BLOG: Including imaging data in IRIS Registry expands its depth
Key takeaways:
- The expansion of the IRIS Registry offers more opportunities for curated data sets.
- AI algorithms may help identify disease biomarkers and drug targets.
The American Academy of Ophthalmology recently expanded the depth and breadth of the IRIS Registry by including data from approximately 3.5 million real-world patient images.
This new data set adds to the robustness of the IRIS Registry, which houses data from more than 78 million patients and 490 million billable patient encounters, with 10 years of longitudinal data. Nearly 16,000 clinicians contribute to the IRIS Registry. The addition of these images, which are securely linked to deidentified patient profiles, allows for the creation of curated data sets that investigators may turn to while conducting research on a variety of ophthalmic conditions.
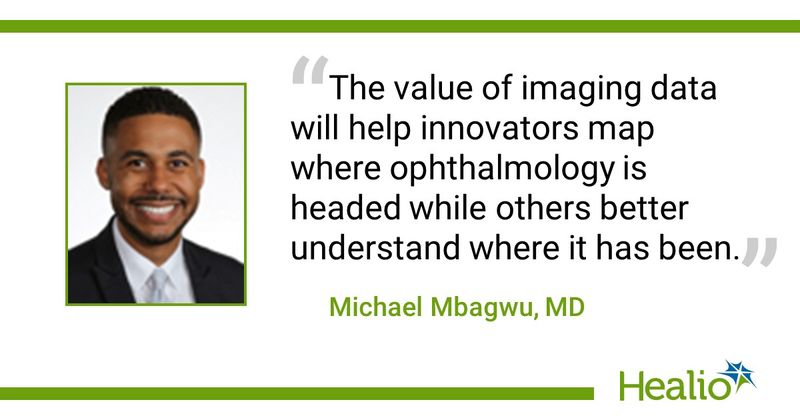
In the earliest versions of the IRIS Registry, clinicians who linked their electronic health record systems to the database contributed deidentified quantitative demographic and clinical data (eg, age, ICD diagnoses, visual acuity measurements) and qualitative data (eg, information derived from unstructured clinical notes in various EHR systems). Life sciences companies gleaned valuable insights from these data: characterization of real-world progression of retinal diseases, tracking of vision-related quality of life data in patients with geographic atrophy (GA), and emulation of the VIEW 1 and VIEW 2 studies in wet age-related macular degeneration using real-world patient data.
The addition of deidentified patient imaging data to the IRIS Registry required a heavy lift. The AAO turned to Verana Health, the exclusive end-to-end data curation and analytics partner of the IRIS Registry, to assist in this initiative; Verana Health’s experience with curating data for the earlier iterations of the IRIS Registry provided the familiarity necessary for this next step.
Artificial intelligence, such as machine learning (with the oversight of clinicians), helped reconcile EHR data and images into fully curated data sets called Qdata modules. Broadly, Qdata modules are research-ready: There is currently a Qdata module for glaucoma and will soon be modules for GA, wet AMD and other ophthalmic areas. Within each Qdata module, investigators will be able to further explore the dynamics of particular patient subgroups by toggling any number of variables within the data set.
An example of how this might work illustrates the value of Qdata modules. Before these data sets were established, investigators might have looked at claims data to answer a question — for example, to determine how race is linked with decline in visual acuity (VA) in patients with GA. These assessments, while useful, were nevertheless blunt instruments. With more refined data sets that enable life sciences companies to filter information within the larger data set, they can find answers to more complex questions — say, to determine the differences in VA outcomes among Black and white GA patients who were diagnosed between 2016 and 2020, had a history of smoking, and were older than the age of 80 years at the time of diagnosis.
Adding images to that data set will help provide an even wider breadth for life sciences companies. Building on the above examples, investigators could look beyond an EHR-derived data point such as VA, instead opting to find the differences in, say, total GA lesion area on fundus autofluorescence imaging among Black, Hispanic and white GA patients who are older than 85 years, have had 5 years’ duration of disease, and have a history of bilateral cataract surgery.
The value of imaging data will help innovators map where ophthalmology is headed while others better understand where it has been. Use of an AI algorithm designed to analyze images may identify novel biomarkers and potential drug targets, empowering life sciences companies to design the next era of therapies based on richer data sets.
References:
• Curated, clinically true data modules to power research. https://www.veranahealth.com/data-and-technology/qdata/. Accessed June 27, 2023.
• Qdata glaucoma powers quality insights only found here. https://info.veranahealth.com/qdata-glaucoma. Accessed June 27, 2023.