Deep learning: Getting better information to clinicians faster, cheaper and more accurately
Click Here to Manage Email Alerts
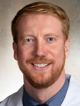
The phrase “art of medicine” underscores the fact that the practice of the profession is open to interpretation within the guidelines of scientific principles.
Mathematics is viewed as more rigid, guided by unyielding constants.
Stir in the power of computer-age analytics and you have a crossroads where science, mathematics and technology combine and create profound intersections of potential advancement.
“The modern era of health care is now inexorably linked to the application of mathematical and computational analysis to offer digital-age diagnostics, novel pharmacotherapies, computer-assisted and robot-assisted interventions, epidemiological data-mining and evidence synthesis,” Hutan Ashrafian, MBBS, PhD, MBA, clinical lecturer in surgery in the department of surgery and cancer at Imperial College London, wrote in The Lancet in 2013 when discussing the more than 300-year history of mathematics in medicine.
Alexander T. Pearson, MD, PhD, assistant professor of medicine in hematology/oncology at University of Chicago, is a formally trained “data generalist” who holds both an MD and PhD in statistics. He told HemOnc Today that his intersecting interests in oncology and data analysis come from his observation that “many of the principles that underlie cancer are mathematically oriented.
Pearson, a HemOnc Today Next Gen Innovator, co-authored a study published earlier this year in Nature Medicine that showed microsatellite instability (MSI) — a genomic mutational instability prevalent in many forms of gastrointestinal cancer — can be reliably predicted from hematoxylin and eosin (H&E)-stained histology slides using deep learning, a form of artificial intelligence. More recently, his research group used the same method to predict the presence of viruses in tumor samples.
The MSI findings could have significant clinical implications because H&E slides are routinely available for patients with cancer, yet evaluating for MSI requires additional genetic or immunohistochemical testing. By developing a proper pipeline and applying deep learning neural networks, clinicians can save time and money for patients while delivering faster personalized treatments, Pearson told HemOnc Today.
Question: What is deep learning?
Answer: As we implement it, deep learning is a way to perform regression analysis on visual data. Deep learning reduces visual characteristics into quantifiable groups of data that can then be associated with outcomes of interest.
Q: So, you are trying to make large quantities of data useful?
A: Yes, exactly. We use it for visual data — meaning visual digital pathology slides. But a deep neural network, which typically is the platform people are referring to when they talk about deep learning, is simply a neural network with hidden layers. A neural network is a complex set of interrelated equations that mimics human neurons to solve complex problems with potentially nonintuitive solutions. A deep neural network developed from images can — at increasing levels — build complex visual characteristics from simple things, like edges and gradients, into more complex things, like cheeks or noses if you are reconstructing faces. We use an approach called a convolutional neural network that extracts numerical information from pixels on a digital slide and then combines a function based on pixels with other adjacent functions. We can then relate simple features from a given image into more meaningful, higher-level characteristics — which, in our case, would be cellular architecture.
Q: How did your background and research interests lead you to arrive at combining artificial intelligence with oncology?
A: I was a statistics major in undergrad and then I did an MD/PhD program, with the PhD in statistics. That was during the first generation of statistics and methods development to analyze gene array data, in the GWAS (genome-wide association studies) period. In those days, it was about coming up with methods to analyze high-dimensional genomic data; we were interested in thousands of genes but could only afford to do gene array sequencing on a few hundred individuals.
I trained as a data generalist before the field of data science was a more well-developed concept. I had always been interested in cancer because many of the principles that underlie cancer are mathematically oriented. Cancer has an array of appealing problems from a computational or quantitative perspective.
During the second half of my physician-scientist training program at University of Michigan, I identified a group who were using mathematical modeling to help predict how to combine different chemotherapy agents. I used the data I collected from my post-doc experiments to build mathematical models of how different chemotherapies should be combined. It’s still a major initiative in my lab, and we have been building platforms to help accurately quantify the behavior of single cells and that of multiple cells interacting with each other to explicitly feed into 3D mathematical models of those same cell subtypes. We have been trying to figure out how tumor cells and immune cells interact and how to build a model to represent that interaction.
