How artificial intelligence impacts the treatment of kidney disease
Health care is entering a new era where the advent of “big data” brings tremendous opportunities to revolutionize the profession. In order to be purposeful, data must be analyzed, interpreted and used to improve patient care. Exploring the associations among different pieces of information derived from large and diverse datasets to enable intelligent and informed decisions is now possible with the emergence of artificial intelligence (AI).
AI is “the science and engineering of making intelligent machines, especially intelligent computer programs” as described by the pioneer Alan Turing in 1950.1 AI is considered the fourth generation of the industrial revolution, since it is anticipated to transform and impact every possible sector of our lives, just like electricity did in the early 1900s (see Figure 1).
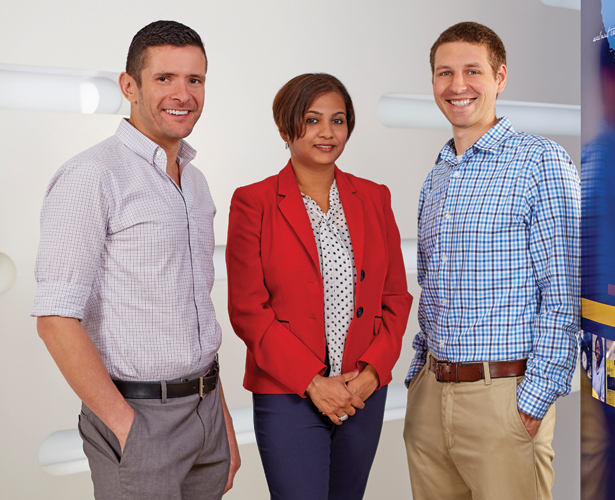
Source: Gerry Evelyn
AI in kidney care and health care uses algorithms and software engineering principles to approximate the decisions made by clinicians in the analysis of complex health care data. Traditionally computer-based algorithms in health care include a set of rules encoding expert knowledge on medical decisions. These rules are subsequently applied to draw conclusions about specific clinical scenarios. AI algorithms, however, strive to learn from the data without concrete rules.
In the profession of nephrology, various initiatives have incorporated machine learning (ML). One such example is with a ML model developed to predict CKD progression. The model was developed and validated using demographic, clinical and the most recent laboratory data from two independent Canadian cohorts of patients with CKD stages 3 to 5.12 This CKD progression model has been validated externally and in international populations,13,14 and is used for triage of CKD care in Canada.15,16 Other studies have developed ML models to accurately predict outcomes and graft survival after kidney transplant.17,18 ML models have just begun to be applied in health care and have the potential to improve patient care paradigms when implemented at the point of care in clinical applications in nephrology.
AI in kidney care
Large dialysis organizations (LDOs) have been at the forefront of the big data revolution, which has contributed a vast number of epidemiological insights to the literature. Fresenius Medical Care (FMC) is a large integrated kidney disease care organization that includes an LDO, a specialty pharmacy, outpatient endovascular centers, central laboratories, renal care coordination and management, software tools for nephrologists, and medical device and drug companies, among many others. In North America alone, this LDO has collected demographic, clinical, treatment and laboratory de-identified data from more than one million patients, which can be harnessed to train ML models (see Figure 3). Data from this large integrated kidney disease care organization have been leveraged to develop, pilot and deploy several ML predictive models that provide clinical decision support to aid clinicians and improve care models and ultimately, the quality and quantity of life of patients with CKD and ESRD.
Examples of some of the ML models in the dialysis clinic operations environment include a 12-month (long-term) and 7-day (imminent) hospital admission risk stratification in patients on in-center dialysis.19,20 Implementation of reporting risk stratifications and subsequent patient-centered clinical evaluations and interventions have revealed positive initial results, including a 23% decrease in 12-month hospital admission rates.20
Another AI tool deployed includes a CKD progression model that identifies the trajectory of the eGFR based on two or more historic values.21 The model has been integrated in an EMR system by Acumen Physician Solutions and is being used in nephrology practices across the United States to aid in confirming patient prognosis and providing patient education. Preliminary signals based on limited adoption indicated nephrologists who use the tool had about a 2% lower rate of central venous catheters in patients who progressed to ESKD and initiated hemodialysis (HD). Other models developed include an end-of-life ML model and a prediction model to identify a decreasing trend in overall functional status in patients on dialysis.22 These models might have utility in identifying patients who could be assessed for timely palliative care.
