Machine learning models improve prediction of mortality in patients with STEMI
Machine learning models enhanced prediction of mortality after STEMI in low-income countries, researchers reported in the International Journal of Cardiology.
“Multiple well-validated, population-based risk scores such as TIMI and GRACE are available to assess mortality following STEMI. However, these risk scores are based on logistic regression analysis, which has a number of shortcomings,” Mohit D. Gupta, MD, DM (Cardiology), FRCP (Edin), FACC, FSCAI, FESC, FCSI, FAPI, professor of cardiology at GB Pant Institute of Post Graduate Medical Education and Research New Delhi, and Arman Qamar, MD, MPH, interventional cardiologist and auxiliary research scholar at NorthShore Cardiovascular Institute, NorthShore Health SystemEvanston, Illinois, told Healio. “Additionally, these scores have been postulated and validated in the Caucasian population. This prompted us to utilize the data from the NORIN-STEMI registry to assess risk factors unique to low-and-middle income countries such as India and develop an artificial intelligence (AI) model based on it.”
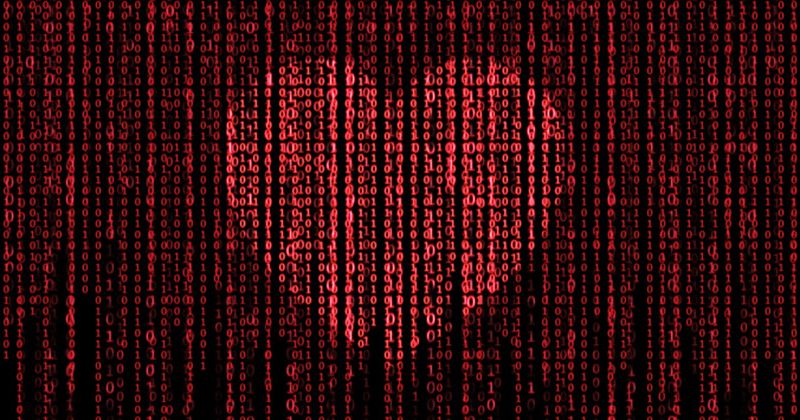
Testing of models
Using data from the prospective NORIN-STEMI registry of patients from northern India with STEMI, the researchers tested and trained all available machine learning models, and chose five for the analysis. The study population included 3,191 patients (mean age, 54 years; 16% women), of whom 2,668 were included in the development dataset and 523 were included in the validation dataset.

“Those five were shortlisted based on the consistency of machine learning model results on unseen dataset, and AI models for which AI interpretability algorithms can be integrated,” Gupta and Qamar told Healio.
The goal was to make mortality predictions as precise as possible, with high sensitivity in the validation dataset.
The rate of mortality at 30 days was 7.7%.
The Extra Tree Classifier, a tree-based method, had the best predictive performance of the models, with a sensitivity of 85%, accuracy of 75% and area under the curve of 79.7%, according to the researchers.
Sensitivity ‘of paramount importance’
“The main reasons for these results might be the data structure, its features — categorical and continuous variables,” Gupta and Qamar told Healio. “Moreover, [Extra Tree] model consistently performed better in terms of sensitivity and AUC. Even though other machine learning models had a good accuracy, it is the sensitivity of the model which is of paramount importance that needs to be considered for risk stratification in low- and middle-income countries. Thus, we finalized the Extra Tree Classifier as the MERC model for risk prediction following STEMI.
“Though there are some concerns with the use of AI, especially in terms of ethical issues with the data and the thought of being greatly dependent on machines than the human brains, our message is quite clear that AI is not going to replace the role of a physician, but on the contrary, it’s going to enhance his wisdom in making a wise decision,” they said.
Using a SHaP interpretable summary plot, the researchers determined that predictors of 30-day mortality included delay in time to revascularization, cardiogenic shock, left ventricular ejection fraction < 30%, age, serum creatinine, HF, female sex and moderate-to-severe mitral regurgitation (P < .001 for all).
“In resource-limited countries, triaging of the health care resources is of utmost importance,” Gupta and Qamar told Healio. “Resource utilization can only be proper when there has been adequate risk stratification prioritizing heath care resources to those who are at highest risk for adverse events. This would help in utilization of the limited available health care resources effectively and efficiently. For STEMI care, these resources would include ICU beds, prompt medical attention, regular and stringent follow-up and need for term monitoring devices. With the ever-increasing rate of STEMI in resource-limited countries such as India, health resource prioritization is all the more needed. This calls for risk stratification to identify high-risk patients needing priority resource allocation.”
For more information:
Mohit D. Gupta, MD, DM (Cardiology), FRCP (Edin), FACC, FSCAI, FESC, FCSI, FAPI, can be reached at drmohitgupta@yahoo.com.
Arman Qamar, MD, MPH, can be reached at aqamar@northshore.org; Twitter: @aqamarmd.