Researchers develop a long-term survival model for patients on dialysis
Click Here to Manage Email Alerts
Key takeaways:
- Previously designed clinical prediction models for hemodialysis have not been effective.
- Clinical prediction models can help guide clinicians regarding vascular access choice and advanced care planning.
Researchers have designed a clinical prediction model to determine short-term mortality and long-term survival among patients on hemodialysis.
“Individuals undergoing maintenance hemodialysis (MHD) are at high risk of mortality, with median life expectancy of approximately [4] years,” Benjamin A. Goldstein, PhD, of the department of biostatistics and bioinformatics at Duke University School of Medicine, and colleagues wrote. “However, somewhat less recognized is the substantial variability in mortality in which 20% of patients die within the first year of MHD initiation while 40% will live for more than 5 years.
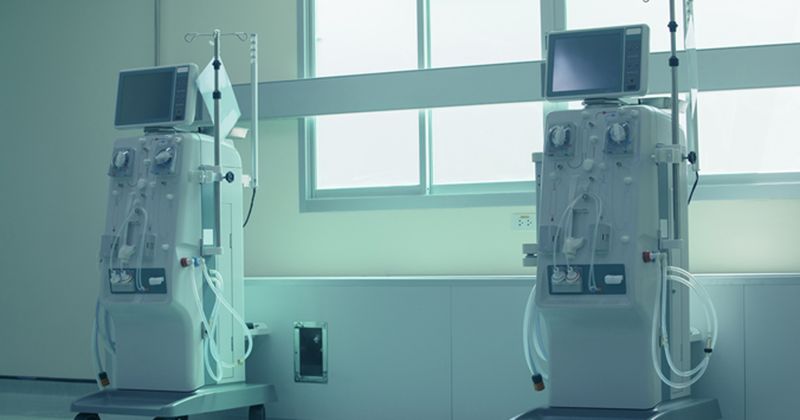
“These dramatic differences in life expectancy can impact many clinical and patient decisions, such as timely referral for a kidney transplant, choosing an optimal dialysis access, intensity of dialysis care, preventive health measures and invasive procedures, among others,” Goldstein and colleagues wrote. “In this context, clinical prediction models (CPMs) have the potential to provide objective information regarding life expectancy that could affect clinical decisions” as well as assist in advanced care planning and vascular access management, they wrote.
Due to poor design, however, CPMs are not widely used, the researchers wrote. “While a variety of CPMs have been reported in the literature, many of these were not well designed to be easily implementable ... prediction of longer-term survival for patients on MHD is important to guide decisions around preventive care, such as cancer screening and primary cardiovascular disease prevention or invasive procedures, such as implantable defibrillators or pacemakers,” they wrote.
Because patients routinely receive MHD three times per week and dialysis providers collect vital signs information, including regularly monthly labs, “the resulting rich electronic health record (EHR) data is ideal to support CPMs that can be implemented at the point of care,” the authors wrote.
Medical records
The researchers reviewed medical records from 42,351 patients during the course of 11 years who received care at Dialysis Clinic Inc., a mid-sized non-profit dialysis provider. Data from the provider EHR system were linked with U.S. Renal Data System Standard Analytic Files to assess mortality and transplant events. Patient data included demographics, laboratory results, vital signs and service utilization.
“For each patient month, we ascertained death within the next 6 months and survival over more than 5 years during receipt of MHD or following kidney transplantation (ie, long-term survival),” the authors wrote. “We compared these to time-to-event models spanning both time horizons” and “explored the performance of decision rules at different cut-points.”
Long-term survival
Results showed that long-term survival models had significantly better performance than near-term mortality models. Time-to-event models performed similarly to binary models. “Applying different cut points spanning from the [first] to 90th percentile of the predictions, a positive predictive value (PPV) of 54% could be achieved for near-term mortality, but with poor sensitivity of 6%,” the authors wrote. “A PPV of 71% could be achieved for long-term survival with a sensitivity of 67%.”
The authors wrote that development of a CPM illustrates “the potential for predicting long-term survival ... we hope it may counteract some of the nihilism inherent in treatment of patients on MHD due to many failed clinical trials among all-comers.
“Future work should validate these models and seek to consider how best to implement and use such tools in clinical practice. This includes engaging with important ethical questions of assessing and making care decisions based on mortality risk,” the authors wrote.