Simpler forecasting models do not account for variability, uncertainty
In an article recently published in Proceedings of the Royal Society B, researchers argued that many of the simpler statistical models used to forecast the spread of infectious diseases may not appropriately account for random elements or uncertainty, and thus resulted in inflated early estimates of Ebola virus disease transmission.
“In the early days of the Ebola outbreak, a lot of people got into the forecasting business,” Aaron A. King, PhD, of the University of Michigan, said in a press release. “They did it using appealingly simple mathematical models, and the result was a series of warnings that alerted the world, quite rightly, to the seriousness of the situation … but in the end, most of those predictions turned out to be overstated.”
King and colleagues explored issues of model structure and data selection by comparing two variants of the susceptible–exposed–infectious–recovered (SEIR) model fitted to early data from the ongoing Ebola outbreak. The first, a deterministic version of the model, fits cumulative case count data to dictate trajectories under the assumption that any discrepancies come from measurement error, while the second, a stochastic version, is designed to account for uncertainty, measurement error and real variability in transmission.
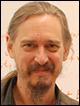
Aaron A. King
The researchers found that, due to its reliance on fitted data, the deterministic model did not account for uncertainty in key epidemiological rates. Additionally, difficulties communicating uncertainty could lead to overconfidence despite inaccurate results.
These issues, along with the relative ease and widespread use deterministic models provide, could continue to harm future outbreak forecasting.
“Deterministic models are easier and faster to work with, and the results look really good,” King said, “but when you use them, it’s a double whammy. Not only are you wrong, you are very sure that you are right.”
Stochastic models, on the other hand, afforded improvements in both of these areas by structurally accounting for variance and accurately measuring uncertainty. These methods are straightforward and computationally inexpensive, the researchers wrote, and should be used instead of the flawed deterministic models.
“Because stochastic models with greater realism, flexibility and transparency can be routinely and straightforwardly fit to outbreak data, there is less and less scope for older, less reliable and more opaque methods,” the researchers wrote.
Disclosure: King reports no relevant financial disclosures.