AI models must be safe, trustworthy in clinical practice
Key takeaways:
- Artificial intelligence models need to explain decisions and quantify uncertainty to be safe and trustworthy.
- These factors can be implemented without worsening performance.
SAN DIEGO — To gain clinical adoption, artificial intelligence models cannot be a black box, according to a speaker at DOS Digital Day at the American Society of Cataract and Refractive Surgery meeting.
Brian M. Fernandez, MD, said AI is gaining traction in ophthalmology, but designers of AI models need to take safety into account.
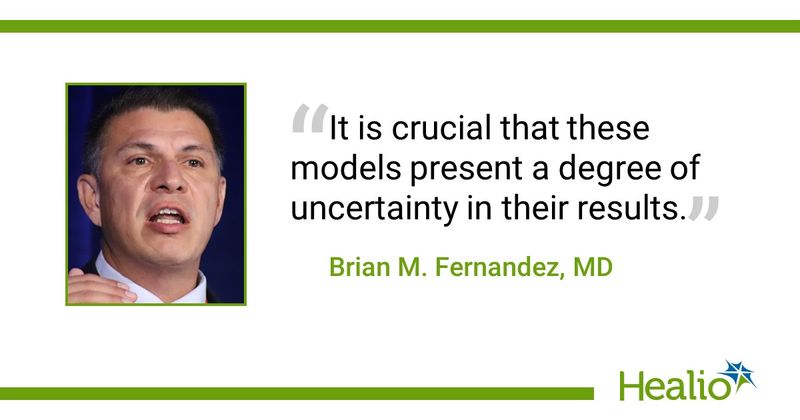
“The most important aspect of these tools is clinical safety,” he said. “Providing a wrong diagnosis or missing a disease has serious consequences for our patients. ... It is crucial that these models present a degree of uncertainty in their results.”
In addition to clinical needs such as safety, Fernandez said AI models need to be explainable and interactive.
AI models also need to adapt to new real-world data outside of the framework in which they were trained.
Fernandez explained the concept of out-of-distribution detection, which will be crucial to adopting AI in the clinic. Out-of-distribution detection is the ability of an AI model to recognize data on which it has not been trained. If the model cannot recognize this data, Fernandez said there is potential that the model will make erroneous predictions.
Finally, models must be able to quantify their uncertainty. Fernandez said including a small number of outliers in the training set can guard the model against misclassifying images while improving outlier exposure and uncertainty measures.
“We believe that a safe and trustworthy AI model can explain why it made its decisions and also be able to quantify the degree of uncertainty,” Fernandez said. “Those aspects can be considered into the model training without worsening the performance of the original task.”