AI in home dialysis
For home therapy, we have developed an ML model to predict patients on PD who are at risk of developing peritonitis in the next month. Early detection of peritonitis could reduce the risk of occurrence of severe infections in patients on PD.
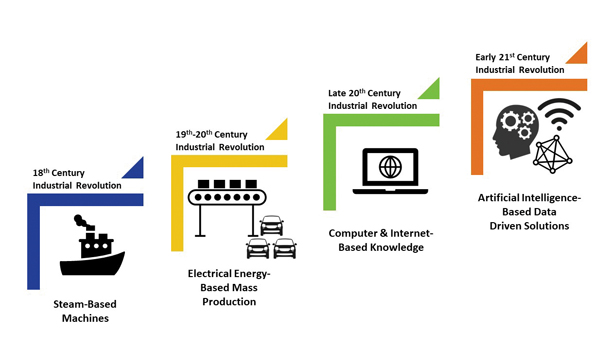
Source: Fresenius Medical Care
To identify the risk of peritonitis, an ML model was trained on historical infection and patient data types (see Figure 4) to identify which active patients on PD were at risk of developing peritonitis within the next month. The model was trained using a machine learning algorithm called XGBoost classifier.23 The performance of the model was evaluated using area-under-the-receiver operating characteristic curve24 which was about 0.66.25
Beginning in December 2018, the peritonitis ML model was used in a nationwide rollout in the United States. The ML model generated risk scores on a monthly basis for active patients on PD treated by the LDO within its integrated kidney disease care organization. As one of the top predictors of peritonitis is that the patient is new to PD, the clinical team scheduled a nurse home visit for all patients within the first 30 days of PD and a phone call between 30 and 90 days after starting the modality.
For the remaining patients, the ML model segmented patients into three risk groups for peritonitis. Nurse home visits were scheduled for high-risk patients and medium-risk patients received a phone call. An assessment was created to track actions, vital assessments and specific interventions performed for each phone call and home visit. Initial findings have seen a decreasing trend in the peritonitis episodes. A thorough analysis is ongoing to quantitatively assess the effectiveness of the new AI risk-directed care paradigm.
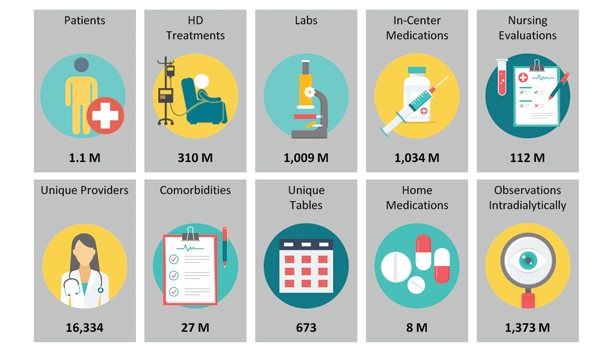
AI in vascular access
Renal Research Institute, a specialized research team with expertise in computational biomedicine and data analytics, is working on an AI-based classification model in collaboration with Azura Vascular Care, a network of outpatient vascular and ambulatory surgery centers, to detect and diagnose arteriovenous fistulae aneurysm (AVFA) stages in the United States. The team has developed a convolutional neural network (CNN) to automatically classify AVFA stages. CNN is a form of DL that is specifically applied for image recognition. CNNs consist of layers that receive an input image and perform mathematical operation to predict the output.26 The team has collected 15- to 20-second panning videos from 30 patients with two AVFA categories:
- AVFA stage 2 with enlarged AVF with hypopigmented skin; and
- AVFA stage 3 with enlarged fistula with open ulcer.
The extracted video frames were used to create an image set and a CNN model was trained using a cloud-based ML platform. CNN was able to automatically classify AVFA stages with greater than 90% classification accuracy. Using this model in a clinical application may reduce workload for physicians, provide timely AVFA diagnosis and improve patient care.
