Machine-learning algorithm improves iFR measurement quality
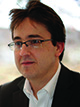
SAN DIEGO — In determining appropriateness of PCI and PCI strategy, a machine-learning algorithm was noninferior to an expert heart team, according to the results of the CEREBRIA-1 study presented at TCT 2018.
The goal was to determine whether computational interpretation of pressure-wire pullback data was similar to or improved upon expert human interpretation for interventional strategies in patients with stable CAD who underwent instantaneous wave-free ratio measurement (Philips/Volcano), Justin Davies, MBBS, PhD, from Hammersmith Hospital, Imperial College NHS Trust, London, said during a presentation.
Davies and colleagues had 1,008 iFR pullback traces, 691 of which were unique, from 640 patients (mean age, 65 years; 74% men) analyzed by computational analysis and by 15 experts from five countries, for a total of 30,240 expert interpretations.
Davies said the computer was trained by physiology experts using a priming dataset to distinguish significant stenoses appropriate for PCI from nonsignificant ones. To prevent bias, the priming dataset was separate from the study dataset and none of the trainers served as human experts for the study dataset.
Among the 691 unique iFR pullback traces, median distal iFR value was 0.87 (interquartile range [IQR], 0.81-0.91) and median proximal iFR value was 0.99 (IQR, 0.98-1.01). Among the vessels analyzed, 79.5% were left anterior descending arteries. Distal iFR was 0.89 or less in 68% of vessels; Davies said that threshold indicated hemodynamic significance.
The 317 duplicate iFR pullback traces were included as an experiment to see whether the human experts would make different decisions on the same case; approximately one of 10 times, they did, Davies said. “On the other hand, a computer never changes its mind or strategy,” he said.
Compared with the median interpretation of the expert humans, computational interpretation was noninferior for determining the appropriateness of PCI in terms of whether it should be performed (expert humans, 89.3%; machine, 89.4%; P for noninferiority = .0073) and PCI strategy in terms of where the stent should be placed (expert humans, 88.8%; machine, 89.7%; P for noninferiority < .001), Davies said.
“Computer analysis can give a decision on the appropriateness of pressure wire pullback curve at least as good as expert consensus,” Davies said.
In most cases, the expert humans and the computer agreed whether PCI should be performed and where the stent should be placed, he said, but 3.8% of cases determined by humans to be significant for PCI were negative when accounting for wire drift, and 27% of cases determined to be nonsignificant for PCI were actually positive.
“When you take into account the fact that lesions were missed in regard to drift and they were missed according to how significant they were, this accounts for the discordance of the algorithm not being in 100% agreement” with humans, Davies said during the presentation. “Computers are very good at watching all the time. They never miss these little things. I think they can help us make sure that in a busy lab, we don’t miss things. The computer never misses drift or significant stenoses. Computer-intelligent algorithms can alert to dampening or artifact to help improve patient safety and quality of measurements. What we’ve demonstrated here is it’s possible to avoid measurement errors such as drift and dampening every time, and essentially bring expert analysis into the cath lab for every single case, independent of how experienced that individual operator is.” – by Erik Swain
Reference:
Davies JE, et al. Keynote Interventional Studies IX: Four Fascinating and Very Different Studies. Presented at: TCT Scientific Symposium; Sept. 21-25, 2018; San Diego.
Disclosure: Davies reports he receives grant/research support, consultant fees/honoraria, royalty income and intellectual property rights from Philips/Volcano.