Race-specific models, machine learning improves HF prediction vs. traditional risk scores
Race-specific machine learning models demonstrated greater prognostic value in the prediction of 10-year HF compared with more traditional, non-race-specific models, according to data published in Circulation.
Tailoring HF prediction models to patients based on their race also identified race-specific risk factors associated with HF, researchers reported in a Circulation issue with a theme of racial disparities.
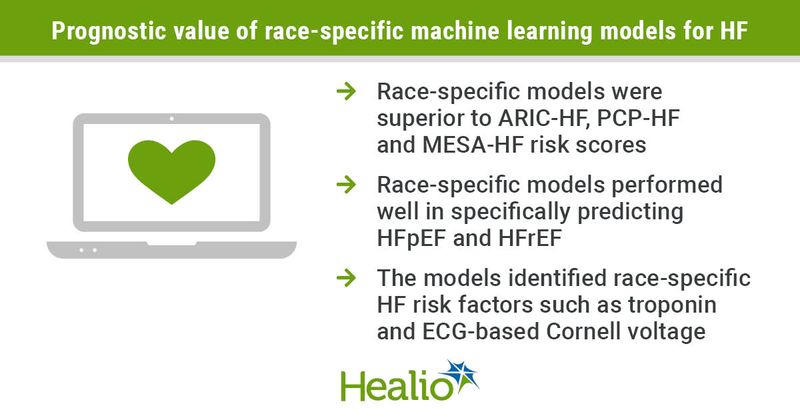
“Self-reported Black race is a heterogeneous entity and a surrogate marker for several factors, including but not limited to genetics, socioeconomic status, cultural elements, and often unmeasured societal experiences related to structured racism,” Matthew W. Segar, MD, MS, internist at University of Texas Southwestern Medical Center, and colleagues wrote. “The disproportionately higher risk of HF and other adverse outcomes in Black individuals is related to the downstream effects of all these components, which may not be adequately accounted for by adjusting for race in the risk models. Accordingly, there is a growing consensus to move away from race as a risk predictor approach. In the present study, we developed and validated HF risk prediction models in Black participants and white participants separately as a strategy to better elucidate the importance of risk factors that may be most relevant to development of HF in Black individuals.”
For this retrospective analysis of the Atherosclerosis Risk in Communities (ARIC) study, Dallas Heart Study (DHS), Jackson Heart Study (JHS) and Multi-Ethnic Study of Atherosclerosis (MESA), researchers evaluated participants older than 40 years who were free from HF at baseline using race-specific risk scoring.
Race-specific machine learning models for HF risk prediction in Black individuals were developed from the JHS cohort, and models for white adults were developed from the ARIC cohort, including 39 variables across demographic, anthropometric, medical history, laboratory and ECG domains.
Researchers externally validated the machine learning models among race-specific subgroups of the pooled MESA/DHS cohort and Black participants of the ARIC study. In addition, the race-specific machine learning models were compared with prior established traditional and non-race-specific machine learning models, including the ARIC-HF, Pooled Cohort Equation to Prevent Heart Failure (PCP-HF) and MESA-HF risk scores.
HF prediction with race-specific models
Among Black adults, researchers observed a high overall C-index for the machine learning model for the prediction of HF in both external validation cohorts (ARIC, 0.8; 95% CI, 0.75-0.84; MESA/DHS, 0.83; 95% CI, 0.77-0.87).
According to the study, the machine learning model was superior to the other traditional and non-race-specific machine learning risk scores, including those that were used to derive these very models.
In the external validation of the race-specific machine learning model among white participants in the DHS/MESA pooled cohort, the race-specific risk score demonstrated the highest C-index for prediction of HF risk (0.82; 95% CI, 0.78-0.86), compared with the non-race-specific risk scores. The race-specific model detected as many as six more HF events per 1,000 patients compared with the ARIC-HF, PCP-HF and MESA-HF risk scores.
Researchers also evaluated the race-specific models when used in race-discordant cohorts. The C-index of race-specific models was higher in race-concordant compared with race-discordant cohorts, and validation in race-discordant cohorts resulted in miscalibration (Greenwood-Nam-D’Agostino P < .05).
The high predictive value of race-specific models was consistent in both men and women, across both races in the validation cohorts.
Among both Black and white participants, the race-specific models performed well in predicting risk for HF with reduced ejection fraction and HF with preserved ejection fraction in the pooled MESA/DHS validation cohort.
Among risk factors included in the race-specific model, natriuretic peptide level was the strongest predictor of HF risk among both Black and white patients, followed by troponin levels among Black participants and ECG-based Cornell voltage among white individuals.
‘The next steps’ to integrating machine learning
“A key next step for the practical implementation of the machine learning-based race-specific model in the clinical setting is its integration with electronic health records,” the researchers wrote. “This will not only improve accessibility and real-world application of these machine learning-based models but also allow better and automated capture of data on the risk prediction variables,” the researchers wrote. “To this end, continued improvements in electronic health record software will allow machine learning models to be developed and stored on a secure cloud server and implemented in the electronic health record through the use of an application programming interface.
“The next steps to facilitate integration of the machine learning-based race-specific models with the electronic health record include storing the models on a secure cloud-computing server, developing an application programming interface to access our race-specific models, and scaling the platform to provide real-time estimates of a patient’s HF risk,” the researchers wrote.