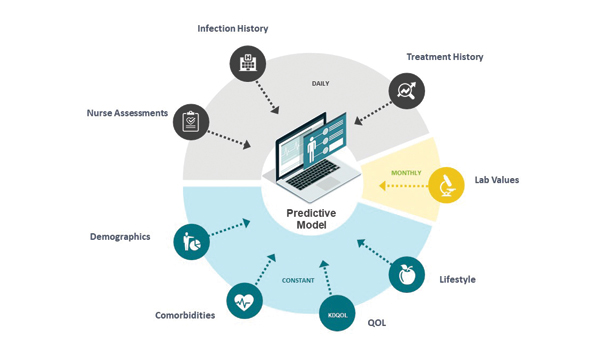
Source: Fresenius Medical Care
AI in anemia management
Outside the United States, the large integrated kidney disease care organization has successfully developed, validated and deployed an anemia control model (ACM) using ML that provides an individualized prediction of optimal erythropoietin stimulating agent (ESA) and IV iron dosing recommendations to maintain target hemoglobin (Hgb) and iron indices.27-30 The ACM is a class 1 medical device that has been approved to be marketed in the European Union (Council Directive 93/42/EEC). The ACM uses the ANN model to reproduce human erythropoiesis and erythrocyte lifespan in each patient and recommend a suitable ESA and IV iron dose upon each hemoglobin (Hgb) measurement received, with the aim to reach predefined clinical targets. This ML model considers an array of factors including the following demographics and current/historic factors:
- Hgb, transferrin saturation, and ferritin levels;
- ESA and IV iron dose, frequency and accumulation;
- weight, adequacy and nutritional status; and
- inflammatory status and mineral bone disorder status.
The ACM has been integrated into the EMR of the dialysis organization to optimize the anemia management process. Also, the ACM is being used by external dialysis providers in the European Union and recommendations are provided through an application.
Leveraging ML to individualize anemia management has yielded promising results. Barbieri and colleagues have found in retrospective analysis that the ACM’s prediction of Hgb levels 1 month in the future was less than or equal to 0.6 g/dL of the observed value.27 A multisite observational clinical trial of the ACM conducted in Czech Republic, Portugal and Spain identified that use of the ACM-guided management was significantly associated with 0.17 mg/kg/month decrease in the median dose of darbepoetin alfa, about 6% more patients on HD achieving Hgb targets; a decrease was seen in the fluctuation of Hgb levels over time, and a decrease in the rate of hospital days, cardiovascular events and transfusions.28 Figure 5 shows the longitudinal changes in Hgb levels and darbepoetin alfa dose before and after use of the ACM. Continued prospective testing in Spain has yielded consistent beneficial findings.30 Retrospective analysis of the ACM’s ability to predict Hgb levels 3 months in the future found predictions were within 0.75 g/dL of the observed value.29 This ML model appears to provide tighter control of anemia management, thereby minimizing ESA use and reducing the risks of negative outcomes, while yielding improvements in achieved targets and streamlining nephrologists workflows.
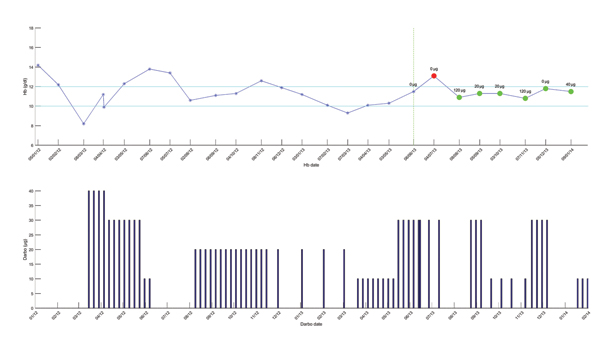
Source: International Society of Nephrology/ Elsevier
Conclusion
The rise of AI has the potential to redefine health care delivery. It should be viewed as a decision support tool to extend human insight and cognition and not something that will replace human medical decision-making on interventions. It should be employed to assist overburdened providers and support their endeavor of achieving best practices and outcomes for the patients they serve.
However, factors such as clinical effectiveness of an AI solution, and accountabilities in case of error, need to be carefully considered before implementing such solutions. Furthermore, policies and regulations need to evolve to guide the development of AI at an acceptable scale. Most importantly, the value of an AI solution to effectively deliver better outcomes needs to be demonstrated to patients, physicians, and providers to foster their trust.
- References:
- 1. Turing A. Mind. 1950;LIX(236):433-460.
- 2. Obermeyer Z, et al. N Engl J Med. 2016;doi:10.1056/NEJMp1606181.
- 3. Gupta T. Deep learning: Feedforward neural network. Towards data science. 2017;https://towardsdatascience.com/deep-learning-feedforward-neural-network-26a6705dbdc7.
- 4. Tran BX, et al. J Clin Med. 2019;doi:10.3390/jcm8030360.
- 5. Saran R, et al. Am J Kidney Dis. 2019;doi:10.1053/j.ajkd.2019.01.001.
- 6. Gargeya R, et al. Ophthalmol. 2017;doi:10.1016/j.ophtha.2017.02.008.
