Whole genome-based antigen testing may improve transfusion outcomes
Click Here to Manage Email Alerts
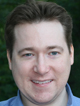
A whole genome-based approach to antigen matching of patients with blood donors may represent a novel method to improve blood transfusion outcomes, according to study results published in The Lancet Haematology.
Antigens on red blood cells can vary by individual.
Testing for compatibility before and after blood transfusion fails to account for all clinically important antigens, according to William J. Lane, MD, PhD, director of clinical laboratory informatics and assistant director of the Tissue Typing Laboratory in Brigham & Women’s Hospital’s department of pathology, and colleagues.
The researchers developed a novel method of antigen testing based on whole genome sequencing.
They performed three rounds of comparisons with bloodTyper, a computer software algorithm.
Lane and colleagues initially used whole genome sequencing data from 110 healthy adults in the randomized MedSeq trial to compare bloodTyper with conventional serology and single nucleotide polymorphism (SNP) methods for typing 38 red blood cell antigens in 12 blood-group systems and 22 human platelet antigens.
The researchers reported 99.5% concordance across the first 20 MedSeq genomes for the first algorithm. Investigators improved the algorithm and achieved 99.8% concordance for the remaining 90 MedSeq genomes.
After a final round of modifications, researchers validated bloodTyper with whole genome sequencing data from 200 participants in the INTERVAL trial, comparing the algorithm with conventional serology. Results of this analysis showed 99.2% concordance.
HemOnc Today spoke with Lane about the study, the potential implications of the results, and what needs to be confirmed in subsequent research.
Question: Can you provide some background about this study?
Answer: We started around 2012. There was a project to perform whole genome sequencing on 100 individuals, and investigators were interested in determining blood groups. I asked if I could be part because I was interested in how they were going to type blood groups from the next generation sequencing (NGS)-based whole genomes. When we were a few months into the project, it became clear that — although a lot was known about blood group genetics — there were major obstacles to applying that knowledge to analyze NGS data. For example, none of the published tables that aggregated the molecular findings from the last 20 or 30 years had been converted to the genomic coordinates used by NGS. Only a few people had done targeted NGS constrained to a small number of blood group genetic changes, so we had to build this from the ground up. The first step was converting the genomic coordinates. Then we curated all the allele tables. Then we started typing people. We did the first one manually. We realized that 99 more would be too much work, so we created computer programs to automate that analysis. It took a few years to recruit all 100 people but, over time, we slowly built up the algorithm and validated it. To get a sense of how well our approach worked, the Brigham and Women’s blood bank performed conventional serology and my collaborators at New York Blood Center performed conventional DNA array typing.
It took me about 4 months to do that first individual manually, and it took us about 4 years to do the other 99 people. These were followed by 10 African-Americans — largely underrepresented in the previous samples — so we could get a better sense of their genomics. We then added another 200 patients from the MedSeq sequencing trial in the United Kingdom, and the automated software analyzed them in just 4 days.
We really think this is a scalable approach for population-level data. It can be used for whole genomes for other clinical uses, or even as an inexpensive way for more targeted genomes for donors. The algorithm can read through the data and give back the types. This area is quite dense in terms of nomenclature. The allele changes are mostly SNPs, but not always. There also are structural rearrangements between genes. This can be a complex and challenging space to interpret manually if you’re not familiar with those specific genes.
Q: Does the algorithm need further validation?
A: There needs to be more validation. So far, we have validated for 38 antigens in 330 genomes. But this is one of these challenging aspects of large-scale genomic projects. If you have a number of changes, how do you validate all of them? We now are taking a more targeted approach, because there are some antigens that display rare phenotypes that might only exist in one of every few thousand individuals, or they may exist only in individuals of specific ethnicities.
Our goal with our first paper was to look at the changes found in the general population. Our goal now is to go back, identify individuals who already have been identified as having rare antigen phenotypes and validate those. Our other approach is to look at the large-scale genomic sequencing with collaborators in the UK to see if we can find unusual phenotypes in the genomic data and the validated serology using follow-up blood samples.
Q: So you have reasonably validated data for 38 of the more than 300 blood antigens ?
A: These are the antigens that vary within most people and, as a result, are those that most people make antibodies to. There also are economic concerns balanced with clinical concerns when thinking about what antigens to type for. This balance is why routinely we only upfront type and match for ABO and RhD in most people, while relying on screening for the presence of alloantibodies to determine if other antigens should be typed and matched for in that individual. However, for chronically transfused individuals — such as those with sickle cell disease — standard care is to extend phenotyping and upfront matching for approximately 10 antigens. These extended antigens have all been validated in this study.
Q : After you perform the validation steps you outlined above, what will be next for research?
A: We hope to start using this approach to inform transfusion decisions in clinical care. We hope to validate more antigens. Specifically, we are looking at antigens that are important for African-Americans, Rh changes and things like that. We also are looking for antigens that are present in one of 10,000 individuals or less. It is very hard to find samples from those individuals. You cannot go to one lab to find samples for all of them. However, if you have a large enough data set of sequenced individuals, you can find them and validate them in reverse. If you start producing this information back on large numbers of individuals, it will be interesting to see how donor centers and hospitals will be able to utilize this data when making transfusion decisions.
Q: How can this make its way into routine clinical practice?
A: We are working on cheap, affordable targeted assays to run on donors. The cost of typing the recipients likely to be transfused often is small compared with the overall health care cost. Let’s say you have a stem cell recipient or a patient with sickle cell disease, and you know they will need a lot of blood. It makes economic and clinical sense to more extensively type them. However, being able to match them with a supply of blood with either a rare or generally untyped antigen change or combination of changes is the expensive part. It requires screening thousands of donors for hundreds of antigens to be able to supply the blood. That is where the current array of technologies is being deployed. But they are still expensive and limited to about 40 antigens. We are creating technology to build out the number of antigens typed in blood donors. If you have an affordable assay that is automatically interpreted, you can use it to type the donor and supply, and build out your donor base. – by Rob Volansky
Reference:
Lane WJ, et al. Lancet Haematol. 2018;doi:10.1016/S2352-3026(18)30053-X.
For more information:
William J. Lane, MD, PhD, can be reached at Brigham and Women’s Hospital, 75 Francis St., Boston, MA 02115; email: wlane@bwh.harvard.edu.
Disclosure: Lane reports nonfinancial support from Illumina outside the submitted work.