BLOG: Comparing unstructured notes within an ophthalmology-specific database
Some data points housed in electronic health record systems are more suitable for large-scale evaluation than others.
Quantifiable data points (eg, IOP and visual acuity) are, in general, more easily aggregated and compared than qualifiable information such as free text in a patient note from a clinical encounter. Recording unstructured notes in a patient record is useful for tracking a clinical course, symptomatology, and a clinician’s observations, prognoses and therapeutic plan. They are beneficial for patient-level encounters, but they present a challenge for large-scale data analysis. Although the answer is complex, the question is simple: How do you compare notes recorded from physician-specific shorthand across multiple EHR systems? Finding an effective methodology to do so could yield important insights.

Source: Adobe Stock.
Methods that efficiently turn unstructured, language-based information into data points that can be compared across different EHR platforms leverage natural language processing (NLP). We encounter artificial intelligence-powered platforms that use NLP in our day-to-day lives. Think, for example, of how you can enter credit card information into an app-based e-commerce platform. You can either manually enter a cardholder name, card number and expiration date into the relevant fields, or you can use your cell phone’s camera to scan the card and autopopulate the corresponding areas into an app. The latter option uses NLP as a means of quickly scanning unstructured data (ie, the cardholder name, expiration date, etc) from anywhere on the card — and from numerous types of credit cards with different formatting, fonts and ordering of information — and transforming it all into structured data.
An AI algorithm seeking to collect and standardize data from unstructured clinician notes would similarly be able to help interpret text-based language housed in different locations across various EHR interfaces and care settings.
The American Academy of Ophthalmology has partnered with Verana Health, the data curation and analytics partner of the Academy, to manage its IRIS Registry. Verana Health uses its VeraQ population health data engine to manage this data at scale, including deidentifying, interpreting and standardizing text-based data from unstructured notes found in EHR systems used by clinicians who participate in the IRIS Registry. Leveraging NLP for this purpose expands the utility and depth of the IRIS Registry database, which can, in turn, help enrich our field’s understanding of real-world patient dynamics.
To illustrate the value of interpreting unstructured notes in ophthalmology, consider the example of a patient who presents to a general ophthalmologist with complaints of decreased visual acuity. Upon examination, it is noted that the patient has extrafoveal geographic atrophy (GA) lesions and mature cataracts. When documenting this encounter, the clinician may use ICD-10 for cataract diagnosis and relevant surgery CPT codes for reimbursement purposes and note the presence of GA lesions in the visit notes section.
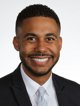
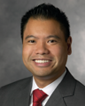
If researchers seeking to understand the real-world prevalence of GA strictly relied on ICD-10 coding data, then this patient would likely be excluded from their data aggregation. If, however, these same researchers’ efforts included search parameters that sought to detect particular phrases and abbreviations linked with GA (eg, “geographic atrophy,” “GA,” “geograph. atroph.”) from clinician notes, then this patient could be included. Verana Health’s NLP algorithms applied in VeraQ are optimized for use in reviewing unstructured language in EHR formats and customized to detect ophthalmology-specific abbreviations such as those outlined above. This has helped to accelerate the pace at which the IRIS Registry is processing unstructured language into quantifiable data points.
Use of NLP in analysis of the IRIS Registry database to create a more robust real-world data set helps researchers sharpen their understanding of disease prevalence and real-world patient dynamics, which may lead to new strategies for effectively addressing challenges revealed by this improved method of collecting data. The specific data yielded from large-scale NLP use is yet to be determined. Still, the potential to arrive at a more refined understanding of our patients’ needs and our field’s nuances should make clear the potential advantages this approach offers.
Collapse