AI has potential to disrupt and transform cancer care, center director says
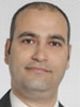
Translating genomic and big data into clinical tools for physicians and patients with cancer is an enormous challenge that has been enthusiastically accepted by Aziz Nazha, MD, hematologist and medical oncologist at Cleveland Clinic Taussig Cancer Institute.
A HemOnc Today Next Gen Innovator, Nazha was recently named director of Cleveland Clinic’s Center for Clinical Artificial Intelligence (CCAI), an initiative that the institution hopes will integrate AI and machine learning with patients’ clinical and genomic data and provide tools that lead to better decision-making and patient outcomes.
Nazha — also a self-taught computer programmer — spoke with HemOnc Today about how he and his students “who speak the two languages of computer science and medicine” have created a computer model at Cleveland Clinic that allows them “to push the envelope of these technologies in health care.”
Question: What are your impressions of how the CCAI is progressing so far?
Answer: It has been awesome but overwhelming at the beginning. We have received a lot of great responses from the media and from academic institutions inside and outside the United States that are interested in collaborating with us.
Q: What was the rationale behind the CCAI project?
A: I have been working on machine learning and AI since 2013, and I believe there is a huge opportunity for the application of AI in health care. In fact, we expect that health care will be the most disrupted industry by AI in the decade to come.
But the challenge in health care — which is often underestimated by technology companies — is the amount of complexity in the industry and how to apply these technologies. What we found is a huge disconnect among physicians, computer scientists and statisticians. Computer scientists can build models that may be accurate but are often difficult to explain to physicians and may not be applicable to health care. On the other hand, physicians usually have a traditional mindset about medicine, and it’s extremely hard to grasp the concept of machine learning.
The mission of the center is to harness the power of AI to transform health care, with a focus on patients. This is a unique center that will be physician-led. The purpose of the center will be to build what we call ‘physician data scientists’ — physician programmers who can understand machine learning and AI and try to apply these technologies in their research with the goal of improving patient outcomes — and health care in general — and decrease costs.
Q: What are some of the specific goals, or effects on outcomes, that you are looking to achieve with the use of AI and data analytics?
A: Currently, our main projects involve hematologic malignancies, and some current and future projects will be applicable to general medicine and intensive care.
To prove to our institution that this could be done by physicians internally, we put together data on approximately 1.5 million admissions to the Cleveland Clinic and tried to answer some questions using machine learning and AI. One of them was 30-day readmissions to the hospital. Another was length of stay. We tried to predict these variables, and were able to with high accuracy.
The accuracy was important, but perhaps more important was what we learned from putting together the model.
When we build the model, we typically extract the important features from it. In other words, we ask the algorithm, what are the important variables that impacted the outcome? By doing this, we achieve two goals: the first is to make sure the variables included in the model are clinically relevant, an important step that could be lost if it’s not done as a physician-led project; the other is to learn something from the AI system that we couldn’t learn from traditional statistics.
We took the project a step further and asked, can we predict gender using clinical data? Can we predict a patient’s race using clinical data? We were able to predict these with high accuracy — approximately 90% of cases.
We are building a cancer-specific model; there is really no cancer-specific readmission length-of-stay model, and we believe that patients with have different characteristics than general patient populations.
Q: In your collaborations on this project, are you receiving data from other institutions, as well?
A: Not too much yet. How to share data across multiple institutes is a very big topic.
We have put together a big project on patients with myelodysplastic syndrome (MDS) and wrote a personalized model that can predict patient-specific outcomes. We received data from collaborators in Europe and the United States for more than 2,000 patients. We presented this project at the ASH Annual Meeting and Exposition and it got a lot of attention. I had patients emailing me that they wanted to use the model.
We used a similar approach in building a model for patients with MDS who undergo transplant, and we are putting together manuscripts for both of these projects and hopefully soon will have the model available for investigators and patients to use.
Q: What are some of the practical uses for AI and the models you create? Can they be used to predict response to treatment?
A: One of the projects we have done and presented at ASH Annual Meeting in 2017 was trying to predict response to azacitidine in patients with MDS.
We used an algorithm that is somewhat similar to the ones used by video streaming services to make recommendations — if you watch this movie, this movie and this movie, then you may want to watch this movie. Similarly, our model said if the patient has this gene, this gene and this gene, then they will have a response or no response to treatment. By doing this, we were able to develop biomarkers that predicted resistance to treatment with approximately 80% accuracy. We validated the model using samples from a cohort within a prospective clinical trial.
We took this further by going back to the lab and used CRISPR-Cas to knock out those biomarkers and modeled the resistance in the lab. So, we have combined two of the most advanced technologies available today that can change humanity by building a platform where you can take what you find in the AI system, validate it in the lab and develop new therapies.
Q: How might the information you are generating at CCAI be used in guiding clinical decisions?
A: We are trying to make all these models available to physicians. It’s not as easy as one might think. We still have a lot of resistance from physicians because we are taking them out of their comfort zone. In the next couple of years, we will have better application, better understanding and better acceptance from physicians for this technology.
One of the things we are doing is trying to diagnose MDS by looking at the pathology slides, and this can be done for other cancers. If we look at the slides, can we predict the survival of the patient?
Another issue is implementing the technology. For example, we have built a web application where physicians can input patients’ clinical and mutational data and the output will be the survival that’s specific to patients with MDS. We are modifying the web application and hopefully we will make this available to physicians soon.
Q: How big of a challenge is it for physicians, many of whom resisted a switch to electronic medical records, to embrace AI and big data outputs?
A: It is a challenge, but its acceptance is getting much better. Everybody viewed AI and machine learning as a black box — but it’s not. We started explaining the model to physicians; once we started doing that, physicians began telling us that the data make sense.
There has been a lot of enthusiasm for our model from physicians around the world. The progress up to this point hasn’t come easy. We began working on this in 2013 and when we presented our project to ASH in 2015 and 2016, we were turned down. In 2017, we finally made it to an oral abstract presentation, and in 2018 ASH created a separate category for health care technology and AI — and it will be an accepted category for the first time at the next annual meeting.
Q: Some have argued that the use of AI in health care will eventually remove decision-making from physicians. What are your thoughts on this argument?
A: The way we view this technology is not human vs. machine, it’s a human plus machine. My work will get better when I have an algorithm that helps me to make some decisions, but the final decision is for me and my patients to make. This is why a tech company or a robot cannot be your doctor.
A lot of decisions in medicine are made between the physician and patient, and that involves a lot of expertise and intuition. By using our tools, we hope that a pathologist in the middle of Kentucky can be as secure in their diagnosis as a pathologist at Cleveland Clinic. Our tools won’t tell a radiologist a diagnosis but may highlight a portion of the scan that contains something important that they didn’t see or may have missed.
The goal is to augment and optimize data available to the physician to make better and more accurate diagnoses, not to replace the physician. We use the AI technology because we believe it has value; we don’t use it because of the hype around it. – by Drew Amorosi
References:
Nazha A, et al. Blood. 2018;doi:10.1182/blood-2018-99-118677.
Nazha A, et al. Blood. 2018;doi:10.1182/blood-2018-99-114774.
For more information:
Aziz Nazha, MD, can be reached at Taussig Cancer Institute, 10201 Carnegie Ave., Cleveland, OH 44195; email: nazhaa@ccf.org.
Disclosure: Nazha reports no relevant financial disclosures.