IOL calculations evolve with artificial intelligence
An experienced cataract surgeon has developed an intuition after having done thousands of cases over the course of many years. If you ask this surgeon about IOL power selection, you will hear many pearls that we know ring true: use the Holladay 2 for very short axial lengths, for longer axial lengths look at the SRK/T formula and choose a higher power to avoid residual hyperopia, if the keratometry values are unusual then avoid the SRK/T and choose the lowest keratometry power in the central zone, the Hoffer Q formula tends to work better in shorter eyes, the Haigis formula is useful for unusual anterior chamber depths, and more. But how can we convert this intuition into an approach that is available and useful to even the novice surgeon?
The first step is to realize that the approach of using one formula for all eyes has significant limitations. The initial generation of theoretical formulas, such as the SRK/T, Hoffer Q, Holladay 1 and Haigis, has been published and is available for analysis. These formulas consist of one equation for all eyes. Some of the more recent developments, such as the Holladay 2, the Barrett Universal, the Hill-RBF and the Ladas Super Formula, are probably better classified as methodologies because they are more than just single equations. Each of these methods has an approach that is adjusted based on the input parameters of the eye. For example, a different calculation is done for a longer axial length than a shorter one. This allows these methods to be appropriate for a wide range of eyes.
Going beyond the single equation
If you want a method for IOL calculation that is appropriate for most eyes, the Barrett Universal is a great starting point. When we plot thousands of simulated eyes calculated with this method, we see break points at axial lengths of about 22.5 mm, 26 mm and 28.5 mm (Figure 1, left graph). While most surgeons are familiar with the two-dimensional plot of IOL power vs. axial length, there is more information to be gleaned when we include the corneal power and plot it in three dimensions using the graphing technique originally described by John Ladas, MD, PhD (Figure 1, right graph).
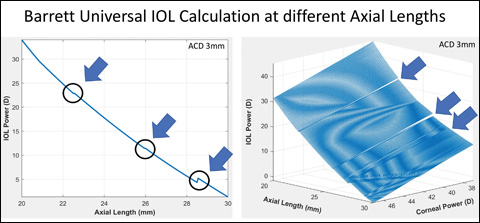
Source: Uday Devgan, MD
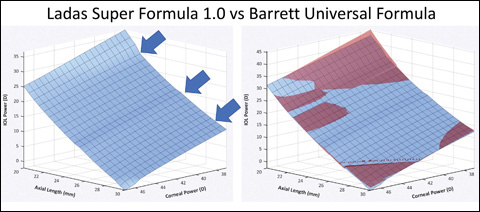
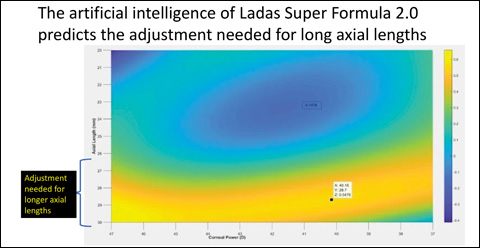
The Ladas Super Formula 1.0, which was described first in 2015, took a similar approach, dividing the eyes based initially on axial length (Figure 2, left graph). When we superimpose the plots of the Ladas and Barrett calculations over a range of axial lengths and corneal powers, we can see that they overlap widely with only small differences for extreme eyes (Figure 2, right graph). But these two methods will need to be refined as we learn more such as the effect of the posterior corneal power and perhaps other variables such as gender or genetic background, which have been postulated to influence the effective lens position determination. In order to see patterns across thousands of eyes and to evolve, we need a big-data approach. Warren Hill, MD, brought tremendous insight to this approach with his Hill-RBF idea, in which the RBF is a radial basis function that can be interpreted as a kind of neural network artificial intelligence (AI).
The artificial intelligence approach
We have seen how AI has challenged the most brilliant minds in chess, and now the best grandmaster in history is a computer. The same approach can be applied to IOL power determination: We give input variables such as axial length, keratometry, anterior chamber depth and more that are then weighed appropriately with the hidden inner layers of the AI, and the IOL power is predicted, consistent with the given data set. A potential downfall of relying solely on AI is the risk of an unreliable or out-of-bounds result due to a sparsity of data for certain types of eyes.
The Ladas Super Formula 2.0 incorporates AI and eliminates the risk for an out-of-bounds result by using the original Ladas Super Formula 1.0 as a framework. Also, additional variables can be learned instantly and appropriate adjustments can be made as they relate to the existing variables. Euclid, the father of geometry, put forth a simple yet enduring mathematical concept, that things are best described as their displacement from the origin. The original Ladas Super Formula is defined as the origin, and the AI and big data approach are the displacement from a perfect outcome.
Instead of outright predicting IOL power, such as with the Hill-RBF, Ladas Super Formula 2.0 uses the big data approach to predict the difference or displacement between an existing formula and perfection for a given eye. These adjustments are not random and typically occur on the order of 0.2 D. These predicted displacements or adjustments are seamlessly incorporated back into the existing formula. Because the baseline is an already proven formula, differences are 100 times smaller in magnitude than the standard AI approach that is instead predicting IOL power (0.2 D vs. 20 D). There is no human that could ever write a single formula that takes into account every adjustment or intuition previously described, but this form of high-resolution AI can accomplish this.
An example that clearly illustrates the power of the Ladas Super Formula 2.0 is the AI prediction that eyes with longer axial lengths will need to be adjusted to improve refractive accuracy. We know from the Wang-Koch axial length adjustment that a long eye certainly does need an adjustment made to the axial length before using the traditional IOL calculation equations. Using a massive amount of computing power, a significant displacement from the origin for IOL power was predicted for axial lengths of about 26 mm or longer, varying somewhat with the keratometry as well (Figure 3).
Multiple more refinements are being done using this big-data approach with the Ladas Super Formula backbone. The result is more accurate postoperative refractive results for our patients and greater confidence in our ability to deliver consistently excellent outcomes. With further evolution, AI may prove to be the grandmaster of both IOL calculations and chess.
- For more information:
- Uday Devgan, MD, is in private practice at Devgan Eye Surgery, Chief of Ophthalmology at Olive View UCLA Medical Center and Clinical Professor of Ophthalmology at the Jules Stein Eye Institute, UCLA School of Medicine. He can be reached at 11600 Wilshire Blvd. #200, Los Angeles, CA 90025; email: devgan@gmail.com; website: www.DevganEye.com.
Disclosure: Devgan reports he is a principal in Advanced Euclidean Solutions, which owns the Ladas Super Formula, which can be accessed at www.IOLcalc.com for free.