The unifying theme is that I’ve always been interested in finding new ways to reduce the extreme variation in biological systems, like cancer, into a tangible, quantifiable distillate that we can analyze or make sense of using existing mathematical tools.
Q: How can deep learning be applied to histology samples to predict MSI in a clinically useful way?
A: The really appealing aspect of deep learning applied to histology is that, if you have a functional platform, you can apply the computational technology almost instantaneously and at a very low expense to produce test results that cost hundreds to thousands of dollars. Our lab’s pipeline, which uses deep learning analysis of H&E slides, starts with a digital slide taken at our institutional digital slide scanner. We take a high-definition image of that slide, upload it to our storage and run the neural network evaluation of the area of tumor in the digital image. Finally, it produces a prediction result.
All of this can happen at scale, within hours, and at a cost of less than $10 per sample. When we thought about how to bring cutting-edge concepts like molecular targeting to the most people, we were really impressed with deep learning’s ability to expand the breadth and reach of molecular and personalized therapy. It is possible for a patient to get a biopsy anywhere in the world and get a digital copy of that biopsy uploaded to a server. It would be phenomenal to watch a biopsy being taken, uploaded and analyzed while the patient waits in the clinic.
Q: What makes MSI such a reliable predictor of a patient’s response to immunotherapy in gastrointestinal cancer?
A: MSI is a phenotype of impaired DNA mismatch repair within a tumor. This corresponds to an increased likelihood of response recovery following treatment with immune checkpoint inhibitors. Pembrolizumab (Keytruda; Merck) received FDA approval in 2017 for metastatic and unresectable MSI-high tumors based on this high degree of response.
Q: What are the impediments to testing every patient with cancer for MSI?
A: For colorectal cancer, MSI testing is part of the standard of care. It is a genetic test. We could bypass the time and cost of those genetic tests if we could further improve our approach. Further, MSI is not limited to colorectal cancer, but low prevalence and high testing cost limits testing across all cancers. Potentially, this barrier could be overcome with a deep learning approach. Although we aren’t there yet from a biomarker performance perspective, we would love to improve our false-negative and false-positive rates until they are perfect.
Q: How are you using deep learning at your lab?
A: We are exploring the ability to extract all kinds of information. To my knowledge, no one has directly deployed this method prospectively in clinical trials, and that is something that we are interested in moving toward.
Q: Are you applying your method retrospectively to existing data?
A: Yes. In both the virus detection and MSI prediction papers, we used a combination of image data from Cancer Genome Atlas, as well as single-institutional validation cohorts.
Q: What are the limitations to applying your method in active clinical practice?
A: There are two obstacles to overcome. The first is the availability of a large cohort of high-quality images with excellent and accurate annotations with which to train a neural network. The second is the target of interest has to directly influence the architecture of the cancer. This may not work for every standard-of-care genetic or clinical annotation, meaning if two cancers grow similarly in two patients with different genetic backgrounds, our network may not necessarily be able to tell them apart based on the histology architecture.
Q: Is there anything else clinicians should know about the possibility of deploying deep learning in the treatment of gastrointestinal cancer?
A: We hope that deep learning as a form of artificial intelligence will improve accuracy and speed diagnosis for patients with cancer. It should be used to augment the armamentarium of available tests. The goal is to get better information into the hands of clinicians faster, cheaper and more accurately. – by Drew Amorosi
References:
Ashrafian H. Lancet. 2013:doi:10.1016/S0140-6736(13)62542-8.
Kather JN, et al. Nat Med. 2019;doi:10.1038/s41591-019-0462-y.Kather JN, et al. bioRxiv. 2019;doi:10.1101/690206.
For more information:
Alexander T. Pearson, MD, PhD, can be reached at Duchossois Center for Advanced Medicine — Hyde Park, 5758 S. Maryland Ave., Chicago, IL 60637; email: apearson5@medicine.bsd.uchicago.edu. Follow him on Twitter at @lab_pearson.
Disclosures: Pearson reports no relevant financial disclosures.