AI applications in ophthalmology achieve human expert-level performance
Artificial intelligence, deep learning and machine learning are being used by ophthalmologists to verify disease diagnoses, read images, perfect IOL calculations and improve surgical outcomes as these advanced techniques become more commonplace in the field.
Artificial intelligence, also called AI, spans a broad field. Machine learning is a subfield of AI, and deep learning is a subfield of machine learning, Aaron Y. Lee, MD, of UW Medicine, said. Most recent breakthroughs have been in the field of deep learning.
“These breakthroughs in computer vision have allowed near human performance on many tasks in the last 4 or 5 years,” Lee said.
For example, deep learning has been used to develop methods for performing automated diagnoses.
“Recently the FDA approved using machine learning algorithms for the purpose of automated diabetic retinopathy grading. This is very exciting as it has potential to deliver care to a wider number of people or in resource-limited settings. I suspect that these algorithms will play a disruptive role in the delivery of health care as well as the way we practice ophthalmology,” Lee said.

Source: SLACK Incorporated
In April the FDA approved IDx-DR (IDx), a diagnostic system for the autonomous detection of diabetic retinopathy. IDx-DR is the first autonomous, AI-based diagnostic system authorized for commercialization by the FDA, according to a company press release.
Surpassing human performance
Many published papers show that deep learning models can achieve human expert performance, and in many cases in which an objective ground truth is available, the models can even surpass expert human performance, Lee said.
“In my opinion, the most exciting areas of AI applications in ophthalmology are in the areas of personalized medicine and future prognosis. Compared to the traditional statistical models used for risk prediction, deep learning models are much more flexible and powerful. For example, deep learning models may have the potential to read a [Humphrey visual field] and predict how quickly they will go blind or read an OCT and predict who will develop wet macular degeneration,” he said.
It is possible to train a neural network to be on par with the performance of retinal specialists for the grading of diabetic retinopathy, Lily Peng, MD, PhD, a product manager at Google, said.
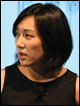
Peng and colleagues used deep learning techniques to develop an algorithm to read and grade retinal fundus imaging for diabetic retinopathy. Peng used adjudication of three retinal specialists as the reference standard and published the results of the study in Ophthalmology.
Reading retinal images
Automated systems generally have little intragrader variability, so the implication of the work is that if they are implemented well, there could be less variability in the screening process, Peng said.
“We used ophthalmologists and retinal specialists to provide grades for the images. The ground truth was the adjudicated grade from three retinal specialists. Although adjudication yields a more reliable ground truth, it requires significant time and resources to perform. We demonstrate that by using existing grades and adjudicating a small subset (0.22%) of the training image grades for the ‘tune’ set, we were able to significantly improve model performance without adjudicating the entire training corpus of images. Leveraging these techniques, our resulting model’s performance was approximately on par with that of individual ophthalmologists and retinal specialists,” she said.
The automated algorithm and trained ophthalmologists graded retinal fundus images from diabetic retinopathy screening programs. According to the results of the study, for moderate or worse diabetic retinopathy, the majority decision of the ophthalmologists had a sensitivity of 0.838 and a specificity of 0.981 compared with a sensitivity of 0.971 and a specificity of 0.923 for the algorithm. For mild or worse diabetic retinopathy, the algorithm had a sensitivity of 0.970 and a specificity of 0.917.
Unlike other machine learning techniques, which rely on “feature engineering” in which computers are programmed to follow a set of explicit rules, deep learning involves programming the computer to learn from many labeled examples without explicitly defining which features are important, Peng said.
“Thus, selection of the right reference standard is critical in building clinically relevant deep learning algorithms. In addition, because obtaining the best reference standard can be resource-intensive, we demonstrate how only a subset of the images (for example, the ‘tune’) have to be labeled in a resource-intensive manner and yet yield superior results,” she said.
Machine learning has the potential to increase availability and accuracy of care, Peng said, especially in ophthalmology, in which there are shortages of trained eye care professionals.
Developments in telemedicine
There is currently a lack of manpower in the field of ophthalmology to screen national and international patients, according to Lama A. Al-Aswad, MD, MPH, of the Edward S. Harkness Eye Institute of Columbia University Medical Center. AI may be able to help make up for that lack of manpower and screen patient images autonomously.
