Machine learning may improve prediction of myocardial recovery on LVAD support
Machine learning models to predict myocardial recovery among patients who underwent left ventricular assist device implantation outperformed prior regression-based prognostic models, according to new data in Circulation: Heart Failure.
“Machine learning has emerged as a powerful tool for learning complex data patterns and identifying nonlinear interactions within large clinical data sets to formulate disease classifications as well as to predict patient outcomes,” Veli K. Topkara, MD, MSc, advanced heart failure and transplant cardiology specialist at Columbia University Irving Medical Center, and colleagues wrote. “Using the multicenter INTERMACS registry, we hypothesized that machine learning-based prediction models may improve selection of candidates who are likely to recover on LVAD support compared with traditional regression-based models and uncover novel clinical risk factors that are positively or negatively associated with myocardial recovery on LVAD support.”
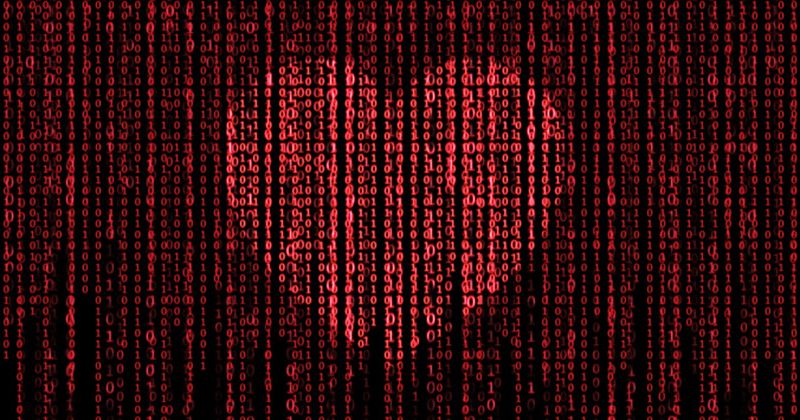
Training machine learning models
To this end, researchers trained the machine learning models to predict myocardial recovery following LVAD implantation using 28 unique clinical features. Half of these features were positively associated with myocardial recovery, including bridge-to-recovery implant strategy, current tobacco abuse, postpartum cardiomyopathy, recent cardiac diagnosis, history of alcohol use, limited social support, noncompliance, higher hemoglobin, sinus rhythm, elevated heart rate, elevated serum sodium, INTERMACS profile 3 and psychiatric disease. The other half were negatively associated with myocardial recovery, and included presence of an implantable cardioverter defibrillator, postimplant reduced ejection fraction, right ventricular assist device implantation with LVAD, old age, higher blood urea nitrogen, ischemic cardiomyopathy, use of centrifugal pump, higher postimplant LV end-diastolic diameter, bridging with intra-aortic balloon pump, preimplant warfarin use, preimplant amiodarone use, bridge-to-transplant indication and concomitant surgical tricuspid valve procedure.
Researchers included 20,270 adult patients from the INTERMACS registry with a durable continuous-flow LVAD.
Machine learning to predict myocardial recovery
According to the study, the machine learning models achieved area under the curve of 0.813 to 0.824 and outperformed the logistic regression-based new INTERMACS recovery risk score (AUC = 0.796), as well as the previously established LVAD recovery risk scores, INTERMACS Cardiac Recover Score (AUC = 0.744) and the INTERMACS Recover Score by Topkara and colleagues (AUC = 0.748; P for all < .05).
“Low positive predictive value of machine learning models is not unexpected given the low incidence of myocardial recovery in patients with LVAD,” the researchers wrote. “Inclusion of additional features that are not available in the INTERMACS, such as pharmacological therapy, doses of medications used, optimization of pump speed, and turn-down echocardiographic or hemodynamic indices, could improve discriminative ability of the models.”
Researchers observed a higher incidence of myocardial recovery resulting in LVAD explant among patients who were identified by the machine learning models to have myocardial recovery compared with patients not predicted to recover by the machine learning models (18.8% vs. 2.6%).
“Use and interpretation of complex machine learning models could be challenging for the clinician compared with simple logistic regression models. However, machine learning algorithms were shown to be superior to traditional risk models with regards to risk prediction of heart failure readmission and mortality,” the researchers wrote. “Similarly, current analysis suggested the superior discriminatory ability of machine learning models with regards to prediction of recovery in LVAD supported patients.”