Mortality prediction models for kidney failure may introduce bias, applicability concerns
Key takeaways:
- Researchers found 54% of studies had risk for bias due to inadequate population selection.
- Moreover, 30% of studies had shortcomings in how predictors were measured.
Current mortality prediction models for patients with kidney failure may introduce bias and applicability concerns in clinical practice, according to a large systematic review.
“Risk prediction models are increasingly endorsed to help patients understand their treatment preferences and promote personalized care. These models are of particular value for people with kidney failure,” Faisal Jarrar, MD, a resident physician at the University of Calgary Cumming School of Medicine in Alberta, Canada, wrote with colleagues. “Personalized risk predictions can inform the management of kidney failure for an individual and support decisions that best reflect their unique goals, preferences and values. Yet, current guidelines do not include any recommendation to consult a mortality prediction model.”
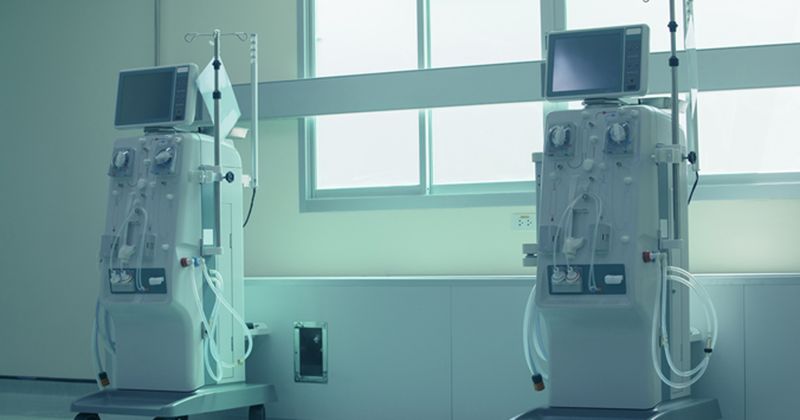
Researchers analyzed 7,184 abstracts published between 2004 and 2024, that included 2,963,157 patients with a median age of 64 years. Overall, 42% of patients were women.
Studies that evaluated prediction models in this population included patients with kidney replacement, hemodialysis or peritoneal dialysis. Reviewers independently graded each study as low, high or unclear in its bias and applicability risk.
Of the abstracts analyzed, 77 made full-text review and 50 met eligibility criteria. Researchers found 54% of included studies were at high bias risk due to inadequate study population selection; 30% had shortcomings in how predictors were measured; 24% had concerns in how outcomes were measured and all 50 studies were judged to have flaws in the analysis strategies.
The researchers also highlighted applicability concerns as 62% of study participants, 34% of predictors and 10% of outcomes did not align with the intended clinical setting. Overall, 2% of studies used decision curve analysis and 30% had tools to enhance model usability.
“We advocate for the use of existing guidelines and checklists to design, conduct and report prediction modeling studies and the involvement of stakeholders in the study design to enhance model usability and clinical uptake,” Jarrar and colleagues wrote.