Big data in bladder cancer: I call B.S.!
Click Here to Manage Email Alerts
I recently returned from Portugal, where I participated in a superb meeting on genitourinary oncology organized by the amazing Prof. Fernando Calais da Silva, a wonderful clinician-educator and researcher who has contributed greatly to the EORTC and Portuguese urology, and to the broader world of international urology.
This was an educational update meeting for highly talented and experienced Portuguese and Spanish clinician-investigators, including some superb urologists, radiation oncologists, medical genitourinary oncologists and pathologists.
The quality of discussion was very high and medically sophisticated. However, what bothered me was the number of times that publications using “big data” and erroneous guidelines were quoted as the (incorrect) basis for one practice or another.
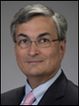
I was so heartened by the teenage students in Florida, who refused to allow themselves to be victimized by a crazed shooter nor by the politicians du jour, and who humiliated many of our leaders into rethinking the gun debate by repeatedly using the phrase “I call B.S.” to describe inaccurate or flawed nonsense arguments — hence, the title of this editorial.
I participated in an ASCO working party that published recommendations for use of observational research, which emphasized the many benefits and uses of observational research — application of large databases — but also emphasized the need for standards and structure in this use. Sadly, I believe that authors increasingly are using large sets of data without considering the following important caveats:
- Are the data accurate, and is the purpose of the database commensurate with the intent of the publication? In other words, is the nature of the data being captured consistent with the level of accuracy and stringency needed for the specific research project?
- Has there been appropriate training for those charged with entering data from routine records into large databases, or perhaps even capturing the data in the first place? The cadre of data managers and research nurses who execute prospective clinical trials and capture the derived data are mostly very well trained, and often have specific certifications for their work from the Society of Clinical Research Associates, a much higher level than needed for many large datasets.
- How rigorous is the attempt to exclude case selection biases? Many medical bureaucrats fail to understand that medical decision-making is a complex process, representing a balance between generic knowledge and patient-specific judgment, and it may be quite impossible to capture the important nuances of this process when listing that “patient A” received “treatment B.”
Allow me to cite two specific examples that were cited at the meeting in Lisbon as evidence to support points of view that I believe may be incorrect.
First, the old chestnut of the effectiveness of adjuvant chemotherapy for bladder cancer was thrown into the cauldron again.
The usual pro/con arguments were raised, including a specific study that attempted to overcome the problems of underpowered randomized trials by using “real-world data” with the tool of propensity matching — namely, a study conducted by excellent investigators using the National Cancer Data Base (NCDB) to compare outcomes of patients who did or did not receive adjuvant chemotherapy.
The problem is this: Despite a valiant attempt to balance the groups of patients drawn from NCDB to allow meaningful comparison, it was not possible to overcome selection bias.
I imagine that the authors, experts themselves, if they thought about it, would recognize the following: There is huge case selection bias when finding a patient who has been through a cystectomy, remains in reasonable shape, has not given up after the vicissitudes of surgery, wants to fight as hard as possible, and still has organ function — and sometimes stoma/reservoir function — that allows the clinician to believe it will be safe to give multiple cycles of cisplatin-based adjuvant chemotherapy.
How can it be possible to find an equivalent set of matched patients who have not received this postsurgical treatment for purposes of comparison? The concept is just nonsense, and yet this well-written study has now been cited by the National Comprehensive Cancer Network in its latest version of Bladder Cancer 5.2017 guidelines as evidence to support the use of adjuvant chemotherapy in this setting.
Without beating the authors of these guidelines, I remind them that they also cited the erroneous meta-analysis from the Cochrane/MRC group that assessed outcomes from six studies, including one that actually did not compare cystectomy plus adjuvant chemotherapy vs. cystectomy plus observation and salvage chemotherapy. They all seem to have forgotten an important Italian study that showed worse outcomes from adjuvant chemotherapy after cystectomy!
My second example, also quoted in Lisbon, is a study reported in JAMA Oncology that purported to study whether adjuvant chemotherapy improves survival for patients with adverse pathological features at radical cystectomy who previously received neoadjuvant chemotherapy.
One must admire that the participants in this conference were certainly up to date! The problem is that, once again, these authors used the NCDB and found 788 patients with pT3-4 and/or pN+ disease, of whom 23% were also given adjuvant chemotherapy and 76% were only observed. The usual amount of statistical mumbo-jumbo was applied by the authors to convince themselves that they were overcoming case selection bias, but there is just no way that statistical chicanery can truly overcome common sense and strong medical decision processes. Sadly, those who enter data into NCDB just don’t have the sophistication to define the nuances of medical decision-making so that one can reliably propensity match the cases in the two groups.
Thus, I call “B.S.” — which, in a former world, was called the GIGO principle. That said, I do wish to give true credit to the authors for noting that their paper was intended to be hypothesis generating, providing a basis for creating a randomized cancer trial to test the hypothesis. Although they are correct in their caveats, the reality is that many clinicians in the real world will read the abstract, consider the case closed, and subject their patients to additional torment.
As clinicians, we want to do the right thing, and there is an obvious desire to do “more” for the younger, fitter patient with extensive nodal disease after neoadjuvant chemotherapy and surgery. However, if added treatment doesn’t really improve prognosis, we are wasting time off treatment and fiscal resources of these unfortunate cancer victims, and they just become additionally victimized by incorrect use of big data. We really need to rethink this algorithm, and medical editors need to set much higher standards for the application of big databases.
References:
Galsky MD, et al. J Clin Oncol. 2016;doi:10.1200/JCO.2015.64.1076.
Seisen T, et al. JAMA Oncol. 2018;doi:10.1001/jamaoncol.2017.2374.
Spiess PE, et al. J Natl Compr Canc Netw. 2017;doi:10.6004/jnccn.2017.0156.
Visvanathan K, et al. J Clin Oncol. 2017;doi:10.1200/JCO.2017.72.6414.
For more information:
Derek Raghavan, MD, PhD, FACP, FRACP, FASCO, is HemOnc Today’s Chief Medical Editor for Oncology. He also is president of Levine Cancer Institute at Carolinas HealthCare System. He can be reached at derek.raghavan@carolinashealthcare.org.
Disclosure: Raghavan reports no relevant financial disclosures.