AI algorithm shows promise for early identification of geographic atrophy biomarkers
Click Here to Manage Email Alerts
FORT LAUDERDALE, Fla. — A machine-learning algorithm showed the ability to identify spectral-domain OCT predictors of geographic atrophy in eyes with intermediate age-related macular degeneration.
“We used the merged [intermediate] AMD database from the prospective A2A SD-OCT study, a prospective, observational, ancillary study of a subset of eyes from AREDS2, including 316 participants,” Eleonora M. Lad, MD, PhD, said at the Retina World Congress.
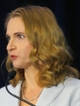
The method automatically identified the specific structural features most associated with or predictive of geographic atrophy (GA), “opening the ‘black box’ that we don’t like as clinicians,” she said.
While some of the features were consistent with prior literature, such as hyperreflective foci, drusen, nascent GA, and incomplete retinal pigment epithelium and outer retinal atrophy, other features were more unexpected and not previously identified by any human experts. These were areas of the neurosensory retina overlying specific types of drusen, areas of choriocapillaris and choroid underlying drusenoid pigment epithelial detachments, and drusen.
“It is not a surprise to us that computers think differently and that the algorithm identified biomarkers that were not previously described by us as human experts. The basis of them needs to be elucidated by future research,” Lad said.
The model had excellent performance characteristics for detecting GA and for predicting progression from intermediate AMD to GA in the short term. The area under the curve (AUC) was 0.945 for the current year and 0.937 for the following years.
“Of note, when we added the contribution of human expert OCT gradings that took a lot of labor and a lot of NIH and Genentech funding over many years, we only improved the AUC by 0.08. The model was more effective and did not require the expert reading by humans to augment the response,” she said.
Further validation in additional data sets is currently underway, and potential modifications will be evaluated for outcome prediction in other retinal diseases.