Convolutional neural networks increase dermatologists’ diagnostic accuracy of skin cancer
Key takeaways:
- Specificity and accuracy rates were higher among convolutional neural networks (CNNs).
- Dermatologists with fewer than 5 years of experience saw increased diagnostic accuracy when considering CNN results.
The integration of convolutional neural networks into dermatology practices, especially those with fewer than 5 years of experience, may increase diagnostic accuracy of skin cancer, according to a prospective study.
“In skin cancer classification tasks, convolutional neural networks (CNNs) achieved diagnostic accuracies similar to trained dermatologists,” Julia K. Winkler, MD, of the department of dermatology at the University of Heidelberg in Germany, and colleagues wrote. “With the present study, we aimed to elucidate the cooperation of dermatologists with a market-approved CNN in a prospective clinical setting.”
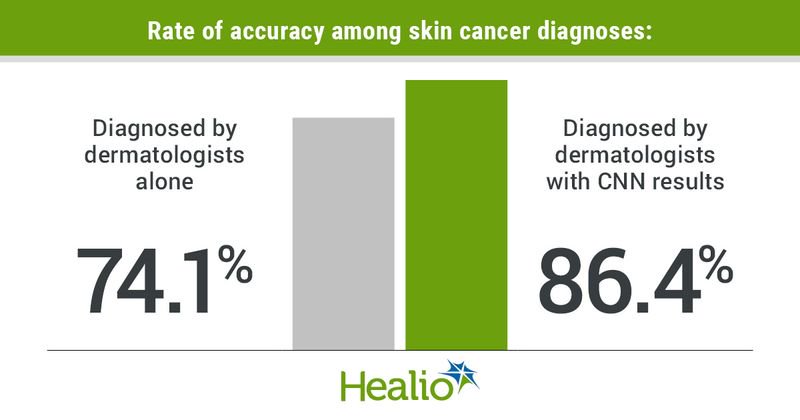
In this prospective, diagnostic study, 22 dermatologists with different levels of experience in using dermoscopy for skin cancer screenings performed full body examinations on 188 patients. Dermatologists then indicated the level of malignancy of suspicious lesions and suggested a management plan.
After this examination, patients were sent to a separate room for a CNN assessment. These results were forwarded to the dermatologists who were then asked to reevaluate their decisions based on the new findings.
Results showed that the dermatologists detected 228 suspect melanocytic lesions, which included 190 nevi and 38 melanomas.
When assessed separately, dermatologist findings were comparable with CNN in sensitivity (84.2%; 95% CI, 69.6%-92.6% vs. 81.6%; 95% CI, 66.6%-90.8%); however, CNN outperformed dermatologists in specificity (72.1%; 95% CI, 65.3%-78% vs. 88.9%; 95% CI, 83.7%-92.7%) and accuracy (74.1%; 95% CI, 68.1%-79.4%. vs. 87.7%; 95% CI, 82.8%-91.4%).
More importantly, when dermatologists integrated the CNN results into their decision-making, sensitivity increased to 100% (95% CI, 90.8%-100%), specificity increased to 83.7% (95% CI, 77.8%-88.3%) and accuracy increased to 86.4% (95% CI, 81.3%-90.3%).
Additionally, with the inclusion of CNN results, unnecessary excisions of benign nevi were reduced by 19.2% (P < .001).
The authors noted that the addition of CNN results mainly benefited dermatologists with fewer than 5 years of experience. Results showed that the diagnostic accuracy of dermatologists with fewer than 2 years of experience increased from 70.5% to 87.2% once the CNN results were included (P < .01).
Dermatologists with fewer than 5 years of experience followed a similar trend (77.1% vs. 91.7%; P < .01) whereas dermatologists with more than 5 years of experience saw no statistical increase with the addition of CNN results (74.1% vs. 75.9%).
“Dermatologists significantly improved their diagnostic performance when cooperating with the tested CNN,” the authors concluded. “These results indicate that a broader application of this human with machine approach, particularly in nonspecialized institutions, could be beneficial to clinicians and patients.”