Convolutional neural networks show promise in management of hidradenitis suppurativa
Key takeaways:
- Convolutional neural networks (CNNs) were 78% accurate at distinguishing disease severity.
- CNN may be capable of registering slight changes of hidradenitis suppurativa inflammatory activity over time.
Convolutional neural networks may be able to detect hidradenitis suppurativa-affected skin and classify disease severity, according to a study.
A convolutional neural network (CNN) is a type of deep-learning system that can classify and analyze image data.
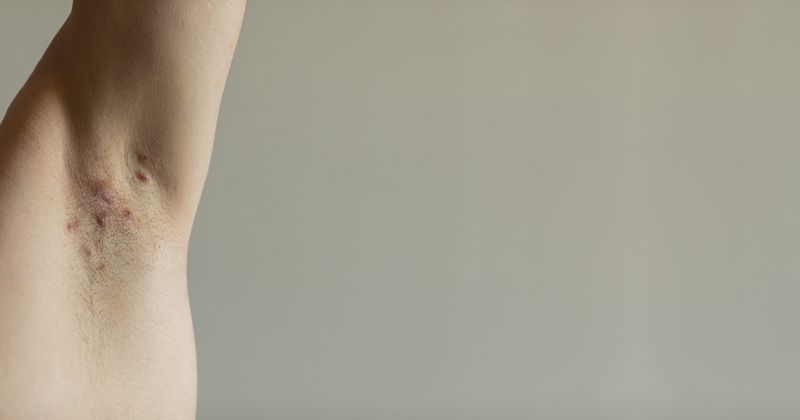
“While the underlying technology itself is not new, as CNNs have been used in medicine and dermatology for some time, the assessment of [hidradenitis suppurativa (HS)] using such an approach has not been reported to date,” Antonia Wiala, MD, of the department of dermatology at the Clinic Landstrasse in Vienna, Austria, and colleagues wrote. “This proof-of-concept study highlights that an automated HS-classification approach has the potential to simplify clinical practice and improve the consistency and reproducibility of clinical scores.”
In this prospective, single-center study, 777 ambient-light and size-controlled images from 149 patients were included in the CNN dataset.
Results showed that CNNs were 78% accurate at distinguishing no HS from mild disease and moderate disease from severe disease. The system also achieved the correct International Hidradenitis Suppurativa Severity Score System (IHS4) classification with 72% accuracy.
The study also found that disease dynamics using IHS4 numerical values aligned with CNN outputs, as reflected by the normalized root mean square error value of 0.2618. According to the authors, this indicates that CNN is capable of registering slight changes of HS inflammatory activity over time.
Lastly, the study found that CNN was able to detect lesional skin with pixel accuracy of 88.1%, and this aligned with physician-labeled HS skin. According to the authors, this feature may allow for the reduction of manually labelling different skin areas in the future.
“This study breaks new ground and provides an outlook for a future with fast, reliable, reproducible and easy-to-use disease severity assessments in HS patients,” the authors concluded.