinical workflow. Identifying the disease severity grade is dependent on the clinician's level of expertise, so we see that this technology could help give greater confidence in their analysis. The potential for this in a cloud delivery model is also promising, where a clinician or a patient could easily and quickly access results from the cloud on a mobile device via any browser we use today.
In relation to the developing world, including rural areas where there is not always a sufficient number of trained specialists available, we see potential that this could help inject greater efficiency into programs that focus on remote diagnosis (teleophthalmology). For example, in Australia, legislation recently changed that allows general doctors to conduct these dilated eye exams and send these images away to specialists for remote analysis. While this is a big step forward for increasing access to screening, the risk of missed follow-up appointments to get the results remains a challenge. Technology like this may potentially allow general practitioners or nurses to give patients an indication of their risk of the disease on the spot, referring them to specialists for immediate care and treatment.
Healio: In what setting would it be administered?
Batstone: Our vision is that after necessary regulatory approvals this assistive technology could become available to support health care professionals as part of their workflow and at the point of care everywhere, including remote and rural areas where access to resources and specialists is often lacking.
Healio: What will its impact be on optometry?
Batstone: The important breakthrough in our research is the ability for the technology to not only triage abnormal cases, but to estimate the severity level of the disease. This is an important factor for eye specialists in informing patient care and treatment, and it is currently a manual and often subjective process. If this type of technology could help support eye specialists to more easily grade severity levels, treatments could be more precise.
For example, according to the National Eye Institute, early stage DR progression can be slowed through optimal diabetes management and at least once yearly screening; whereas those patients with severe or proliferative DR require screening as often as every 2 to 4 months with more invasive and complex treatment options to prevent blindness. – interviewed by Abigail Sutton
Disclosure: Batstone is the vice president and lab director, IBM Research–Australia, and chief technology officer, IBM Australia and New Zealand. She has published more than 80 papers and organized industry conference symposia.