Using noncentralized AI learning to diagnose skin cancer can improve patient privacy
Click Here to Manage Email Alerts
Key takeaways:
- Federated learning uses an institution’s own AI model and data that is facilitated by a central coordinator.
- It performed worse than centralized learning on a holdout dataset but better on an external dataset.
Federated learning may be a viable alternative to centralized learning for diagnosing invasive melanomas and nevi while maintaining patient privacy, according to a study.
Artificial intelligence (AI) models can improve diagnostic accuracy of skin cancer with the most common use of AI in skin cancer diagnostics being classical centralized learning, where patient data are transferred to one site for training and testing to develop AI algorithms. However, this AI approach has raised safety concerns.
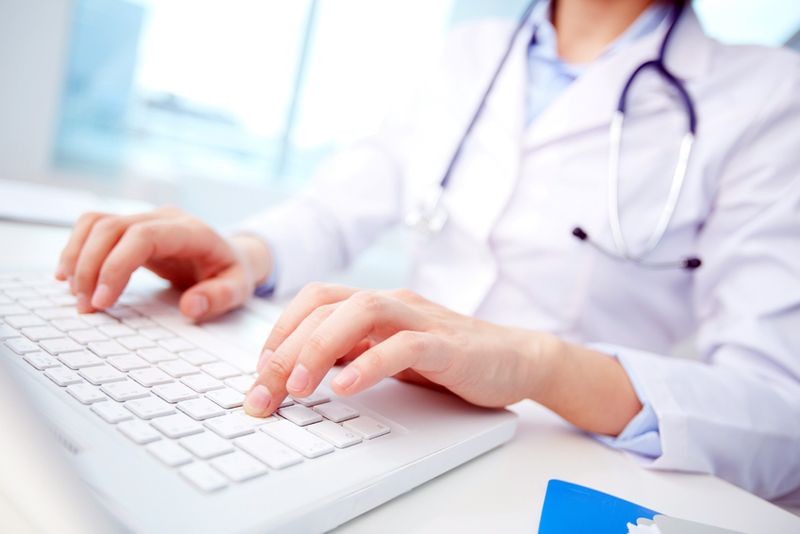
“In the medical field, ensuring patient data confidentiality is of utmost importance; consequently, sharing patient data is heavily regulated,” Sarah Haggenmüller, MSc, of the Digital Biomarkers for Oncology Group, National Center for Tumor Diseases, German Cancer Research Center, and colleagues wrote. “Thus, the transfer of patient data to an external facility to generate the envisaged algorithms can raise serious privacy concerns.”
Another type of AI learning called ensemble learning, where institutions can use their own data and computing power to develop separate AI algorithms that merges all decisions into one, is an alternative option to maintain privacy; however, clinics often face resource constraints.
To address these challenges, the authors evaluated a third approach called federated learning. Federated learning allows for an institution to train its own model with its own data while communication and aggregation are executed by a central coordinator.
In their study, the authors compared federated learning to centralized and ensemble learning using area under the receiver operating characteristic curve (AUROC) to judge each approach’s diagnostic performance.
Results showed that the federated approach (AUROC, 0.8579; 95% CI, 0.7693-0.9299) performed significantly worse than the centralized (AUROC, 0.9024; 95% CI, 0.8379-0.9565) and ensemble approach (AUROC, 0.8867; 95% CI, 0.8103-0.9481) on a holdout test dataset.
However, federated learning (AUROC, 0.9126; 95% CI, 0.881-0.9412) performed better than centralized learning (AUROC, 0.9045; 95% CI, 0.8701-0.9331) on an external test dataset, whereas ensemble learning performed better than both (AUROC, 0.9227; 95% CI, 0.8941-0.9479).
This has led the authors to conclude that federated learning has the potential to achieve at least an on-par performance to centralized learning making it “a reliable alternative for the classification of [invasive melanomas] and nevi,” they said.
“Additionally, [federated learning] empowers institutions to contribute to the development of AI models, even with relatively small datasets or strict data protection rules, thereby fostering collaboration across institutions and countries,” the authors added.