- 7. Gulshan V, et al. JAMA. 2016;doi:10.1001/jama.2016.17216.
- 8. www.fda.gov/news-events/press- announcements/fda-permits-marketing-artificial-intelligence-based-device-detect-certain-diabetes-related-eye.
- 9. Kann BH, et al. Oncology. 2019;33(2):46-53.
- 10. www.businesswire.com/news/home/20190206005175/en/current-health-receives-FDA-clearance-remote-patient.
- 11. Health Tech Pulse. 2019;https://healthtechpulse.com/2019/01/30/current-remote-patient-monitoring-reduces-readmissions-dartford-and-gravesham-nhs-trust.
- 12. Tangri N, et al. JAMA, 2011;doi:10.1001/jama.2011.451.
- 13. Lennartz CS, et al. Clin J Am Soc Nephrol. 2016;doi:10.2215/CJN.08110715.
- 14. Tangri N, et al. JAMA. 2016;doi:10.1001/jama.2015.18202.
- 15. Hingwala J, et al. Can J Kidney Health Dis. 2017;doi:10.1177/2054358117722782.
- 16. Smekal MD, et al. BMC Nephrol. 2019;doi:10.1186/s12882-019-1269-2.
- 17. Yoo KD, et al. Sci Rep. 2017;doi:10.1038/s41598-017-08008-8.
- 18. Molnar MZ, et al. Transplantation. 2017;doi:10.1097/TP.0000000000001326.
- 19. Long A, et al. Using artificial intelligence to help predict imminent hospitalizations in patients with ESRD [Abstract FR-PO791]. J Am Soc Nephrol. 2018;29:627.
- 20. Sweet D, et al. Effects of predictive modeling assisted care interventions on hospitalization rates in hemodialysis patients. J Am Soc Nephrol. 2017;28:635.
- 21. Han H, et al. Prediction of six months progression to end-stage renal disease. J Am Soc Nephrol. 2016;27:299A.
- 22. Maddux D, et al. Development of a novel functional status score for identification of dialysis patients who are candidates for palliative and hospice care. Presented at: the ISN World Congress of Nephrology; April 21-25, 2017; Mexico City.
- 23. www.analyticsvidhya.com/blog/2018/09/an-end-to-end-guide-to-understand-the-math-behind-xgboost/.
- 24. Narkhede S. Understanding AUC - ROC curve. Towards Data Science. 2018;https://towardsdatascience.com/understanding-auc-roc-curve-68b2303cc9c5.
- 25. Blanchard TC, et al. Abstract TH-PO408. J Am Soc Nephrol. 2018;29:221.
- 26. Gogul I, et al. Flower species recognition system using convolution neural networks and transfer learning. IEEE Xplore; 2017 Fourth International Conference on Signal Processing, Communication and Networking (ICSCN). 2017;Chennai:1-6; https://ieeexplore.ieee.org/document/8085675.
- 27. Barbieri C, et al. Comput Biol Med. 2015;doi:10.1016/j.compbiomed.2015.03.019.
- 28. Barbieri C, et al. Kidney Int. 2016; doi:10.1016/j.kint.2016.03.036.
- 29. Barbieri C, et al. PLoS One. 2016;doi:10.1371/journal.pone.0148938.
- 30. Bucalo ML, et al. Nefrologia. 2018;doi:10.1016/j.nefro.2018.03.004.
Disclosures: The authors report no relevant financial disclosures.
- For more information:
- Sheetal Chaudhuri, MS, senior director of Knowledge Management and Analytics, Dinesh Chatoth, MD, associate chief medical officer, John W Larkin, PhD, senior director of Publications and Research, Andrew Long, PhD, senior data scientist, and Len A. Usvyat, PhD, vice president of applied advanced analytics, are with Fresenius Medical Care North America in Waltham, Massachusetts. Hanjie Zhang, PhD, principal biostatistician, is with Renal Research Institute in New York. Isabella Cattinelli, PhD, senior data scientist, Luca Neri, MD, PhD, director of clinical and intelligent systems, and Carlo Barbieri, PhD, senior director of Clinical Systems, are with Fresenius Medical Care EMEA in Bad Homburg, Germany. Franklin Maddux, MD, is global chief medical officer with Fresenius Medical Care in Bad Homburg, Germany. Jeroen P. Kooman, MD, PhD, professor, department of internal medicine and nephrology, and Frank M van der Sande, MD, PhD, associate professor, department of internal medicine and nephrology, are with Maastricht University Medical Center in Maastricht, The Netherlands.