Model demonstrates ability to differentiate active corneal ulcers, healed scars
A convolutional neural network was able to identify corneal ulcers and scars with high accuracy even among a patient population outside of its training data, according to a study.
“Using photographs of corneal ulcers from the SCUT and MUTT studies, we developed a machine learning algorithm that classifies corneal ulcers and scars with high accuracy,” Charles C. Lin, MD, told Healio/OSN.
Lin and colleagues trained a convolutional neural network to classify active corneal ulcers and scars using 1,313 photos of corneal ulcers and 1,132 photos of corneal scars sourced from the Steroids for Corneal Ulcers Trial (SCUT) and the Mycotic Ulcer Treatment Trial (MUTT).
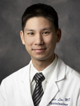
Following training, the model classified 115 of 123 active corneal ulcers and 65 of 77 corneal ulcer scars for a sensitivity of 93.5% and a specificity of 84.42%. This first test set was comprised of Indian patients from the SCUT and MUTT, but the images had not been seen by the model before.
In the second test set, comprised from a patient population in Northern California, the model correctly classified 43 of 55 active corneal ulcers and 42 of 46 corneal scars, with a sensitivity of 78.2% and a specificity of 91.3%.
“The generalizability of our model indicates that the algorithm identifies salient features of corneal ulcers and scars that are present despite epidemiological differences,” the authors wrote.
Potential limitations to the study include the slight decrease in generalization performance in patients with different geographic and epidemiological backgrounds. Additionally, because the study images were obtained with high-resolution digital cameras, the model may not be as effective with the use of lower-quality images. Possible solutions include training the model specifically for different populations and using standardization guidelines for image quality.
“This convolutional neural network also generalizes well to patient populations outside of its training data, demonstrating potential as an inexpensive diagnostic approach in areas with limited access to trained eye care specialists,” Lin said.