Novel risk stratification model outperforms standard prediction tools for myelodysplastic syndrome
Click Here to Manage Email Alerts
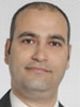
SAN DIEGO — A personalized risk prediction model developed using machine learning showed utility as a predictive and prognostic tool among patients with myelodysplastic syndrome, according to findings presented at ASH Annual Meeting and Exposition.
Results showed the new model better predicted mortality and transformation to acute myeloid leukemia than the standard International Prognostic Scoring System (IPSS) and Revised IPSS (IPSS-R).
Appropriate risk stratification is essential for this heterogenous patient population, Aziz Nazha, MD, medical hematologist and oncologist at Cleveland Clinic, said during his presentation.
“The survival for these patients can sometimes be measured over years but, for some of them, it’s over months,” he said.
However, he acknowledged that current risk stratification models may be insufficient.
“You can find some patients with low risk who behave worse than other patients with high risk,” he said. “This has made us ask the question of whether we can build a model that can provide personalized prediction for a given patient.”
It is also important to note the relationship between risk stratification and treatment plan, with chemotherapy and hematopoietic stem cell transplantation used for patients with very different risk profiles, according to Nazha.
Nazha and colleagues “harnessed the power of artificial intelligence and machine learning” to develop a model that included individual patient genomic and clinical data, Nazha said. The researchers then entered the data into a machine-learning algorithm and a web application with the aim of investigating OS and transformation to AML probability at various time points.
The analysis included 2,302 patients, including 1,471 (median age, 71 years; range, 19-99) in the training cohort and 831 in the validation cohort.
Sixteen percent of patients in the training cohort had progressed to AML, 11% had secondary myelodysplastic syndrome and 9% underwent HSCT.
According to IPSS criteria, 27% were low risk, 43% intermediate-1 risk, 19% intermediate-2 risk and 7% high risk. According to IPSS-R, 51% were very low/low risk, 23% intermediate risk, 13% high risk and 6% very high risk. Seven percent of patients had missing risk data.
Using their model, researchers identified the following characteristics — ranked in order —as most impactful on OS: cytogenetic risk categories by IPSS-R; platelets, mutation number, hemoglobin, bone marrow blasts percentage, 2008 WHO diagnosis, white blood count, age, absolute neutrophil count, absolute lymphocyte count, TP53, RUNX1, STAG2, ASXL1, absolute monocyte counts, SF3B1, SRSF2, RAD21, secondary vs. de novo myelodysplastic syndrome, NRAS, NPM1, TET2 and EZH2.
The group compared the ability of the new model to predict OS and transformation to AML compared with the IPSS and IPSS-R.
Results showed that the concordance index for the novel scoring system was 0.74 for OS and 0.81 for transformation to AML.
By comparison, the IPSS carried a concordance index of 0.66 for OS and 0.73 for AML transformation. For the IPSS-R, the C-index was 0.67 for OS and 0.73 for AML transformation.
“Compared with IPSS and IPSS-R, our model significantly outperformed these models,” Nazha said.
Nazha further reported that for OS, their model was prognostically superior to models based on genetic mutations only (C-index = 0.64), mutations plus cytogenetics (C-index = 0.68), and mutations, cytogenetics and age (C-index = 0.69).
Regarding AML transformations, the novel model bested models that considered mutations only (C-index = 0.72), mutations plus cytogenetics (C-index = 0.74), and mutations, cytogenetics and age (C-index = 0.75).
In the validation cohort, the novel method yielded a C-index of 0.8 for OS and 0.78 for AML transformation.
“With a machine learning approach and artificial intelligence, we are able to achieve much higher accuracy in terms of OS prediction as well as leukemia-free survival,” Nazha said. – by Rob Volansky
Reference:
Nazha, et al. Abstract 793. Presented at: ASH Annual Meeting and Exposition; Dec. 1-4, 2018; San Diego.
Disclosures : Nazha reports consultant roles with Karyopharma and Tolero, and being on the data monitoring committee of MEI Pharma. Please see the abstract for the full list of author disclosures.