Artificial neural network shows potential for assessment of glioblastoma treatment response
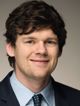
Researchers from Heidelberg University Hospital and the German Cancer Research Center have developed a novel technology that involves machine learning methods trained on standard MRI to assess treatment response among patients with glioblastoma, according to a press release.
“In the future, we want to advance the technology for automated high-throughput analysis of medical image data and transfer [the technology] not only to brain tumors, but also to other diseases such as brain metastases or multiple sclerosis,” Klaus Maier-Hein, PhD, head of medical imaging computing at Heidelberg University Hospital in Germany, said in a press release.
Maier-Hein and colleagues sought to overcome the limitations of manual assessment of glioblastoma by developing a framework relying on artificial neural networks for fully automated quantitative analysis of MRI in neuro-oncology.
According to results of the retrospective study, the technology was more reliable and precise than standard radiological methods.
Maier-Hein told HemOnc Today about what prompted the development of the technology, the results of the study and plans for additional research.
Question: What prompted the development of this technology ?
Answer : One of the essential criteria for the precise assessment of the efficacy of a new brain tumor therapy is the growth dynamic, which is determined by MRI. However, determining tumor progression using the established Response Assessment in Neuro-Oncology (RANO) criteria has severe shortcomings — measurements of the tumor volume are derived from two-dimensional perpendicular diameters in an MRI image slice that is manually chosen by the clinician. This type of measurement, performed reasonably fast in clinical practice, is inherently inaccurate and poorly reproducible.
We designed an artificial neural network capable of generating a volumetric segmentation of the tumor within 10 minutes and without manual intervention by the clinician. Aside from taking away subjectivity and poor reproducibility, volumetric segmentations provide more precise estimations of tumor progression.
Q: How did you conduct the study?
A: We collected three different retrospective data sets from patients with brain tumors. Each case had four MRI modalities — pre- and post-contrast T1, T2 and fluid-attenuated inversion recovery.
All cases were annotated semi-automatically by experienced radiologists as reference annotations. We used a single institutional data set that we acquired in Heidelberg, Germany, that included 455 MRI scans to train and develop our artificial neural network. We additionally used two data sets that involved longitudinal imaging information for testing the algorithm. One data set consisted of 239 MRI scans from 40 patients. To demonstrate the robustness and generalizability of our artificial neural network, we also used an external multi-institutional data set consisting of 2,034 MRI scans from 532 patients acquired across 34 different institutions and included in the EORTC-26101 study. The second data set included RANO-based measurements and time estimates for tumor progression. These measurements were conducted twice, independently of each other.
Q: What did you find?
A: Our study showed excellent agreement between the automatically generated segmentations and a radiologist-generated reference segmentation (0.91 median DICE score for enhancing tumor region; 0.92 median DICE score for edema on the EORTC-26101 test set). The disagreement between time to progression derived from manual vs. automatic segmentations (13%) was substantially lower than the disagreement between local and central RANO assessment (49%). Further, time to progression based on tumor volumes derived from automated segmentations proved to be a better surrogate endpoint for OS compared with the central RANO assessment.
Q: What should clinicians take away from this?
A: Tumor volumetry based upon segmentations has clear advantages over the two-dimensional measurements typically used in clinical practice. Creating volumetric segmentations, however, is tedious and requires substantial effort if done manually or semi-automatically. The artificial neural network developed in the context of this work is capable of creating high-quality annotations that offer more precise and reliable tumor progression analysis fully automatically. We have demonstrated an XNAT-based integration of our algorithm into the workflow of the clinic, allowing segmentations and volumetric measurements of all patients treated at Heidelberg University Hospital in a seamless and fully automated fashion. The entire pipeline takes about 10 minutes per patient for processing.
Q: What is next for research ?
A: We plan to also validate the added value of automated quantitative volumetric assessment of tumor response compared with RANO in a prospective setting. One direct implication of the availability of automatically generated segmentations is the improved ability to derive tissue-specific imaging biomarkers in neuro-oncology at high throughput. Furthermore, the present methodology can serve as a blueprint for the application of artificial neural networks in radiology to improve clinical decision-making. – by Jennifer Southall
Reference:
Kickingereder P, et al. Lancet Oncol. 2019;doi:10.1016/S1470-2045(19)30098-1.
For more information:
Klaus Maier-Hein, PhD , can be reached at Heidelberg University Hospital, Heidelberg 69120, Germany; email:k.maier-hein@dkfz-heidelberg.de.
Disclosure: Maier-Hein reports no relevant financial disclosures. The study was funded by Medical Faculty Heidelberg Postdoc-Program, Else Kröner-Fresenius Foundation.