Smart devices may detect cardiac arrest
A proof-of-concept system with smart devices such as an Amazon Echo and Apple iPhone was able to effectively identify agonal breathing associated with cardiac arrest, according to a study published in NPJ Digital Medicine.
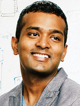
“Prior research has shown that the presence of agonal breathing can significantly increase the chance of survival once you get CPR in the case of cardiac arrest,” Shyamnath Gollakota, PhD, MS, associate professor at the Paul G. Allen School of Computer Science and Engineering at the University of Washington in Seattle, told Cardiology Today. “We show that a smart speaker or a smartphone can listen to sounds and identify agonal breathing associated with cardiac arrests with a greater than 97% accuracy and in real time.”
Emergency calls
Justin Chan, doctoral student at the Paul G. Allen School of Computer Science and Engineering at the University of Washington, and colleagues analyzed data from 729 emergency calls to 911 for a known cardiac arrest and instances of agonal breathing between 2009 and 2017. There were 82 hours of audio from these phone calls, which were mostly recorded from an iPhone 5S, Amazon Alexa and a Samsung Galaxy S4.
“The technology itself can work on both smartphones and speakers,” Gollakota said in an interview. “However, smart speakers are a lot more attractive for this application since they are already listening and are plugged in next to your bedside, so you are not worried about power consumption. Further, most people who work on mobile health are focused on smartphones. This project is a very good example of how smart speakers can also be used for detection of medical conditions.”
In addition, there was 83 hours of negative data from 12 patients who were recorded on a phone in a sleep lab.
Both sets of recordings were played at distances of 1 m, 3 m and 6 m with interference from indoor and outdoor sounds.
The area under the curve was 0.9993, with an overall specificity of 99.51% (95% CI, 99.35-99.67) and sensitivity of 97.24% (95% CI, 96.86-97.61).
The false positive rate was between 0% and 0.14% for over 82 hours of polysomnographic sleep lab data that included hypopnea, snoring, obstructive sleep apnea events and central sleep apnea events.
When assessing the system in home sleep environments, the false positive rate was between 0% and 0.22%.
Further research
“Right now, the algorithm has been trained on agonal breathing sounds from 911 calls in the Seattle area from 2009 to 2017,” Gollakota told Cardiology Today. “Getting more 911 call data across the country will help generalize the performance.” – by Darlene Dobkowski
For more information:
Shyamnath Gollakota, PhD, MS, can be reached at University of Washington, Box 352350, Seattle, WA 98195; email: gshyam@cs.washington.edu.
Disclosures: All authors are inventors on a U.S. provisional patent that was submitted by the University of Washington. Chan reports he has equity stakes in Edus Health unrelated to the technology in this study. Gollakota reports he is a co-founder of Jeeva Wireless and Sound Life Sciences. Please see the study for all other authors’ relevant financial disclosures.