Algorithm predicts short-term mortality among patients with cancer, may foster timely discussions of goals
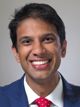
Machine learning algorithms identified patients with cancer who were at risk for short-term mortality and could benefit from immediate discussions about end-of-life preferences, according to results of a prospective study presented at Supportive Care in Oncology Symposium and published simultaneously in JAMA Oncology.
“On any given day, it’s actually pretty difficult to identify which patients in my clinic would benefit most from a proactive advanced care planning conversation,” Ravi B. Parikh, MD, MPP, instructor of medical ethics and health policy at University of Pennsylvania and staff physician at Corporal Michael J. Crescenz VA Medical Center, said in a press release. “Patients oftentimes don’t bring up their wishes and goals unless they are prompted, and doctors may not have the time to do so in a busy clinic. Having an algorithm like this may make doctors in clinic stop and [ask themselves], ‘Is this is the right time to talk about this patient’s preferences?’”
Previous studies have shown that machine learning algorithms, using electronic health record data, can accurately predict short-term mortality among patients in general medicine settings and, with oncology-specific algorithms, among those starting chemotherapy. However, it is unclear how various algorithms compare and whether they spur physicians to have timely conversations about treatment and end-of-life preferences.
Parikh and colleagues developed, validated and compared three machine learning algorithms — logistic regression, random forest and gradient boosting — to predict 6-month mortality based on structured EHR data prior to a clinic visit among patients with cancer.
The machine learning models analyzed predictors such as most recent albumin and alkaline phosphate levels, number of recent and total diagnostic codes for solid tumors without metastasis, and metastatic cancer. Sex, total or direct bilirubin, creatinine and hemoglobin lacked high importance in the models.
The models differed in some of the predictors they analyzed. For example, logistic regression analyzed anemia and red blood cells, random forest and gradient boosting analyzed lymphocytes, and gradient boosting analyzed neutrophils.
The researchers applied the models to 26,525 adults who had outpatient hematology/oncology or oncology encounters within the University of Pennsylvania Health System between Feb. 1, 2016, and July 1, 2016. Researchers randomly selected patients for training (n = 18,567) and validation (n = 7,958) cohorts and randomly selected an encounter in both groups. Patients underwent observation for at least 500 days following each encounter.
Six-month mortality from the date of the encounter served as the study’s primary endpoint, with 500-day mortality from the index encounter as a secondary endpoint.
Within 6 months, 1,065 patients (mean age, 67.3 years) died. Among patients still alive, mean age was 61.3 years.
At a prespecified alert rate of .02, researchers observed higher positive predictive values with the random forest (51.3%) and gradient boosting (49.4%) algorithms than with the logistic regression (44.7%) algorithm.
After adjustment for multiple comparisons, results showed no significant difference in the area under the curve (AUC) among the random forest (AUC = 0.88; 95% CI, 0.86-0.89) gradient boosting (AUC = 0.87; 95% CI, 0.85-0.89) and logistic regression (AUC = 0.86; 95% CI, 0.84-0.88) models (P for comparison = .02).
In the random forest model, observed 6-month mortality was 51.3% (95% CI, 43.6-58.8) among patients identified by the model as high risk vs. 3.4% (95% CI, 3-3.8) among those identified as low risk. After 500 days, observed mortality was 64.4% (95% CI, 56.7-71.4) in the high-risk group vs. 7.6% (95% CI, 7-8.2) in the low-risk group.
Fifteen oncologists who responded to a survey indicated that 58.8% of patients flagged as high risk by the gradient boosting algorithm were appropriate candidates for timely conversations about treatment and end-of-life preferences.
“We’re excited about the scalability of this decision-support method for providers, and not just in oncology,” Parikh said. “Our process of using machine learning to flag high-risk patients in real time is broadly applicable, and our approach risk-stratifies patients in a usable way that just hasn’t been available to us before.” – by John DeRosier
Disclosures: Parikh reports personal fees from GNS Healthcare and grants from Conquer Cancer Foundation, Penn Center for Precision Medicine, and Veterans Affairs Center for Health Equity Research and Promotion. Please see the study for all other authors’ relevant financial disclosures.