“Telemedicine in ophthalmology will help in disease detection, access to care and blindness prevention. Currently we do not have the manpower to screen individuals nationally and internationally for the four leading causes of blindness — cataract, diabetic retinopathy, glaucoma and macular degeneration — not to forget about refractive error. AI can help us in screening for disease by having a high negative predictive value. In other words, the ability for the AI to predict normal or what is called normalcy. AI is able to help us by identifying the normal individuals and flagging the abnormal to refer to eye care specialists even if we had high false positive,” she said.
The FDA approval of IDx-DR is an exciting development as well, Al-Aswad said. The approval opens the door for other systems to be developed and approved for the detection of glaucoma, age-related macular degeneration, retinopathy of prematurity and ocular oncology, she said.
“There are currently a few companies working on AI for diabetic retinopathy, glaucoma and macular degeneration. In addition, AI is currently used in helping with low vision such as the smart glasses by Microsoft and other companies and few apps that can be downloaded to your smartphone such as TapTapSee to help maneuver the surroundings of visually impaired individuals,” Al-Aswad said.
Improving refractive surgery formulas
Artificial intelligence is also being used to improve formulas and advance accuracy of refractive surgery, Healio.com/OSN Section Editor Uday Devgan, MD, said.
AI can analyze “tremendous amounts of information or data” and see trends or patterns that ophthalmologists would not be able to see themselves, he said.
Experienced cataract surgeons have developed an intuition after doing thousands of cases and have a sense of what IOL calculation will offer patients the best outcomes, Devgan said.
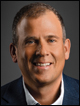
“So, how can we incorporate that into our calculations and go from there to improve things?” Devgan asked.
Using just one formula for every eye has limitations. The Barrett Universal formula may be appropriate for most eyes, but Devgan and John G. Ladas, MD, PhD, devised their own formula in 2015 to improve accuracy.
The Ladas Super Formula 1.0 incorporated ideal portions of existing IOL formulas to maximize their strengths and reduce their weaknesses. According to a 2015 study in JAMA Ophthalmology, the super formula calculated the most accurate IOL power value in 100% of 100 eyes tested when compared with five other IOL formulas.
Ladas Super Formula
Now, Devgan and Ladas are using AI and big data to improve upon their formula and inch IOL accuracy closer to perfection.
“What that ends up getting us is an unprecedented level of accuracy,” Devgan said.
By taking outcome data and seamlessly incorporating it into the original Ladas Super Formula, there are no out-of-bounds areas, improving what is already a “good solution,” according to Ladas, of Maryland Eye Consultants.
As new input parameters come into play, such as more precise measurements of posterior corneas, they can be “seamlessly incorporated” and evolve quickly in this artificial intelligence algorithm because it already has a backbone formula to work from, Ladas said.
“It does not have to go back and relearn everything; you can feed it new pieces of information. As time goes on and different instrument devices are used to feed information to the process, our algorithm can learn it very quickly. The analogy I like to use is that we all know a computer can beat a grandmaster in chess; this was based on feeding the computer the rules of the game and letting it play the same game over and over. If the rules change in the middle of the game or if a new chess piece is introduced — you get another rook, for example — this would be the equivalent of having a new IOL calculation input variable. The machine learning can quickly learn the influence of the new rules, weigh it appropriately in concert with the other variable involved, and you do not have to reinvent the game over and over again,” he said.
High level of accuracy
The new formula may be able to offer more accurate results than LASIK, Devgan said.
An independent surgeon in Florida, Vinay Gutti, MD, sent Devgan data from 140 eyes of patients on which he performed cataract surgery. Using the preoperative measurements and knowing which lens each patient received, Devgan used the formula and a specially created neural net to see how many eyes it could get to within ±0.5 D.
“What would have been the perfect lens? It’s hindsight. If we use just the Ladas Super Formula, we get about 85% of the patients on target. The Barrett formula is similar — we get about 82%. If we now use our AI, our neural net, with other people’s data, we can get 87% correct. If I use the neural net and use [Gutti’s] data, I can get his eyes to 94% correct. LASIK, remember, is 92% of patients getting to ±0.5 D target. You can get better than LASIK or equivalent to LASIK results with cataract patients using artificial intelligence,” he said.
