Cancer trials: Lies, damned lies and statistics
(with apologies to Benjamin Disraeli, Mark Twain or Leonard Courtney)
Click Here to Manage Email Alerts
Enrico Fermi, the famed Italian physicist who contributed to the development of quantum theory, reportedly commented: “Before I came here, I was confused about this subject. Having listened to your lecture, I am still confused, but on a higher level!”
As I review the pages of material that we publish in HemOnc Today, I am increasingly struck by the aptness of this quotation as a descriptor of our modern approach to the publication of cancer trial data.
It seems to me that the interpretation of data from cancer trials has become increasingly challenging because of the burgeoning sophistication of the available statistical tools and methods of display. This may be, in part, due to the different reasons for publishing a set of observations.
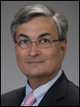
Derek Raghavan
It may be that there is fundamental self-interest as the driver — for example, an academician seeking fame or a pharmaceutical enterprise seeking fortune. In that situation, which may be compromised by innate conflict of interest, biostatistics actually can be used to obfuscate the truth or, alternatively, to heighten the perceived impact of the observation.
Once upon a time, it was relatively simple, and single-point comparisons were implemented. As oncology evolved as a research discipline, time-based and time-independent variables assumed increasing dominance with the ascent of nonparametric statistical manipulations and the emergence of the famed Kaplan-Meier plots and equivalents.
However, for these to be meaningful, large numbers of cases were required to demonstrate numerically small but clinically important statistically significant differences. This created a new set of problems for the rugged individualists in single institutions who wished to maintain their own primacy and independence (or patent protection) in reporting their data.
Confusion and misinformation
As a consequence, new tools were developed. Instead of completing a simple, well-powered and well-designed randomized trial, the biostatistical fraternity came up with the concept of meta-analysis, the overall analysis of sets of individual data or published trial data. This allowed parallel comparisons to be effected, strengthening the overall perceived impact by increasing the numbers of observed vs. expected outcomes.
Although this approach sometimes can be useful when trials are close to the requisite size and reveal similar outcomes, it becomes a quagmire of bias and confusion when one attempts to link multiple vastly underpowered studies with broad confidence intervals in the company of one or two large but still inadequately powered studies.
This applies especially if the fundamental design of some of the studies is flawed ab initio but lost in translation. Nonetheless, meta-analysis often is sufficient grist for the mills of the cadre of abstract-only readers who see the numbers, adopt the conclusion and continue on their merry ways, heartened by a new level of confusion and misinformation.
In this editorial, I just don’t have space to address my concerns about network meta-analysis, the technique in which larger data sets from multiple sources — comparing multiple endpoints — are used to try to achieve synchronous multiple and parallel outcome comparisons. Suffice it to say, there is an even greater potential for misinterpretation and inculcation of bias unless very carefully designed and interpreted by a fusion of statistical and clinical experts who understand the implications of unexpected interactions of the various factors being measured.
Sadly, riding closely upon the heels of the advent of meta-analysis, the application of the visually pleasing and statistically confusing (to the humble clinician) forest plot to create an apparently simpler visual tool for impact enhancement has now assumed greater prominence. The forest plot, so often characterized by long horizontal lines emerging in each direction from the middle point — representing huge confidence intervals — attracts the eyes of the innocent reader to the position of that middle point. Usually one’s focus is pointed there by the heady accompanying rhetoric that compares unity (1.0) to the midpoint of (say) 0.8, implying a reduced hazard of an adverse event, while ignoring the lack of statistical power and breadth of overlapping confidence intervals around unity. A case in point is the sad saga of non-data supporting the use of adjuvant chemotherapy for invasive bladder cancer.
Another example of potential misuse of statistical tools is the recent vogue for the application of the waterfall plot to every clinical trial! This also is visually elegant, as it allows one to draw little vertical lines (upward or downward) that represent surrogates for tumor progression or response, respectively. Thus, at a glance one can see what happened to the tumor in response to some novel therapy. Easy! Perfect! So … what’s the problem?
Well, the problem is that there are many ways of using this tool to deceive. Perhaps my least favorite is the loss-of-data trick. The next time you look at a waterfall plot, take a look at how many lines are drawn up and down (that is, the total of the data points shown) and check the number against the total number of cases entered into the trial. It’s amazing how often the numbers do not tally, meaning that someone has deleted an important set of data points from the analysis. Reasons, albeit inappropriate, might include absence of measurement, changes too small to illustrate, and deaths prior to tumor measurement.
The problem, of course, is that the visually pleasing demonstration of these artificially heightened response rates can so easily influence the reader to believe that the agent is more useful than it truly is, while losing the impact of toxic deaths or poorly disciplined clinical trial operations that have lost important quanta of measurement information. Linked to this is the suggestion that a reduction of (say) 10% to 20% in tumor volume is somehow clinically important, independent of duration of response or the actual real magnitude of the response. In some cases, small reductions plotted actually represent a volume change that is within observer error clinically.
Art of measurement
Recently, HemOnc Today published a very interesting cover story on practical implications of the genomic revolution, focusing somewhat on the need for training in this complex domain for oncologists, and the provision of more digestible, accurate information for patients and clinicians. That particular story did not address the complexities of genomic biostatistics, the strengths and limitations of Bayesian design in gene expression/mutation studies, and issues pertaining to the funding of experimental work in that domain. I don’t have space to address these issues but plan to do so in the future. That said, they form another part of this complex interplay between clinical and biostatistical science.
When I started my career in clinical and translational research, it was pretty easy to identify chicanery and misrepresentation of data. The much-esteemed Ian F. Tannock, MD, PhD, FRCPC, and others wrote elegantly in the early years of modern oncology, chiding our colleagues about the folly of inaccurate measurement and self-delusion.
Unfortunately, in the past few years, some of the biostatistical ploys have become more complex, less real and much more subject to abuse. In our enthusiasm to identify true progress, it has become increasingly important to add a much greater level of sophistication to the training of young oncologists in the art of measurement and biostatistical appraisal. Think about that the next time you read another breathless report of purported quantum leaps with actual changes of outcome most accurately expressed in days.
For more information:
Derek Raghavan, MD, PhD, FACP, FRACP, FASCO, is HemOnc Today’s Chief Medical Editor, Oncology. He can be reached at derek.raghavan@carolinashealthcare.org.
Disclosure: Raghavan reports no relevant financial disclosures.