Deep learning score predicts PD-L1 status among patients with non-small cell lung cancer
Click Here to Manage Email Alerts
A deep learning score accurately predicted PD-L1 expression among a cohort of patients with non-small cell lung cancer who underwent PET/CT scans, according to study findings published in Journal for ImmunoTherapy of Cancer.
“This study is important, as it is the single largest multi-institutional radiomic study population of [patients with NSCLC] to date treated with immunotherapy who had PET/CT scans that were used to predict PD-L1 status and subsequent treatment response,” Robert J. Gillies, PhD, chair of cancer physiology and vice chair of radiology research at Moffitt Cancer Center, said in a press release. “Because images are routinely obtained and are not subject to sampling bias per se, we propose that the individualized risk assessment information provided by these analyses may be useful as a future clinical decision support tool pending larger prospective trials.”
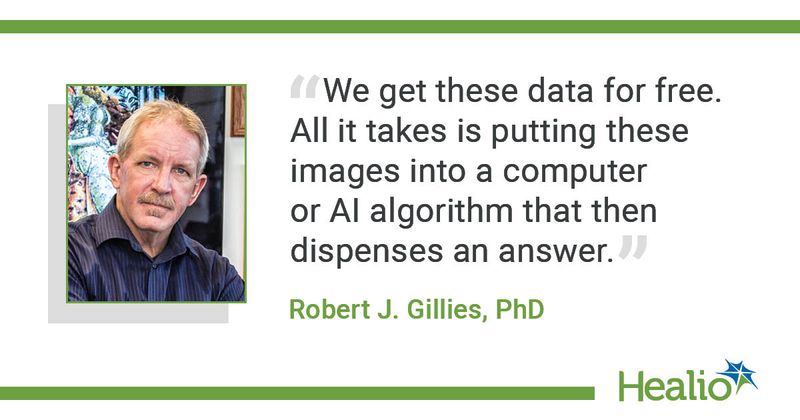
Gillies and colleagues developed a deep learning score to predict PD-L1 expression, durable clinical benefit, PFS and OS among 697 patients with NSCLC treated with immune checkpoint inhibitors across three institutions.
According to study results, the score enabled researchers to distinguish between patients with PD-L1-positive and PD-L1-negative status.
Gillies spoke with Healio about the research, what the findings showed and what future research will entail.
Healio: How are biomarkers currently measured for patients with NSCLC?
Gillies: The only biomarkers used for treatment decisions for patients with NSCLC are those obtained by biopsy and either running a genetic test to determine what driver genes are expressed or immunohistochemistry to see what proteins are expressed. One exception in common practice is fluorine 18-fluorodeoxyglucose (FDG) PET scan, which is used to identify patients who have multifocal disease. Generally speaking, when people are suspected to have late-stage cancers, they will get a PET scan to see if they have metastatic disease, which definitely changes the treatment schedule.
Healio: What are the drawbacks of that method?
Gillies: There is always a possibility of an adverse event, such as a pneumothorax resulting from the biopsy, but adverse events are rare. The biggest challenge with using either genetic or histology biomarkers is sampling. Cancers are heterogeneous, and the biopsy sample only requires a relatively small portion of the tumor, which may or may not reflect the status of the whole tumor. This is called a sampling artifact.
In genetic testing, the methods used are very robust. Immunohistochemistry is not as robust. The treatment decision for immunohistochemistry is 1% or 50% expression, so there is a lot of room for interpretation. Nonetheless, both tests suffer somewhat from sampling artifact and noninvasive imaging does not.
Healio: Why is there a need for an effective noninvasive approach?
Gillies: We have not done a very good job yet of quantitatively analyzing images to make treatment decisions. We do not yet have an approved and understood serum biomarker in lung cancer, although there are serum biomarkers available for other cancers — most notably PSA used in prostate cancer. Serum biomarkers can be obtained longitudinally, just like images, and they can be very informative. Serum biomarkers are commonly used to make treatment decisions — not just for cancer, but for many diseases. Specifically in lung cancer, however, it is limited to biopsy material. An advantage of noninvasive biomarkers, of course, is that they can be longitudinally monitored throughout the course of diagnosis, treatment and recovery, and treatments can be adapted depending on the response.
Healio: Can you explain the rationale for this approach?
Gillies: We are interested in multiple biomarkers, but the two primary markers — EGFR and PD-L1 — are known to affect the metabolism of cells that express it. FDG PET scans are a measure of glucose metabolism, which we felt would be reflective of general metabolic changes. We expected that patients with an EGFR mutation, for example, would have higher glucose metabolism. Interestingly, the biomarkers we identified were not simply reflective of how much glucose is metabolized, but where and how it is localized to different areas of the tumor — how heterogeneous this metabolism is that we are able to encompass in this single deep learning score. It is a very complex biomarker and it is not straightforward, but the numbers we observed were very good in terms of identifying patients who had the EGFR mutation or PD-L1 expression.
Healio: Has this approach been validated sufficiently to warrant widespread adoption?
Gillies: Not yet, but we have had discussions with several potential partners. We plan to use this signature in a prospective, observational trial that other investigators who have developed imaging biomarkers can join. We expect a multi-institutional trial wherein we use the images and whatever other data are part of the model to predict patient outcome. In this case, we will use models on the same data to predict both EGFR expression and PD-L1 expression. We also have a biomarker to predict adverse responses to immunotherapy. A small fraction of patients have a very bad response to immunotherapy, so we have come up with a model to incorporate that into our decision support model.
Healio: What are the implications if this approach becomes mainstream?
Gillies: PET scans are routine in developed countries or those at the cusp of development. They are widely available as part of standard of care because they are used for staging patients to determine whether they have multifocal disease. We get these data for free. All it takes is putting these images into a computer or AI algorithm that then dispenses an answer.
Healio: Is there a possibility for this approach to be effective beyond this patient population?
Gillies: Yes, absolutely. Not all cancers require routine PET scans. Notably, the application of these deep learning methods is not limited to PET scans. My colleagues and I — and many colleagues around the world — have developed models using CT scans, which are very routine across every cancer. The biggest challenge right now is working with the treating physician to use this information as the basis for treatment decisions, because there is no way they could be convinced by logic alone. They must be convinced by experience, and that is the purpose of a prospective, observational trial.
Healio: Is there anything else that you would like to mention?
Gillies: The next stage in radiomics — the quantitative analysis of imaging, whether it is deep learning or conventional — is basing treatment decisions on radiomics scoring, and several groups currently are testing those waters.
Reference:
Mu W, et al. J Immunother Cancer. 2021;doi:10.1136/jitc-2020-002118.
For more information:
Robert J. Gillies, PhD, can be reached at robert.gillies@moffitt.org.