Artificial intelligence may improve risk assessment for TAVR
A semi-supervised automated machine learning algorithm improved predictions of adverse events after transcatheter aortic valve replacement in patients with severe aortic stenosis compared with the Society of Thoracic Surgeons risk score.
“What we showed is that this machine learning model can be better than the old statistical models, and it can allow you to classify your patients into higher-risk phenotypes,” Partho P. Sengupta, MD, chief of cardiology and Abnash C. Jain Chair and Professor of Cardiology at West Virginia University School of Medicine in Morgantown and senior author for the study, told Healio.
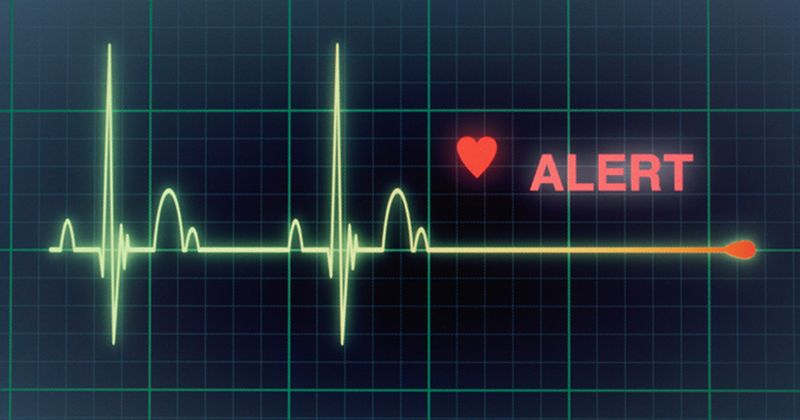
Single-center assessment of TAVR
In the study published in The American Journal of Cardiology, Yasir Abdul Ghaffar, MD, cardiology fellow at West Virginia University School of Medicine who worked under Sengupta’s mentorship for the project, and colleagues analyzed data from 344 patients from the STS/American College of Cardiology Transcatheter Valve Therapy registry who underwent TAVR at West Virginia University Hospital from April 2014 to May 2019.
Patients were divided into two cohorts to account for improvement in procedural techniques and devices over time, in addition to changing risk profile. These cohorts included patients who underwent TAVR from April 2014 to June 2018 (n = 211; mean age, 80 years; 45% men) and from July 2018 to May 2019 (n = 143; mean age, 79 years; 50% men).
Clinical features from the first cohort were used to identify unique patient groups with aortic stenosis. Patients in the second cohort were identified using a supervised machine learning classifier that produced phenogroup labels for new patients using information on clinical features. To validate the phenogroup, researchers compared clinical features between the groups, then compared the phenogroups for their link to TAVR outcomes.
“There are so many different ways you can do machine learning models,” Sengupta said in an interview. “It’s the common buzzword, but we showed a different model here where it learns from the data itself and creates those high-risk phenogroups. After the machine learning shows us the high phenogroups, then we train it further on to be able to detect those phenogroups. It’s almost an autonomous way to find the risk groups and then basically be able to train the model to be able to detect it.”
Clinical endpoints included CV mortality and all-cause mortality, both of which were assessed in-hospital and within 30 days.
Researchers defined five patient phenogroups:
- group 1 (n = 38): classic high gradient, normal left ventricular ejection fraction, severe aortic stenosis and a median intermediate STS risk score of 4.7 (95% CI, 3.55-5.44; P < .001);
- group 2 (n = 41): class high gradient and low gradient, normal LVEF, severe aortic stenosis and a median intermediate STS risk score of 6 (95% CI, 3.6-7.4);
- group 3 (n = 61): similar echocardiographic parameters as group 2;
- group 4 (n = 41): high and low gradients, normal and reduced LVEF, severe aortic stenosis and a median intermediate STS risk score of 8.1 (95% CI, 5.2-11.33; P < .003); and
- group 5 (n = 30): high number of implantable cardioverter defibrillators and pacemakers, HF exacerbations before TAVR and pre-TAVR cardiac procedures.
When both cohorts were combined, group 1 had the lowest rates of procedural complications after TAVR (OR = 0.25; 95% CI, 0.09-0.7). In contrast, group 5 had higher rates of in-hospital all-cause mortality (OR = 9; 95% CI, 2-33), in-hospital CV mortality (OR = 35; 95% CI, 4-309), 30-day all-cause mortality (OR = 3; 95% CI, 1.2-9) and 30-day CV mortality (OR = 18; 95% CI, 3-94).
Predicting 30-day CV mortality with both the phenogroups and the STS score improved overall prediction compared with STS scores alone (area under the curve = 0.96 vs. 0.8; P = .02).
Future research
“What we need is an independent further validation in bigger data sets, national registries and different geographical locations to get successfully translated into clinical practice, but it’s going to happen,” Sengupta told Healio. “These kinds of models and these kinds of tools will definitely come into play.”
For more information:
Partho P. Sengupta, MD, can be reached at partho.sengupta@hsc.wvu.edu.