AI algorithm for ECGs may identify LV systolic dysfunction
ECGs analyzed by AI identified left ventricular systolic dysfunction in patients who presented to the ED with dyspnea, researchers found.
“An artificial intelligence-enabled ECG can detect left ventricular systolic function quicker and more accurately than NT-proBNP among patients with shortness of breath,” Demilade Adedinsewo, MD, MPH, chief fellow in the division of cardiovascular medicine at Mayo Clinic in Jacksonville, Florida, told Healio. “It can potentially lead to improved and quicker diagnosis in the emergency department and provides a unique opportunity to identify high-risk cardiac patients earlier and link them to appropriate cardiovascular care.”
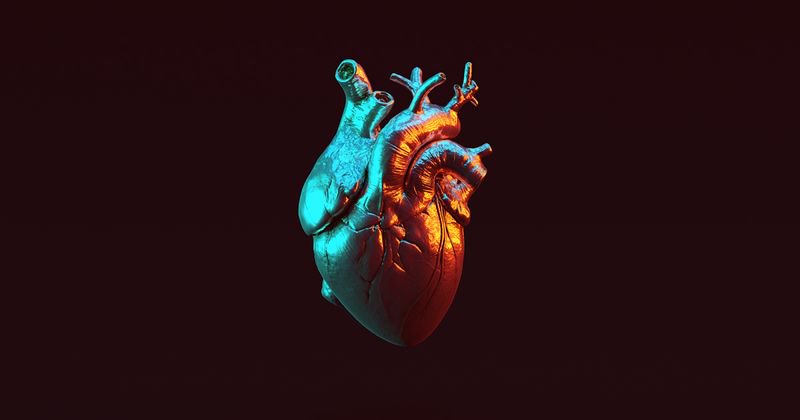
Patients with dyspnea
In this retrospective study published in Circulation: Arrhythmia and Electrophysiology, researchers analyzed data from 1,606 patients (median age, 68 years; 47% women) who presented to the ED with dyspnea between May 2018 and February 2019. These patients had at least one ECG performed within 24 hours and within 30 days of the ED visit. Those with a prior diagnosis of systolic, diastolic or unspecified HF were excluded.
The primary outcome for this study was the identification of patients with new LV systolic dysfunction, defined as an LV ejection fraction of 35% or lower, within 30 days of the ED visit. The secondary outcome was defined as the identification of patients with an LVEF of less than 50% within 30 days of the visit. Both outcomes were determined by ECGs assessed by a deep learning network, which was an AI-ECG algorithm that was developed and validated to identify LVEF of 35% or lower without additional optimization or training.
The median time to an ECG after the ED visit was 1 day.

The AI-ECG algorithm to identify new LV systolic dysfunction in patients who presented to the ED with dyspnea had an area under the receiver operating characteristic curve of 0.89 (95% CI, 0.86-0.91). This algorithm had 85.9% accuracy (95% CI, 84.1-87.6), in addition to 87% specificity, 74% sensitivity, 40% positive predictive value and 97% negative predictive value.
The algorithm was also able to identify patients with an LVEF less than 50% with an area under the receiver operating characteristic curve of 0.85 (95% CI, 0.83-0.88) and 86% accuracy (95% CI, 84.2-87.7). This also achieved 91% specificity, 63% sensitivity, 62% positive predictive value and 92% negative predictive value.
Researchers also assessed a subgroup of 866 patients with available N-terminal pro-B-type natriuretic peptide values. NT-proBNP levels greater than 800 pg/mL identified patients with new LV systolic dysfunction with an area under the receiver operating characteristic curve of 0.8 (95% CI, 0.76-0.84).
“The current study is retrospective, and prospective studies are needed to evaluate the effect of the AI-ECG on long-term clinical outcomes,” Adedinsewo said in an interview. “Our research team is currently in the process of evaluating this.”
Adedinsewo added that this technology is currently being used throughout her health care system. “This AI-ECG tool is currently available at all Mayo Clinic sites and can be accessed through our electronic medical record system,” she told Healio. “In addition, this tool recently received emergency use authorization through the FDA in May to screen for left ventricular dysfunction in patients with confirmed or suspected COVID-19.”
‘Potential to advance patient care’
In a related editorial, Kazi T. Haq, PhD, of the Knight Cardiovascular Institute at Oregon Health and Science University in Portland, Oregon, and colleagues wrote, “Overall, the findings by Adedinsewo, et al. show that AI using the standard 12-lead ECG can improve the identification of new heart failure among patients in the emergency department with dyspnea. This is a strategy that can be easily and readily employed in clinical practice and has the potential to advance patient care significantly.”
Reference:
For more information:
Demilade Adedinsewo MD, MPH, can be reached at adedinsewo.demilade@mayo.edu.