While the 94% accuracy using the neural net and Gutti’s data is impressive, Ladas said the 87% accuracy rating using just the formula and a neural net created from other surgeons’ data is the most impressive outcome.
“We took a very good surgeon, an ‘A’ surgeon, and then took data from other people within our library and created a neural net. We created an adjustment that did not include his data. We made him even better. It’s taking the outcomes of people who are potentially not as good, and what this tells me is there is inherent potential room for improvement in all formulas and for all surgeons,” Ladas said.
Accurate disease diagnosis
One challenge in every field of medicine is accurate disease diagnosis. Diagnosis can be particularly subjective and qualitative, especially in ophthalmology when so much is dependent on looking at patterns and morphology, according to Michael F. Chiang, MD, of the Casey Eye Institute at Oregon Health and Science University.
Because of this, ophthalmology often has a high level of variability in diagnosis, even among experts in the field, he said.
“We definitely see that in retinopathy of prematurity, where it has been very well demonstrated that different people can look at the same retina and come up with different diagnoses. On the one level, methods from artificial intelligence can be applied to improve the accuracy and reproducibility of ophthalmic diagnoses, and ROP is one example of that,” Chiang said.
Systems have been developed that can diagnose ROP better than expert ophthalmologists, he said, which highlights how much diagnostic variability there can be in this field.
AI has the potential to provide tools for ophthalmologists to take better care of their patients and help ophthalmologists rediscover the “art of doctoring,” Chiang said.
“We’re in an era of electronic health records. Because of their design right now in 2018, they take longer to use. They have the risk of creating more separation between patients and doctors. We have good published data showing that this happens. I hope that artificial intelligence is a technology that will have the potential to help streamline the diagnostic process for doctors and hopefully help doctors spend more time in focusing on getting to know their individual patients and building that patient-provider relationship,” he said.
The art of doctoring
The human factor, the “art of doctoring,” has been slowly deteriorating over the last 10 to 15 years, Chiang noted, but AI systems may be a way for doctors to focus their efforts on “the uniquely human aspects of medicine.”
“How do we thoughtfully design what these systems should do and how do we integrate them into our day-to-day practice, to leverage them for what they can do to help us? They’ll get rid of the doctoring of medicine if we let them get rid of the doctoring of medicine. If we anticipate what they are going to do and how we can take our practice of medicine and make it better based on these systems, I think that is where we will make those advances,” he said.
It is incumbent upon the health care profession to be thoughtful about the best way to integrate these systems into the field and to find the right way to advance medicine and not be helpless to these technologies, Chiang said.
Automated solutions
Deep learning models have the potential to take ophthalmologists away from “trivial visual tasks that were impossible to fully automate” by now providing fully automated solutions, Lee said.
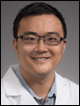
For example, the tracing of every intraretinal fluid cyst in a patient with severe diabetic macular edema is “extremely tedious” for a human grader to perform, Lee said.
“Yet, total macular fluid volume is a much more sensitive measure to follow in patients being treated with anti-VEGF injections. A fully automated solution has been difficult to create since the OCT shadow casted by overlying retinal vessels can cause hyporeflectivity that can confuse traditional algorithms. Since deep learning networks are designed after our own visual processing system, the models can not only see visual features in an image but have a higher-level understanding about these visual features,” Lee said.
The AI field is constantly moving and accelerating, with new systems, formulas and algorithms being developed, Ladas said.
Recently, Ladas and Devgan have made strides in a new AI algorithm for post-refractive eyes that has yielded exciting results.
“We took virgin eye data from people who underwent LASIK, 50 eyes. We created a neural net to find out the difference between what they would have needed before LASIK and after LASIK, and then we applied that adjustment to a completely random data set that underwent cataract surgery after LASIK, and we got 73% of eyes within 0.5 D. Nothing in a completely different data set has ever been over 63%. It’s unprecedented,” Ladas said.
Even with the most problematic eyes, the new algorithm is 10% greater than anything out there in the literature, he said.
Not a replacement for experience
Despite these advances, AI will never “take the place of your brains or your experience,” Devgan said. Systems can give unreasonable, out-of-bounds answers that professionals need to recognize and take steps to correct.
