Back to basics on AI
AI is an umbrella term that brings together concepts from several fields such as statistics, algorithmics, machine learning, information retrieval and data science at large. Machine learning (ML), a sub-discipline of AI, is the scientific study of algorithms and statistical models that are able to learn how to perform a specific task without using explicit instructions by relying on patterns and inference instead. Consequently, ML algorithms are highly “data hungry,” often requiring thousands of observations to reach acceptable performance.2
The vast amounts of data collected in electronic medical records at the point of care provide a rich platform to employ ML. It thrives in handling enormous numbers of predictors and can combine them in nonlinear and highly interactive ways. This capacity allows for employing new kinds of data (eg, free text, images, sound and temporal data), whose sheer volume or complexity would previously have made analyzing them untenable.
Deep learning (DL) is another subset of ML that uses multi-layered artificial neural networks (ANN) to create more advanced non-linear feature engineering than traditional ML methods (see Figure 2).3
In a recent bibliometric study on the global evolution of research of AI in health care and medicine, it is evident that AI techniques and clinical applications of AI are relatively more common in professions like ophthalmology, oncology and cardiology.4 It is less prevalent in nephrology, even though the daily disease burden for patients with CKD and ESKD is higher compared to the other diseases.5
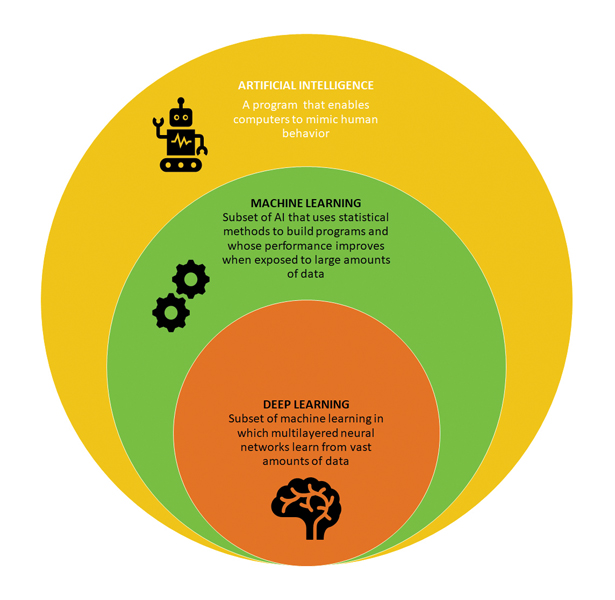
Source: Fresenius Medical Care
In ophthalmology, multiple AI-based grading algorithms have been developed to screen fundus photographs obtained from patients with diabetes and identify who should be referred to the ophthalmologist for treatment. DL models developed have had high reliability with 94% to 98% sensitivity and 93% to 98% specificity across differing models.6,7
The first AI-based device that was approved and permitted for marketing by the FDA was the IDx-DR (IDx Technologies Inc., Coralville, Iowa), which combines DL image recognition software to analyze retinal images and provide recommendations to refer patients for evaluation of diabetic retinopathy.8 In oncology, AI has helped with tumor imaging, pathology and clinical decision-making.9
AI has also entered the lives of patients in the form of wearable devices to monitor patients remotely and make personalized recommendations. Current Health’s AI-enabled wearable device measures multiple vital signs and has recently received FDA authorization for patients to use at home.10 The device can measure pulse, respiration, oxygen saturation, temperature and mobility. The device provides patients and physicians real-time updates and alerts on vital signs, which empowers patients in their care and allows physicians to address complications promptly. This technology uses ML to analyze continuous vital sign and kinetic data to detect unusual trends.
Currently, this device is mostly used by patients with chronic obstructive pulmonary disease and heart failure. AI-enabled remote monitoring may help improve hospitalization and mortality in such patients.11