Deep neural network detects AF using smartwatch data
The measurement of blood volume changes and a deep neural network on smartwatches were used to detect atrial fibrillation in patients, according to a study published in JAMA Cardiology.
“Smartwatches are well-positioned to accomplish these goals in a cost-efficient and resource-efficient fashion,” Geoffrey H. Tison, MD, MPH, cardiologist and assistant professor in the division of cardiology at the University of California, San Francisco, and colleagues wrote. “Wearable technology has shown a steady increase in global usage, and the smartwatch, most popular among all wearable sensors, is projected to reach 55 million global shipments by 2020.”
Deep neural network
Researchers analyzed data from three cohorts when developing a deep neural network for the detection of AF through a smartwatch. Information such as heart rate and step count from a mobile application (Cardiogram) for a commercially available smartwatch (Apple) was collected for the deep neural network.
Network development and training were performed on the remote cohort from the Health eHeart study (n = 9,750; mean age, 42 years; 63% men). The network was trained on more than 139 million heart rate measurements.
The network was then evaluated and validated in another cohort of participants with AF who were undergoing electrical or pharmacologic cardioversion (n = 51; mean age, 66 years; 84% men). Exclusion criteria for this cohort included those with atrial arrhythmias other than AF, ventricular-assist devices and ventricular pacing. Participants wore the watch for at least 20 minutes in workout mode and an ECG was obtained.
Exploratory analysis was performed with data from a subset of participants from the remote cohort to detect AF from ambulatory data.
AF was observed in 347 patients in the remote cohort (mean age, 56 years; 76% men).
Sensitivity of smartwatches
Among participants who were undergoing cardioversion, the deep neural network had a C-statistic of 0.97 (95% CI, 0.94-1). Specificity to detect AF against a reference of a 12-lead ECG was 90.2% and sensitivity was 98%.
In an exploratory analysis of an ambulatory subcohort of 1,617 participants (4% with self-reported AF), the technology detected persistent AF with a C-statistic of 0.72 (95% CI, 0.64-0.78), a sensitivity of 67.7% and a specificity of 67.6%.
“Given the broad and growing use of smartwatches and ready accessibility of downloadable mobile applications, this approach may ultimately be applied to perform AF detection at large scale, ultimately leveraging common wearable devices to guide AF management and rhythm assessment,” Tison and colleagues wrote.
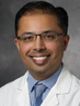
“Deep learning and artificial intelligence more broadly will be extended beyond arrhythmia detection in cardiology quite rapidly,” Mintu P. Turakhia, MD, MAS, associate professor of cardiovascular medicine and director of research at the Center for Digital Health at Stanford University in California, director of cardiac electrophysiology at the VA Palo Alto Health Care System and a Cardiology Today Next Gen Innovator, wrote in a related editorial. “As a field, we need to think creatively but prudently about finding optimal use cases for these technologies... There will remain extraordinary opportunities as the interface of design, scientific evaluation and implementation.” – by Darlene Dobkowski
Disclosures: The study was funded by Cardiogram. Tison reports he is an adviser for Cardiogram. Please see the study for all other authors’ relevant financial disclosures. Turakhia reports he received research support from the American Heart Association, Apple, AstraZeneca, Boehringer Ingelheim, Cardiva Medical, Janssen and Medtronic; received personal fees from Abbott, Cardiva, iBeat, Medscape, Medtronic and Precision Health Economics; and holds equity in AliveCor, iBeat, CyberHeart, Forward and Zipline Medical.