“To prevent that, one thing we do in our formula in our artificial intelligence is we use our original formula as a framework. We don’t let the AI come up with an answer that is too far away from what we would have predicted. If I predict the lens score to be 20 and the AI says 30, then I’m out. But if we predict 20 and the AI predicts 20.5, then maybe I’ll listen to it. It has to be close. It can’t be an out-of-bounds thing. It has to make sense,” Devgan said.
With so many advances in AI, it is a common misconception that these technologies will replace jobs for clinicians in ophthalmology, Lee said.
“This is simply not true. Deep learning has the potential instead to allow clinicians to become much more efficient and deliver better care. The current state-of-the-art deep learning models are very good at a specific task that it was trained to perform and cannot generalize. For example, a model trained to grade diabetic retinopathy will not know what to make of a fundus image with someone with a branch retinal vein occlusion. It will not report that this is a new image that it has never seen before but instead attempt to assign a retinopathy grade to the image,” he said.
Deep learning models have an impressive ability to learn, but they are limited by the breadth of the training data and the ground truth labels, Lee said. – by Robert Linnehan
- References:
- American Academy of Ophthalmology finds Google’s AI program is building better algorithms for detecting eye disease. https://www.prnewswire.com/news-releases/american-academy-of-ophthalmology-finds-googles-ai-program-is-building-better-algorithms-for-detecting-eye-disease-300613264.html. Published March 13, 2018. Accessed April 23, 2018.
- Devgan U. Achieving refractive accuracy in cataract surgery. https://www.healio.com/ophthalmology/cataract-surgery/news/print/ocular-surgery-news/%7B271c23b1-124c-4ae8-ac3f-0eda884c5609%7D/achieving-refractive-accuracy-in-cataract-surgery. Published Feb. 25, 2017. Accessed April 10, 2018.
- Devgan U. IOL calculations evolve with artificial intelligence. https://www.healio.com/ophthalmology/cataract-surgery/news/print/ocular-surgery-news/%7bc42e220a-ca4e-47b4-a1d7-1bb7e0dffdfb%7d/iol-calculations-evolve-with-artificial-intelligence. Published April 25, 2018. Accessed April 26, 2018.
- Google’s AI Program: Building better algorithms for detecting eye disease. https://www.aao.org/newsroom/news-releases/detail/google-ai-building-better-eye-disease-detection. Published March 2, 2018. Accessed April 9, 2018.
- Gulshan V, et al. JAMA. 2016;doi:10.1001/jama.2016.17216.
- Krause J, et al. Ophthalmology. 2018;doi:10.1016/j.ophtha.2018.01.034.
- Ladas JG, et al. JAMA Ophthalmol. 2015;doi:10.1001/jamaophthalmol.2015.3832.
- For more information:
- Lama A. Al-Aswad, MD, MPH, can be reached at The Edward S. Harkness Eye Institute, Columbia University Medical Center, 635 West 165th St., Suite 533, New York, NY 10032; email: laa2003@cumc.columbia.edu.
- Michael F. Chiang, MD, can be reached at Oregon Health and Science University, 3375 SW Terwilliger Blvd., Portland, OR 97239; email: chiangm@ohsu.edu.
- Uday Devgan, MD, can be reached at Devgan Eye Surgery, 11600 Wilshire Blvd. #200, Los Angeles, CA 90025; email: devgan@gmail.com.
- John G. Ladas, MD, PhD, can be reached at Maryland Eye Consultants, 2101 Medical Park Drive, #101, Silver Spring, MD 20902; email: jladas@marylandeye.com.
- Aaron Y. Lee, MD, can be reached at UW Medicine, 1959 NE Pacific St., Seattle, WA 98195; email: leeay@uw.edu.
- Lily Peng, MD, PhD, can be reached at Google, 1600 Amphitheatre Parkway, Mountain View, CA 94043; email: lhpeng@google.com.
Disclosures: Al-Aswad and Lee report no relevant financial disclosures. Chiang reports he is an unpaid member of the scientific advisory board for Clarity Medical Systems, a consultant for Novartis and an initial member of Inteleretina. Devgan reports he is a principal in Advanced Euclidean Solutions, which owns the Ladas Super Formula, of which he generates no revenue. Ladas reports he is a principal in Advanced Euclidean Solutions. Peng reports she is an employee of Google, submitted a patent to Google and has stock ownership in Google.
Click here to read the , "Do you envision ophthalmologists becoming too dependent on artificial intelligence?"