Value and P values
“Think of what you’re saying
You can get it wrong and still you think that it’s alright.”
— “We Can Work It Out” by The Beatles
Measuring value in cancer care is challenging.
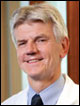
The day I wrote this column, I was in a meeting discussing what might be reasonable longitudinal outcomes for evaluating cancer care in our complex, multidisciplinary environment, where most data are collected around episodes of care.
The value models proposed by ASCO — discussed by my fellow Chief Medical Editor Derek Raghavan, MD, PhD, in the Sept. 25, 2015 issue of HemOnc Today — and by the National Comprehensive Cancer Network certainly have helped frame this discussion. However, the choice of outcome metrics is fundamental to the value proposition in cancer care — they have to be meaningful, reproducible and robust.
In the ASCO value framework, the gold standard is for efficacy of an intervention to be based on results from prospective, randomized clinical trials. Although some would argue effectiveness rather than efficacy is a more meaningful patient-centered outcome, it would be wrong to criticize ASCO for choosing an outcome which — unlike comparative effectiveness data — already is available for many common cancers. At least the society has begun to address this issue and adopted the most widely accepted efficacy measure available to us.
Positive vs. negative trials
Questions about the true reliability and impact of data from large randomized studies continue to surface, and two recently published reports revived this discussion.
The first was an excellent analysis of the scientific impact of positive and negative phase 3 cancer trials, written by Joseph M. Unger, PhD, and colleagues and published in JAMA Oncology.
Researchers looked at Southwest Oncology Group randomized phase 3 trials conducted between 1985 and 2014. They evaluated the impact factors for the published articles and secondary articles resulting from the trials, as well as their citation rates.
The primary conclusion from the study is certainly not new: Publication bias exists!
Of the 94 trials evaluated in this study, 26 (28%) were considered “positive” — defined as achieving a statistically significant result in favor of the new experimental treatment for the protocol-specified primary endpoint.
Interestingly, the researchers noted 38 of 40 “negative” trials also were published, concluding that there was no publication bias. However, they reported the positive trials were published in journals with significantly higher 2-year mean impact factors and were cited twice as frequently as negative trials. That seems like publication bias to me.
Followed over time, the researchers noted the citation counts for positive and negative trials eventually leveled out, such that their total scientific impact seemed to be roughly equivalent after several years.
This is reassuring from a scientific perspective and, as the researchers of the article and authors of the accompanying editorial point out, this is how it should be. If the purpose of randomized clinical trials is to explore the null hypothesis, we should expect to see a sizable proportion of negative studies, and their impact on practice should be regarded as no less important than the positive ones. Who would doubt the societal and direct patient benefit from randomized studies that showed no improvement in outcome for patients with early-stage breast cancer treated with high-dose therapy and autologous stem cell transplantation?
Further, as many commentators have pointed out, the true meaning to a patient of a statistically significant difference in a randomized trial is sometimes in doubt. Improvements in PFS or EFS measurable in days to weeks may be associated with impressive P values but do not necessarily translate into meaningful benefits for our patients.
Value of P values
As long as we are aware of potential publication bias, realize that statistical significance and clinical significance are not always correlated, and factor these into our value framework, the data from these studies, albeit subject to interpretation, are reliable — right?
Well, maybe not.
Statistical methods — which seemed elusive to me when I entered the oncology world — have become increasingly mystifying, but I have always thought I could rely to some extent on a P value to help me distinguish effectiveness. It turns out that may not be true.
In fact, the American Statistical Association (ASA) just issued a statement warning us about the interpretation or misinterpretation of P values. Most of us have thought a P value of .05 or less indicates a trial result is statistically significant. In other words, there is a 95% chance the null hypothesis can be rejected and the hypothesis is proved.
Apparently, it is not that simple. I get lost in some of the subtleties and semantics of this, so I will not pretend to understand the statistical details, but the bottom line is that the exact conduct of these analyses can have a profound effect on their interpretation and on the P value. As datasets become larger and more complex, and as the number of variables increases, the ability to obtain “false-positive” P values expands.
An amusing example of this — and the inspiration for The Beatles reference opening this article — is a study from Wharton Business School titled “False-positive psychology: Undisclosed flexibility in data collection and analysis allows presenting anything as significant.”
This is a great title for a great study in which the investigators, by choosing a particular method of analysis of their data, were able to show listening to The Beatles’ song “When I’m Sixty-Four” made a group of undergraduates about 18 months younger compared with a control group who listened to a tune used as background music.
These investigators and the ASA called for circumspection in the interpretation of P values and also recommend a new level of transparency in describing statistical methods. They also pointed to practices such as “P hacking” and “data dredging” — the search for small P values, often in subset analyses, that may overcome publication bias and get a body of work into print.
Data from prospective, randomized phase 3 trials are among the best metrics we have when exploring the outcome part of the value proposition. Recent reports show even these are flawed, and we need to look for other data sources to supplement them. Assessing effectiveness as well as efficacy — and exploring models that measure outcomes longitudinally — are some way in the future but badly needed to add more meaning to value in cancer care.
References:
Baker M. Nature. 2016;doi:10.1038/nature.2016.19503.
Huntington SF, et al. JAMA Oncol. 2016;doi:10.1001/jamaoncol.2015.6540.
Schnipper LE, et al. J Clin Oncol. 2015;doi:10.1200/JCO.2015.61.6706.
Simmons JP, et al. Psychol Sci. 2011;doi:10.1177/0956797611417632.
Unger JM, et al. JAMA Oncol. 2016;doi:10.1001/jamaoncol.2015.6487.
For more information:
John Sweetenham, MD, is HemOnc Today’s Chief Medical Editor for Hematology. He also is senior director of clinical affairs and executive medical director at Huntsman Cancer Institute at University of Utah. He can be reached at john.sweetenham@hci.utah.edu.
Disclosure: Sweetenham reports no relevant financial disclosures.