AI-based echo analyses best human analyses for COVID-19 mortality prediction
In patients with COVID-19 who had an echocardiogram, analysis by artificial intelligence better predicted mortality than analysis by experts, according to the results of the WASE-COVID study.
For the study, presented at the American College of Cardiology Scientific Session, researchers enrolled 870 patients hospitalized with COVID-19 (mean age, 59 years; 44% women) who underwent transthoracic echocardiography.
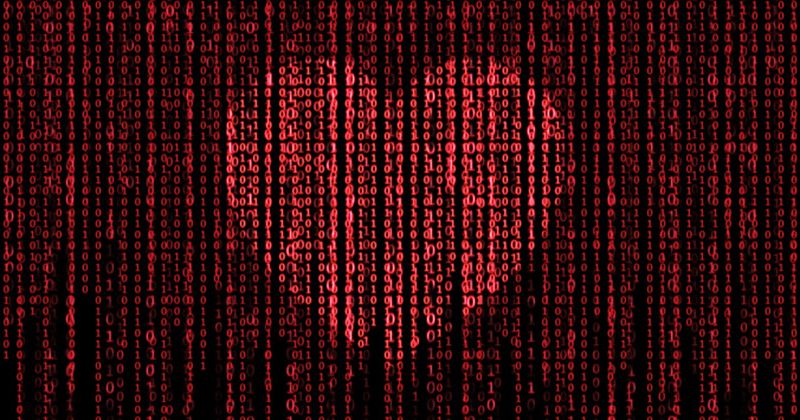

In phase 1 of the study, to determine predictors of in-hospital mortality, the rate of in-hospital all-cause mortality was 21.6%, Federico M. Asch, MD, FASE, FACC, director of the Echocardiography Core Lab at MedStar Health Research Institute in Washington, D.C., and associate professor of medicine at Georgetown University, said during a presentation. Phase 1 was simultaneously published in the Journal of the American Society of Echocardiography.
“Myocardial injury has been linked with poor outcomes; therefore, an echocardiogram at admission may be a powerful tool to predict death,” Asch said.
In a multivariate analysis, left ventricular longitudinal strain was associated with in-hospital mortality (HR = 1.179; 95% CI, 1.045-1.358; P = .012), as were age, lactate dehydrogenase level, right ventricular free wall strain and previous lung disease, but LV ejection fraction was not, Asch said.
Phase 2 of the study, to compare AI software-based echocardiography analysis (Ultromics) with manual expert analysis, included echocardiograms from 476 patients for whom echocardiograms could be analyzed by both AI and manual expert methods. All-cause mortality through a mean follow-up of 230 days was 27.4%, Asch said.
“With reader-dependent technologies such as echo, fully automated, AI-based analysis should result in lower variability of results than those obtained from human reads,” Asch said during the presentation. “With increased interpretation consistency, it is foreseeable that the use of automated measurements could improve the capacity to predict outcomes.”
The experts and the software predicted mortality based on LV longitudinal strain and LVEF.
Variability was significantly larger for manual expert analysis than for AI analysis. Analysis by different operators was the main source of variance in the expert analyses (47.39% of variance for EF, 51.81% for longitudinal strain). AI software analysis had minimal variance, which was mostly due to selection of different video frames (6.3% variance for EF; 5.96% for longitudinal strain), Asch said.
The software outperformed the experts for prediction of in-hospital mortality (EF manual, OR = 0.985; 95% CI, 0.969-1.003; P = .083; EF software, OR = 0.97; 95% CI, 0.952-0.988; P = .001; longitudinal strain manual, OR = 1.035; 95% CI, 0.999-1.074; P = .058; longitudinal strain software, OR = 1.082; 95% CI, 1.035-1.132; P < .001) and follow-up mortality (EF manual, OR = 0.99; 95% CI, 0.975-1.005; P = .187; EF software, OR = 0.974; 95% CI, 0.956-0.991; P = .003; longitudinal strain manual, OR = 1.024; 95% CI, 0.991-1.059; P = .155; longitudinal strain software, OR = 1.06; 95% CI, 1.019-1.105; P = .004), according to the researchers.
“Automated quantification of left ventricular ejection fraction and left ventricular global longitudinal strain using artificial intelligence minimized variability,” Asch said. “AI-based LVEF and longitudinal strain analyses, but not manual, were significant predictors of in-hospital and follow-up mortality. AI analyses of echo could increase statistical power to predict outcomes, possibly requiring smaller sample sizes in clinical trials.”